Effective Variational-Autoencoder-Based Generative Models for Highly Imbalanced Fault Detection Data in Semiconductor Manufacturing
IEEE Transactions on Semiconductor Manufacturing(2023)
摘要
In current semiconductor manufacturing, limited raw trace data pertaining to defective wafers make fault detection (FD) assignments extremely difficult due to the data imbalance in wafer classification. To mitigate, this paper proposes using a variational autoencoder (VAE) as a data augmentation strategy for resolving data imbalance of temporal raw trace data. A VAE with few defective samples is first trained. By means of extracting the latent variables that characterize the distribution of the defective samples, we make use of the statistical randomness of the latent variables to generate synthesized defective samples via the decoder scheme in the trained VAE. Two data representations and VAE modeling strategies, concatenation of multiple and individual raw trace data as the input of the VAE during the training stage, are investigated. A real-data plasma enhanced chemical vapor deposition (PECVD) process having only few defective samples is used to illustrate the performance enhancement to wafer classification arising from the proposed data augmentation framework. Based on the computational comparisons between noted classification models, the proposed generative VAE model via the individual strategy enables the adaptive boosting (AdaBoost) classifier to achieve perfect performances in every metrics if the 80% and 100% over-sampling ratios are adopted.
更多查看译文
关键词
Variational autoencoder (VAE),data augmentation,wafer classification,semiconductor manufacturing,fault detection
AI 理解论文
溯源树
样例
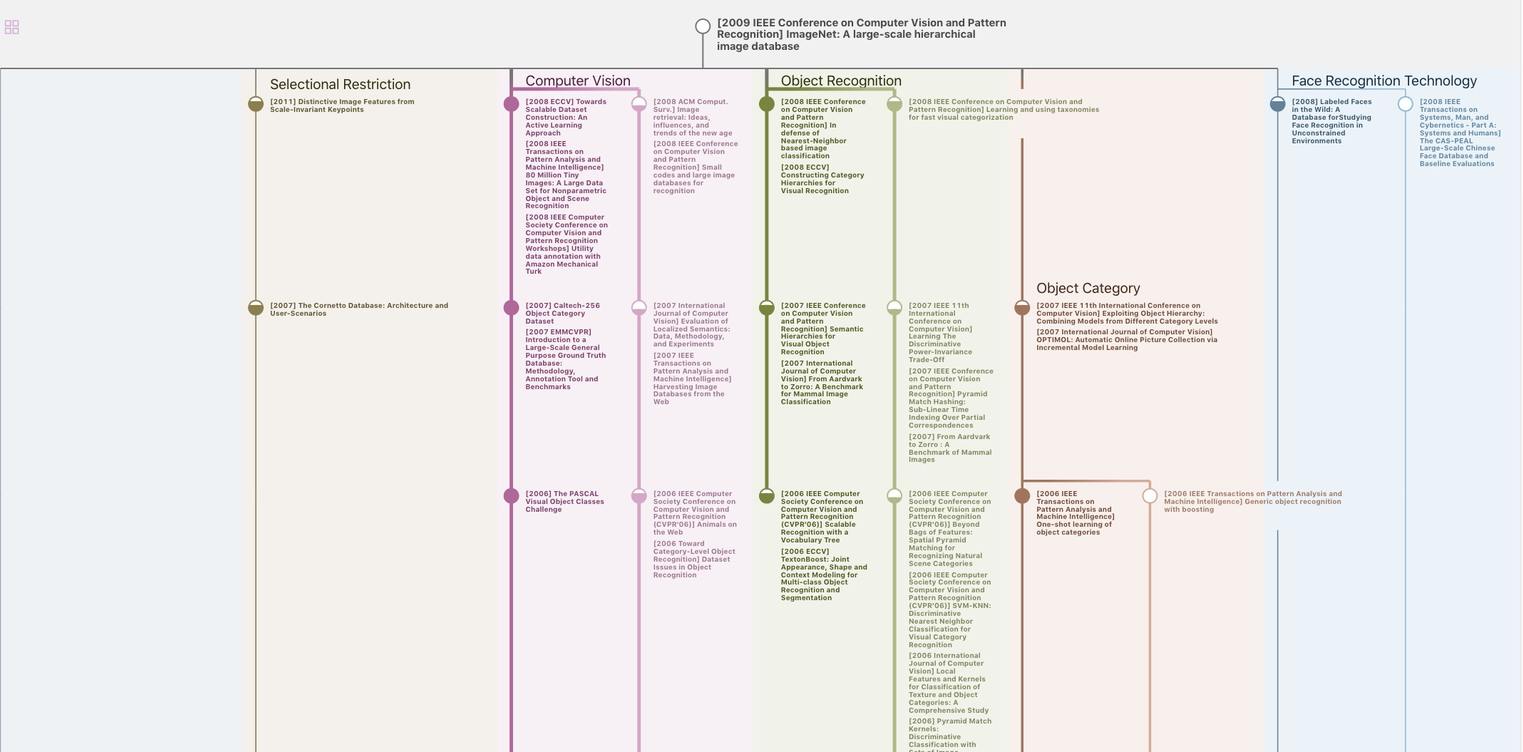
生成溯源树,研究论文发展脉络
Chat Paper
正在生成论文摘要