Uncertainty-Aware Few-Shot Class-Incremental Learning
ICASSP 2023 - 2023 IEEE International Conference on Acoustics, Speech and Signal Processing (ICASSP)(2023)
摘要
In a real-world setting, machine needs to continuously recognize new categories without forgetting. However, the number of new categories may be small. For some difficult categories, even humans cannot recognize only based on few-shot examples. To address the above issues, an innovative uncertainty-aware few-shot class incremental learning method (UACL) is proposed, which allows the model to continuously recognize new classes with few-shot examples and identify the classes it cannot recognize currently. Besides, in order to imitate the cognitive way of human beings and improve the continuous representation ability, we propose a pseudo-incremental task construction mechanism based on uncertainty estimation, where the machine learn to recognize from simple to difficult. Further, a large-scale pre-training model is used as an expert system to guide the model to recognize difficult classes. We evaluate our method on three popular benchmark datasets, showing that UACL is state-of-the-art.
更多查看译文
关键词
Few-Shot Learning,Class-Incremental Learning,Uncertainty-Aware,Curriculum Learning
AI 理解论文
溯源树
样例
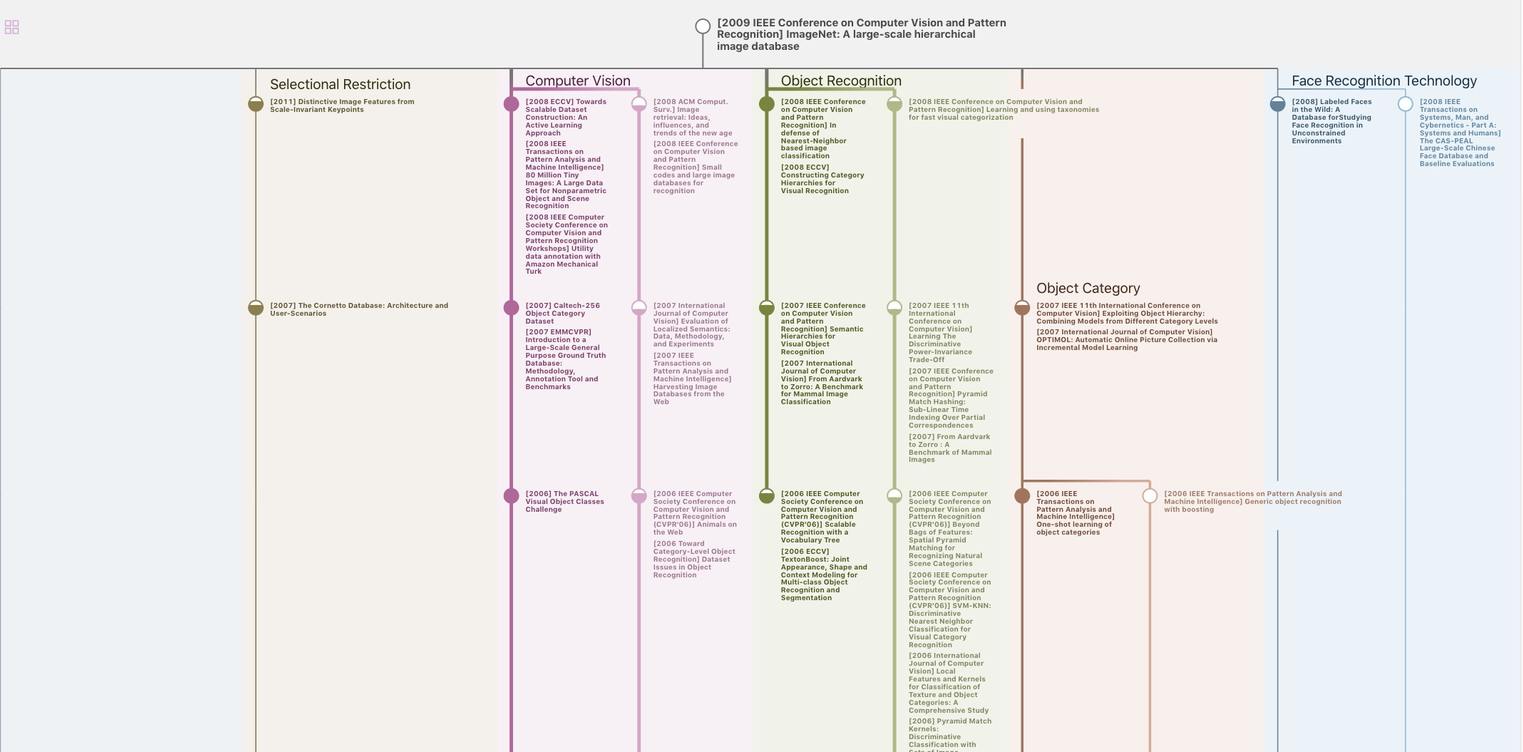
生成溯源树,研究论文发展脉络
Chat Paper
正在生成论文摘要