Intracardiac Electrical Imaging Using the 12-Lead ECG: A Machine Learning Approach Using Synthetic Data.
CinC(2022)
摘要
Current state-of-the-art techniques for non-invasive imaging of cardiac electrical phenomena require voltage recordings from dozens of different torso locations and anatomical models built from expensive medical diagnostic imaging procedures. This study aimed to assess if recent machine learning advances could alternatively reconstruct electroanatomical maps at clinically relevant resolutions using only the standard 12-lead electrocardiogram (ECG) as input. To that end, a computational study was conducted to generate a dataset of over 16000 detailed cardiac simulations, which was then used to train neural network (NN) architectures designed to exploit both spatial and temporal correlations in the ECG signal. Analysis over a validation set showed average errors in activation map reconstruction below 1.7 msec over 75 intracardiac locations. Furthermore, phenotypical patterns of activation and the morphology of the activation potential were correctly reconstructed. The approach offers opportunities to stratify patients non-invasively, both retrospectively and prospectively, using metrics otherwise only available through invasive clinical procedures.
更多查看译文
关键词
activation map reconstruction,anatomical models,average errors,cardiac electrical phenomena,cardiac simulations,clinically relevant resolutions,ECG signal,electroanatomical maps,electrocardiogram,intracardiac electrical imaging,intracardiac locations,invasive clinical procedures,machine learning,medical diagnostic imaging procedures,neural network architectures,noninvasive imaging,spatial correlations,temporal correlations,voltage recordings
AI 理解论文
溯源树
样例
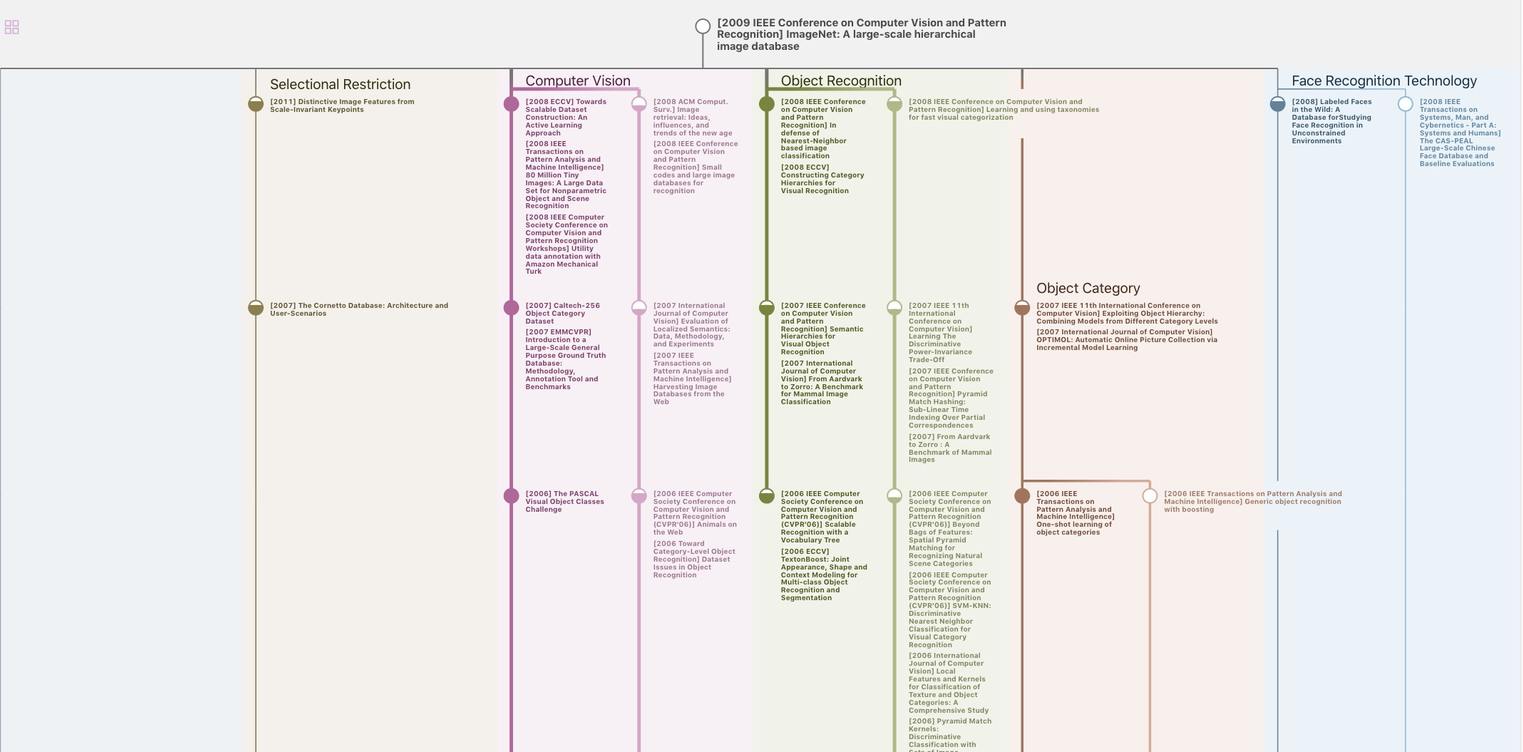
生成溯源树,研究论文发展脉络
Chat Paper
正在生成论文摘要