Test-Time Training-Free Domain Adaptation
ICASSP 2023 - 2023 IEEE International Conference on Acoustics, Speech and Signal Processing (ICASSP)(2023)
摘要
Deploying deep learning models to new environments is very challenging. Domain adaptation (DA) is a promising paradigm to solve the problem by collecting and adapting to unlabeled data in new environments. Though research efforts have led to steady performance improvement over the past decade, DA algorithms are still hard to deploy, as training on unlabeled new data makes tuning difficult and not feasible for inference-only devices. To make DA practical, in this paper we study a new problem named Test-time Training-Free Domain Adaptation (TTDA), where trained models must adapt to a single input (mimicking the test-time scenario) without training. By exploiting spatial activation that was previously overlooked and simply averaged out, we propose a simple method based on Feature Statistics Transformation (FST) on-the-fly for each test example. The proposed algorithm is tested in the TTDA setting on two standard DA benchmarks. Surprisingly, it surpasses or performs on par with state-of-the-art DA methods, even though they require additional training. We envision that this training-free paradigm has the potential to bring DA to embedded devices and would be of interest to audience of community.
更多查看译文
关键词
Transfer Learning,Domain Adaption,Training-Free Model,Feature Statistics Transformation
AI 理解论文
溯源树
样例
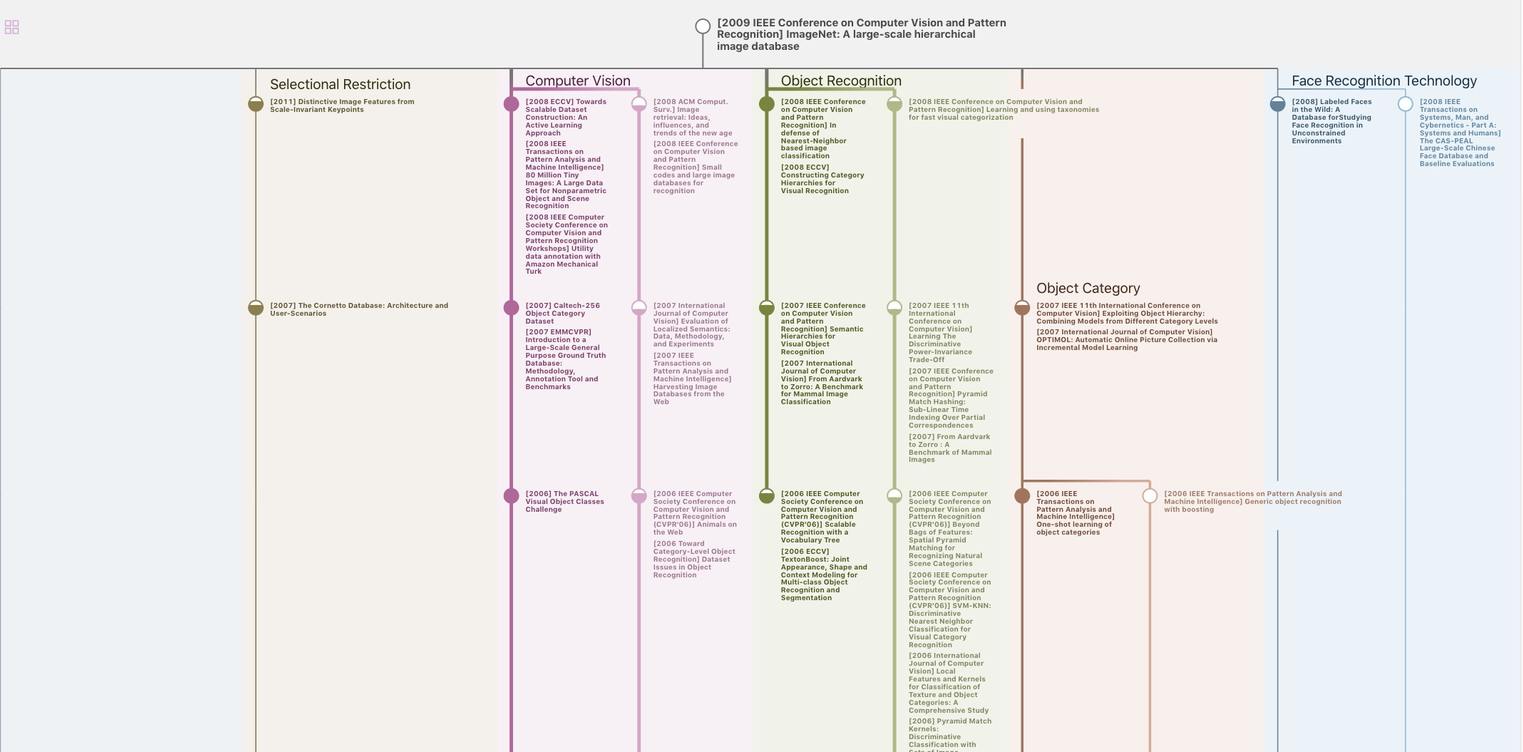
生成溯源树,研究论文发展脉络
Chat Paper
正在生成论文摘要