Vision Transformer With Enhanced Self-Attention for Few-Shot Ship Target Recognition in Complex Environments
IEEE Transactions on Instrumentation and Measurement(2023)
Abstract
Ship target recognition is essential for maritime transportation, commercial trade, maritime security, and monitoring illegal activity. The majority of previous ship target recognition models are based on fully supervised learning, which necessitates vast amounts of labeled training data. However, optical lenses capturing ship images in natural scenarios have numerous complex environments, making feature extraction difficult due to hardware, weather, light, waves, and other factors. Moreover, image annotation is also both expensive and time-consuming. How to effectively learn from unbalanced, limited, and sparsely annotated sample data is a significant challenge. In this article, we propose a new vision transformer with enhanced self-attention (SAVT) that can locate ship targets in complex environments with just a few shots. SAVT consists of a novel enhanced self-attention (ESA) module and a novel measurement self-adjusting computation (MAC) module to differentiate unique ship classes in complex environments with few labeled samples. The ESA module focuses on the features of the target region at each layer of the backbone network to ensure that the contour detailed features are extracted effectively from ship images with complex environments. The model is then enhanced in terms of speed and stability using the MAC module. The CIB-ships and public MAR-ships datasets are used to evaluate the proposed method. In this article, the results demonstrate that SAVT achieves good performance on few-shot labeled data under both one-shot and five-shot settings, while also providing valuable guidance for maritime ship target recognition with limited labeled samples.
MoreTranslated text
Key words
Enhanced self-attention (ESA),few-shot,measurement self-adjusting computation (MAC),ship image in complex environments,vision transformer
AI Read Science
Must-Reading Tree
Example
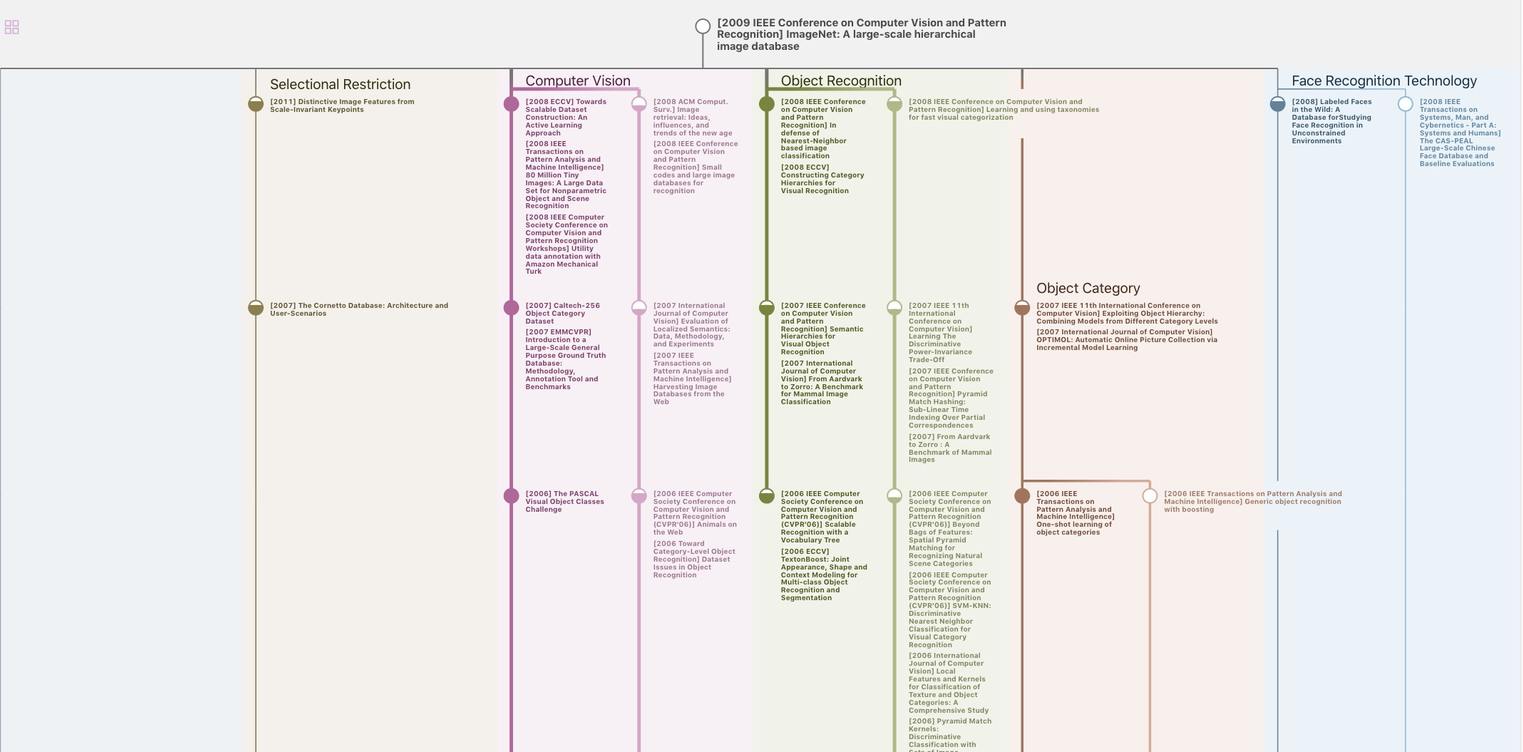
Generate MRT to find the research sequence of this paper
Chat Paper
Summary is being generated by the instructions you defined