Compressed Distributed Regression over Adaptive Networks
ICASSP 2023 - 2023 IEEE International Conference on Acoustics, Speech and Signal Processing (ICASSP)(2023)
摘要
We examine the learning performance achievable by a network of agents that solve a distributed regression problem using the recently proposed ACTC (Adapt-Compress-Then-Combine) diffusion strategy. The agents operate under communication constraints: they are allowed to communicate only with their immediate neighbors, and the exchanged signals are encoded by using randomized differential compression operators. We show that the mean-square estimation error of each agent comprises the error that the agents would achieve without communication constraints plus a compression loss. Our results reveal the fundamental quantitative relationship existing between the compression loss and the peculiar attributes of the distributed regression problem. We show how these quantitative relationships can be used to optimize the allocation of communication resources across the agents and improve their learning performance as compared to a uniform allocation.
更多查看译文
关键词
Distributed optimization,diffusion strategy,randomized quantizers,differential quantization
AI 理解论文
溯源树
样例
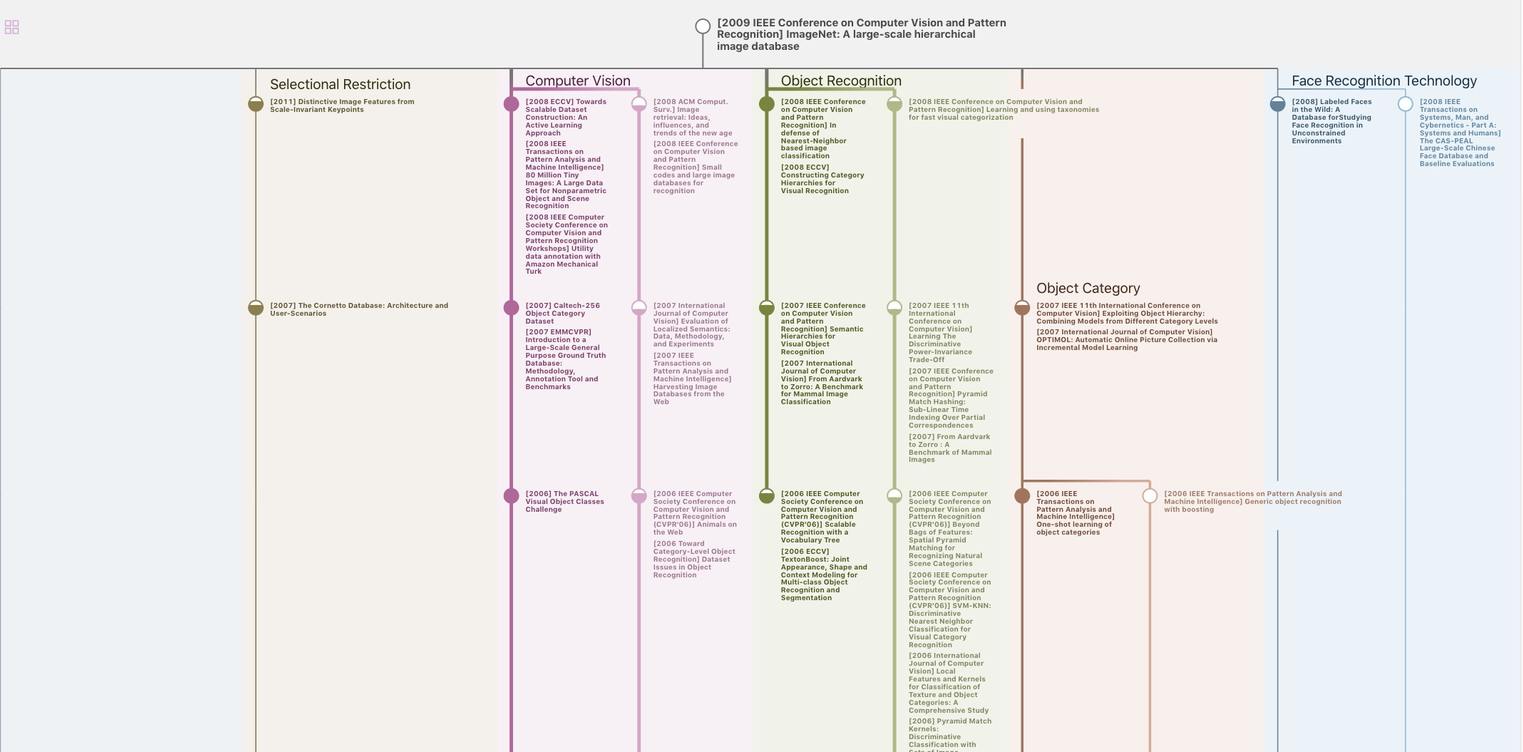
生成溯源树,研究论文发展脉络
Chat Paper
正在生成论文摘要