Improving patient flow through hospitals with machine learning based discharge prediction
medRxiv (Cold Spring Harbor Laboratory)(2023)
摘要
Background: Advanced analytics, underpinned by large-scale electronic health record (EHR) data, have the potential to transform the efficiency of healthcare delivery. Methods: We used data from 4 hospitals in Oxfordshire, UK (01-February-2017 to 31-January-2020), to develop machine learning models for predicting hospital discharge in the next 24 hours, conditional on the duration of hospitalisation to date. We fitted separate XGBoost models for patients in hospital after planned and emergency admissions and for patients with different lengths of stay since admission. Models were trained and tested using data from 126,054 emergency and 45,609 planned admissions. Findings: In held-out test data (01-February-2019 to 31-January-2020), individual patient discharge was predicted with positive and negative predictive values of 87.5% and 94.1% respectively in emergency patients (sensitivity 82.3%, specificity 98.5%, AUPRC 87.6%, AUC 87.5%) and 95.6% and 97.8% after a planned admission (sensitivity 83.5%, specificity 98.1%, AUPRC 90.5%, AUC 90.1%). Combining individual discharge probabilities allowed accurate estimates of the total number of discharges from hospital, mean average error 5.8% for emergency and 3.1% for planned patients. Previous hospital exposure, procedures, antibiotic use, and comorbidities were the most predictive features, but the most important features differed between planned and emergency patients, and over the course of admission. Performance was best in short-stay and planned admission patients, but generally robust over time, across subgroups, and different model training strategies. Interpretation: Our approach could help improve flow of patients through hospitals, resulting in faster care delivery and patient recovery, and better use of healthcare resources. Funding: National Institute for Health Research.
### Competing Interest Statement
DWE declares lecture fees from Gilead, outside the submitted work. DAC reports personal fees from Oxford University Innovation, personal fees from BioBeats, personal fees from Sensyne Health, outside the submitted work. No other author has a conflict of interest to declare.
### Funding Statement
This study was funded by the National Institute for Health Research (NIHR) Health Protection Research Unit in Healthcare Associated Infections and Antimicrobial Resistance at Oxford University in partnership with the UK Health Security Agency (UKHSA) and the NIHR Biomedical Research Centre, Oxford. The views expressed in this publication are those of the authors and not necessarily those of the NHS, the National Institute for Health Research, the Department of Health and Social Care or the UKHSA. DAC was supported in part by the National Institute for Health Research (NIHR) Oxford Biomedical Research Centre (BRC); the InnoHK Hong Kong Centre for Cerebro-cardiovascular Health Engineering; the Pandemic Sciences Institute at the University of Oxford; an NIHR Research Professorship and a Royal Academy of Engineering Research Chair. DWE is a Robertson Foundation Fellow. The funders had no role in in study design; in the collection, analysis, and interpretation of data; in the writing of the report; or in the decision to submit the paper for publication.
### Author Declarations
I confirm all relevant ethical guidelines have been followed, and any necessary IRB and/or ethics committee approvals have been obtained.
Yes
The details of the IRB/oversight body that provided approval or exemption for the research described are given below:
National Research Ethics Service South Central - Oxford C Research Ethics Committee (19/SC/0403), the Health Research Authority and the national Confidentiality Advisory Group (19/CAG/0144) gave ethical approval for this work.
I confirm that all necessary patient/participant consent has been obtained and the appropriate institutional forms have been archived, and that any patient/participant/sample identifiers included were not known to anyone (e.g., hospital staff, patients or participants themselves) outside the research group so cannot be used to identify individuals.
Yes
I understand that all clinical trials and any other prospective interventional studies must be registered with an ICMJE-approved registry, such as ClinicalTrials.gov. I confirm that any such study reported in the manuscript has been registered and the trial registration ID is provided (note: if posting a prospective study registered retrospectively, please provide a statement in the trial ID field explaining why the study was not registered in advance).
Yes
I have followed all appropriate research reporting guidelines, such as any relevant EQUATOR Network research reporting checklist(s) and other pertinent material, if applicable.
