Neural Network-Guided Synthesis of Recursive List Functions.
TACAS (1)(2023)
摘要
Kobayashi et al. have recently proposed NeuGuS, a framework of neural-network-guided synthesis of logical formulas or simple program fragments, where a neural network is first trained based on data, and then a logical formula over integers is constructed by using the weights and biases of the trained network as hints. The previous method was, however, restricted the class of formulas of quantifier-free linear integer arithmetic. In this paper, we propose a NeuGuS method for the synthesis of recursive predicates over lists definable by using the left fold function. To this end, we design and train a special-purpose recurrent neural network (RNN), and use the weights of the trained RNN to synthesize a recursive predicate. We have implemented the proposed method and conducted preliminary experiments to confirm the effectiveness of the method.
更多查看译文
关键词
synthesis,functions,network-guided
AI 理解论文
溯源树
样例
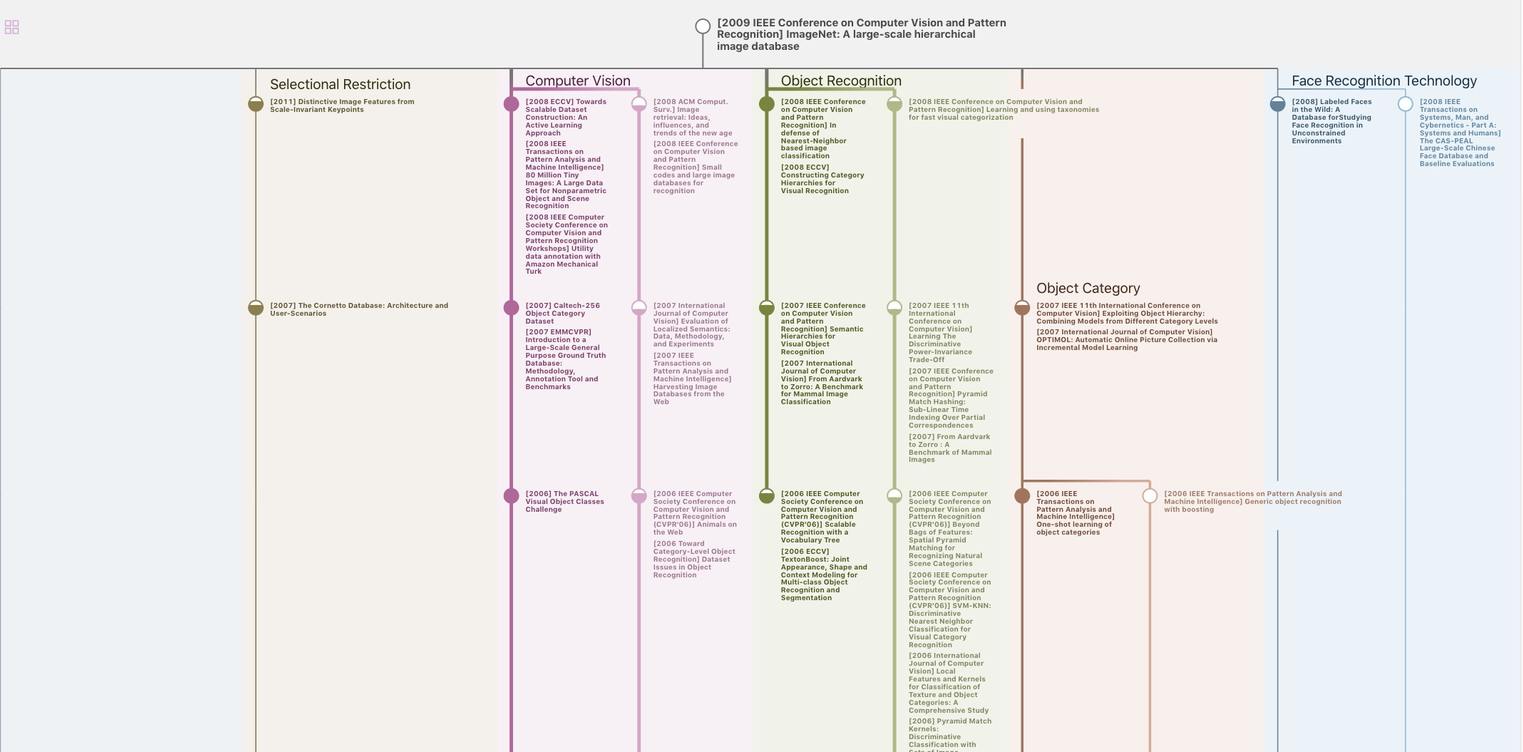
生成溯源树,研究论文发展脉络
Chat Paper
正在生成论文摘要