Embedding Global Contrastive and Local Location in Self-Supervised Learning
IEEE Transactions on Circuits and Systems for Video Technology(2023)
摘要
Self-supervised representation learning (SSL) typically suffers from inadequate data utilization and feature-specificity due to the suboptimal sampling strategy and the monotonous optimization method. Existing contrastive-based methods alleviate these issues through exceedingly long training time and large batch size, resulting in non-negligible computational consumption and memory usage. In this paper, we present an efficient self-supervised framework, called GLNet. The key insights of this work are the novel sampling and ensemble learning strategies embedded in the self-supervised framework. We first propose a location-based sampling strategy to integrate the complementary advantages of semantic and spatial characteristics. Whereafter, a Siamese network with momentum update is introduced to generate representative vectors, which are used to optimize the feature extractor. Finally, we particularly embed global contrastive and local location tasks in the framework, which aims to leverage the complementarity between the high-level semantic features and low-level texture features. Such complementarity is significant for mitigating the feature-specificity and improving the generalizability, thus effectively improving the performance of downstream tasks. Extensive experiments on representative benchmark datasets demonstrate that GLNet performs favorably against the state-of-the-art SSL methods. Specifically, GLNet improves MoCo-v3 by 2.4% accuracy on ImageNet dataset, while improves 2% accuracy and consumes only 75% training time on the ImageNet-100 dataset. In addition, GLNet is appealing in its compatibility with popular SSL frameworks. Code is available at GLNet.
更多查看译文
关键词
Self-supervised representation learning,contrastive learning,location-based sampling,ensemble learning
AI 理解论文
溯源树
样例
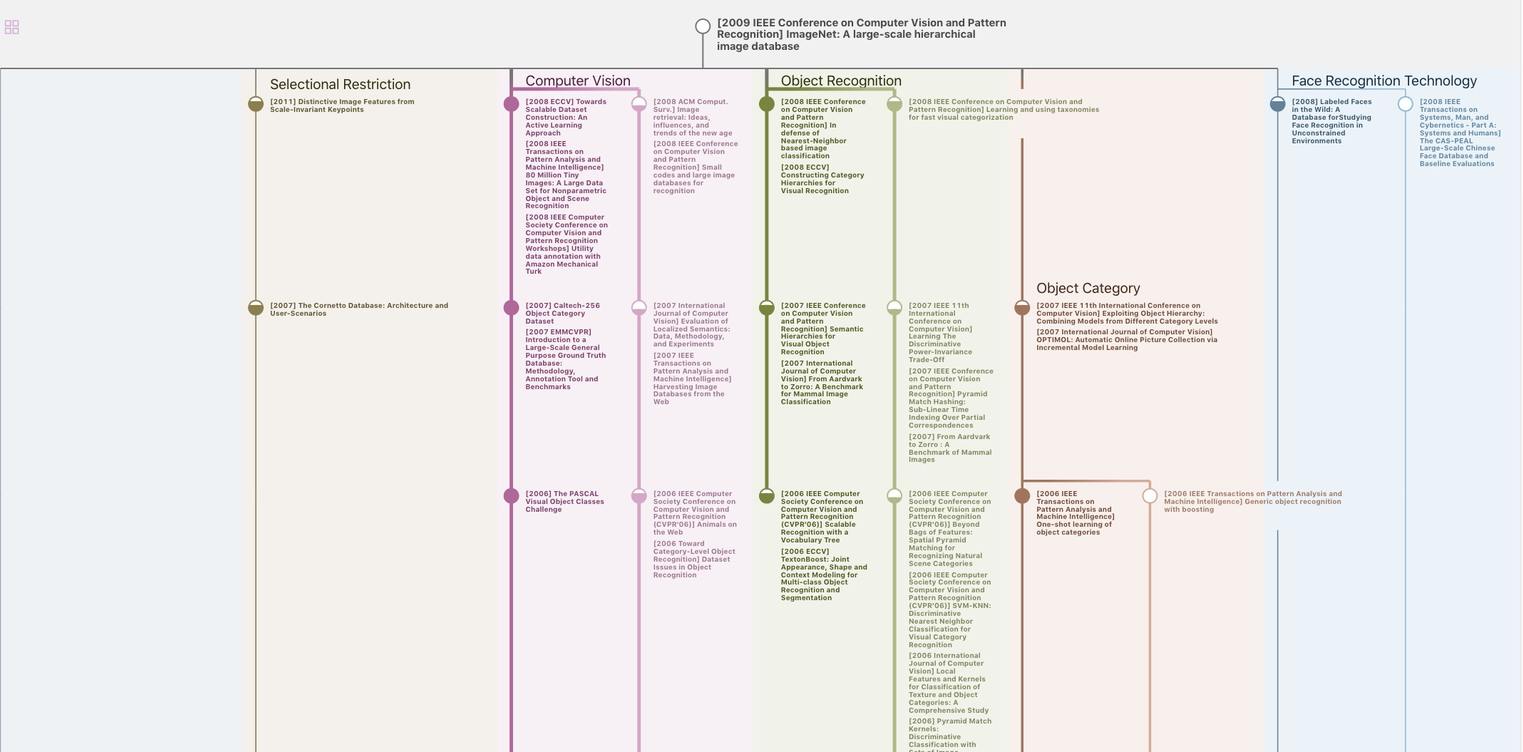
生成溯源树,研究论文发展脉络
Chat Paper
正在生成论文摘要