Yes
The datasets analysed during the current study are not publicly available as they contain personal data but are available from the Infections in Oxfordshire Research Database (https://oxfordbrc.nihr.ac.uk/ research-themes-overview/antimicrobial- resistance-and-modernising-microbiology/ infections-in-oxfordshire-research-database-iord/), subject to an application and research proposal meeting the ethical and governance requirements of the Database. For further details on how to apply for access to the data and for a research proposal template please email iord@ndm.ox.ac.uk.
更多查看译文
关键词
discharge prediction,patient flow,hospitals,machine learning
AI 理解论文
溯源树
样例
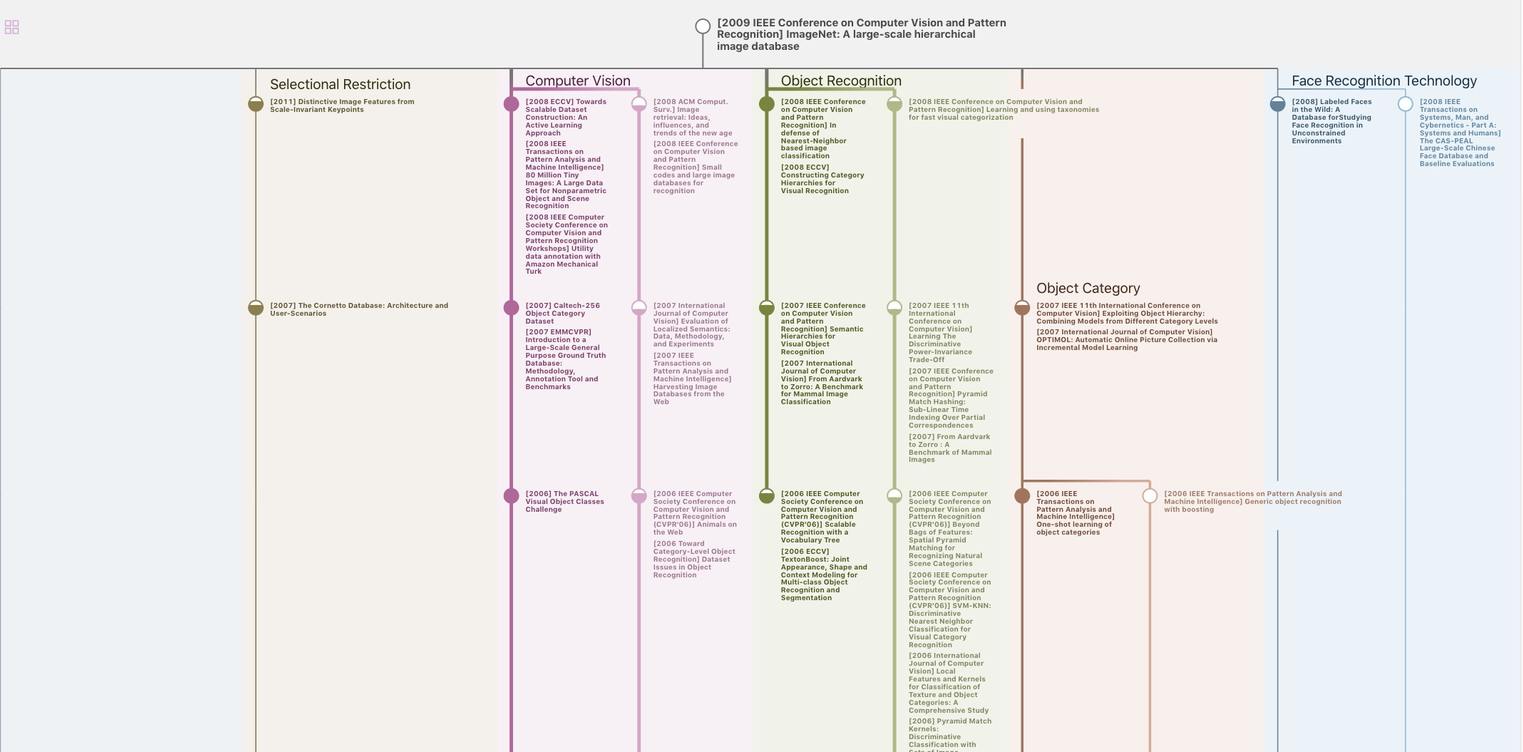
生成溯源树,研究论文发展脉络
Chat Paper
正在生成论文摘要