Permutation-Equivariant and Proximity-Aware Graph Neural Networks With Stochastic Message Passing
IEEE Transactions on Knowledge and Data Engineering(2023)
摘要
Graph neural networks (GNNs) are emerging machine learning models on graphs. Permutation-equivariance and proximity-awareness are two important properties highly desirable for GNNs. Both properties are needed to tackle some challenging graph problems, such as finding communities and leaders. In this paper, we first analytically show that the existing GNNs, mostly based on the message-passing mechanism, cannot simultaneously preserve the two properties. Then, we propose Stochastic Message Passing (SMP) model, a general and simple GNN to maintain both proximity-awareness and permutation-equivariance. In order to preserve node proximities, we augment the existing GNNs with stochastic node representations. We theoretically prove that the mechanism can enable GNNs to preserve node proximities, and at the same time, maintain permutation-equivariance with certain parametrization. We report extensive experimental results on ten datasets and demonstrate the effectiveness and efficiency of SMP for various typical graph mining tasks, including graph reconstruction, node classification, and link prediction.
更多查看译文
关键词
Graph neural network,message passing,node proximity,permutation equivariance
AI 理解论文
溯源树
样例
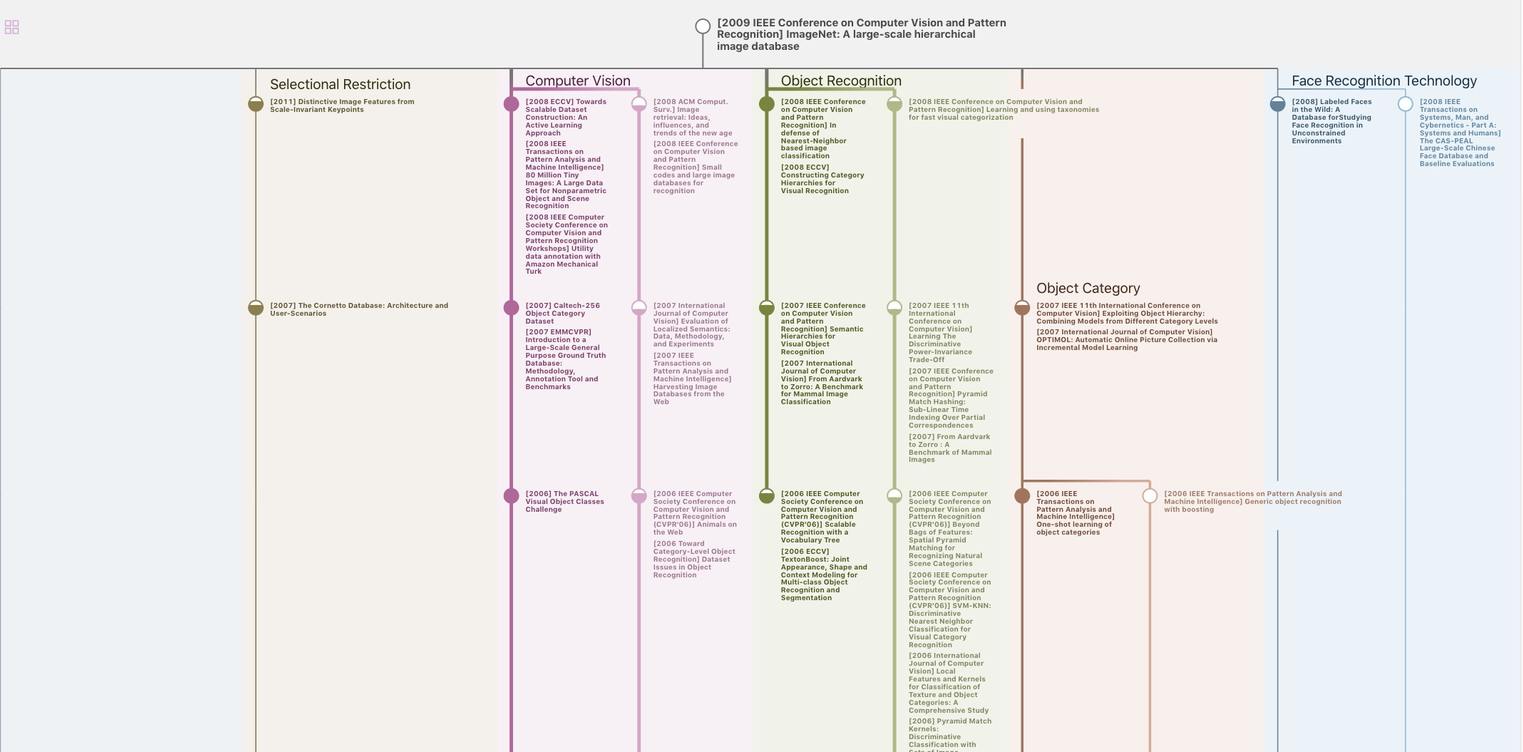
生成溯源树,研究论文发展脉络
Chat Paper
正在生成论文摘要