Improved Leakage Detection Generalization Ability for Multiscenes Deployment in Industry
IEEE Sensors Journal(2023)
Abstract
In the unattended industrial environment, the piping leakage detection algorithm based on the combination of image detection and machine learning has the advantages of high sensitivity and reliability. However, there are some problems in the practical application, such as a large number of industrial leakage scenes to be detected, a big spatial feature gap between the deployed scenes and the undeployed scenes, and the unpredictable actual leakage situation. These cause a significant decrease in the accuracy and reliability of vision-based piping leakage detection models during large-scale multiscenes industrial deployment. To solve the above problems, we propose a piping leakage detection method with domain generalization (DG) capability. First, we use the adaptive color recovery multiscale Retinex (AMSRCR) to enhance the spatial features and improve the image quality of leakage liquid, which is colorless and transparent. Based on the idea of DG, we design the structure of dynamic convolution module (DCM)-DenseNet121, which can improve the generalization ability of the model by learning the domain-invariant features of the leakage liquid and solve the problem of decreasing accuracy in large-scale multiscenes deployment. The experimental results show that the leakage detection balanced accuracy (BA) achieves 99.7% when inferring on three target scenes. Furthermore, our model achieves efficient detection, maintains a relatively lightweight when performing in extra-domain target scenes, and satisfies the industrial field bandwidth limitations.
MoreTranslated text
Key words
Domain generalization (DG),dynamic convolution module (DCM),image detail enhancement,piping leakage detection deployment
AI Read Science
Must-Reading Tree
Example
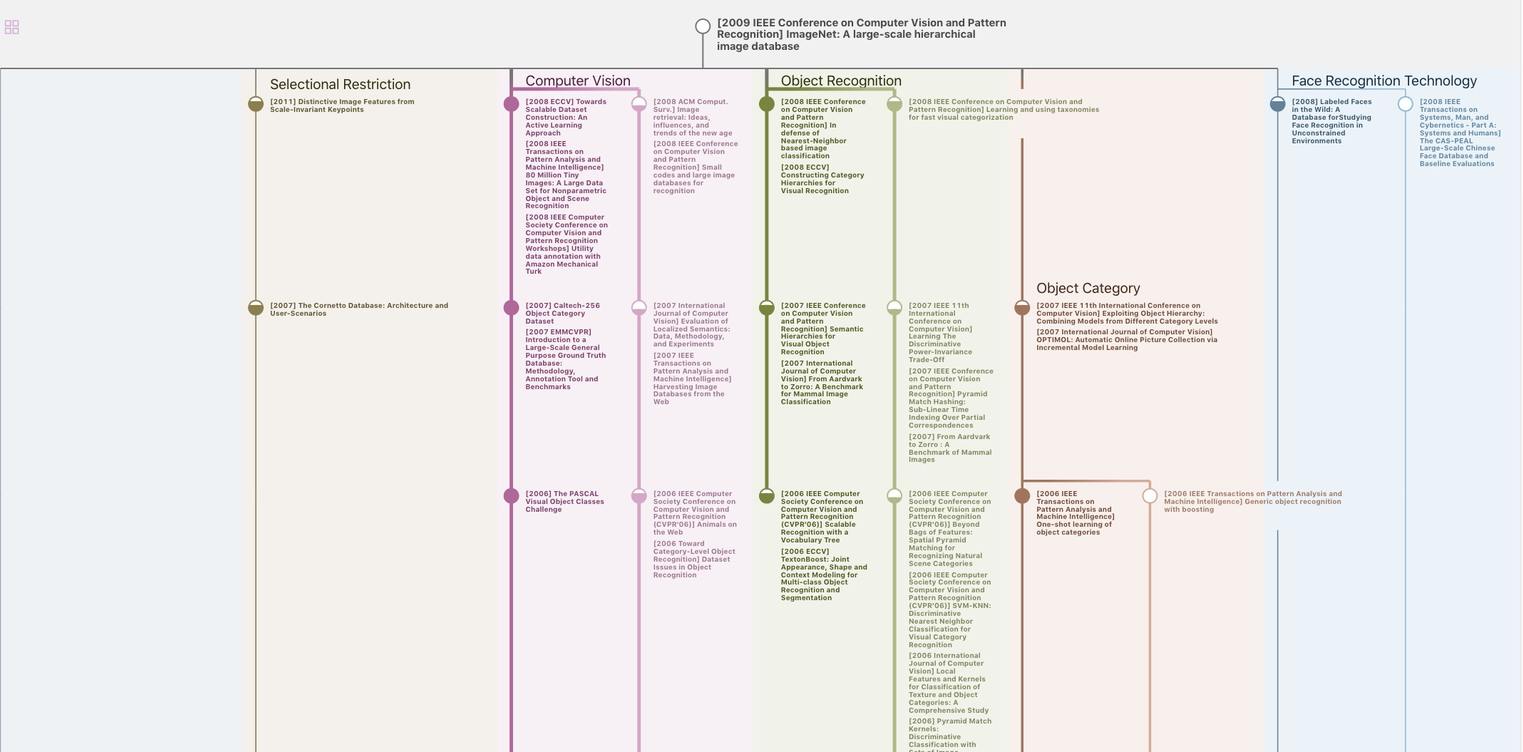
Generate MRT to find the research sequence of this paper
Chat Paper
Summary is being generated by the instructions you defined