Robust State Estimation via Maximum Correntropy EKF on Matrix Lie Groups With Application to Low-Cost INS/GPS-Integrated Navigation System
IEEE Sensors Journal(2023)
摘要
This article focuses on solving state estimation problems of nonlinear systems whose states evolve on matrix Lie groups and measurements live in the Euclidean space under non-Gaussian measurement noise. Building upon the maximum correntropy criterion (MCC) and the invariant Kalman filtering theory, a generalized robust extended Kalman filtering (EKF) algorithm on matrix Lie groups is derived from maximizing a novel optimization cost function. This cost function is introduced by considering the correntropy and the system geometry structure. Then, two alternative versions of the proposed filtering algorithm are provided according to the distinct processing approaches of the nonlinear measurement function in the cost function. A Gaussian–Newton method on Lie groups and a fixed-point iterative method are applied to update the posterior estimation. In addition, sufficient conditions are given to ensure the convergence of fixed-point iterative steps. Extensive Monte-Carlo simulations on an inertial navigation system/global position system (INS/GPS) integrated navigation system and experiments on open-source datasets validate the theoretical analysis and illustrate the effectiveness and robustness of the proposed filter.
更多查看译文
关键词
Extended Kalman filtering (EKF),Gaussian--Newton,inertial navigation system/global position system (INS/GPS)-integrated navigation system,matrix Lie groups,maximum correntropy criterion (MCC)
AI 理解论文
溯源树
样例
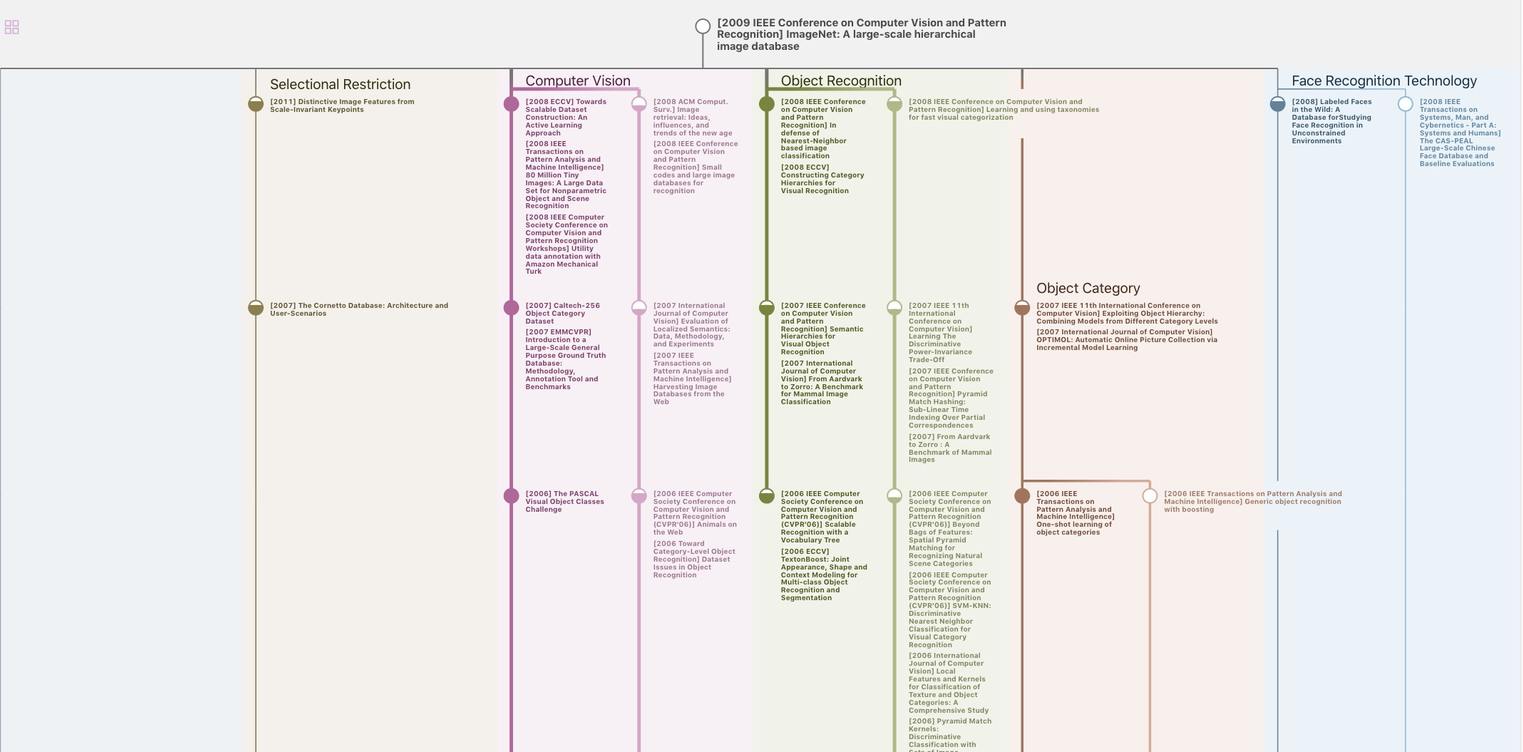
生成溯源树,研究论文发展脉络
Chat Paper
正在生成论文摘要