Model-based and Unsupervised Machine-learning Approaches for the Characterization of Responder Profiles for Cardiac Resynchronization Therapy.
CinC(2022)
摘要
The objective of this study is to improve the inter-pretability of a previous unsupervised clustering analysis of the CRT response through a physiological model-based approach. The developed clustering approach was applied on 250 CRT candidates based on clinical, original and classical echocardiographic features. Patient-specific computational models were proposed for patients associated of each cluster barycenter in order to provide an ex-plainable analysis in relation with physiological mecha-nisms. Five phenogroups were identified from the clustering approach with response rates ranging from 50% to 92.7%. Concerning the model-based approach, a match was observed between the 16 experimental and simulated myocardial strain curves pattern with a mean RMSE of 3.97%(
$\pm$
1.74) on the five patients. Moreover, the identified model parameters provide us information about the mecano-electrical coupling and tissue properties. The gain of information provides by the parameters model identification, added to the clinical and classical echocar-diographic features is promising for an understanding of LV mechanical dyssynchrony and the identification of patients suitable for CRT.
更多查看译文
关键词
canoelectrical coupling,cardiac resynchronization therapy,classical echocar-diographic features,classical echocardiographic features,clinical echocar-diographic features,cluster barycenter,clustering approach,CRT response,experimental strain curves pattern,model-based approach,myocardial strain curve pattern,patient-specificcomputational models,physiological mechanisms,physiological model-based approach,response rates,tissue properties,unsupervised clustering,unsupervised machine-learning approaches
AI 理解论文
溯源树
样例
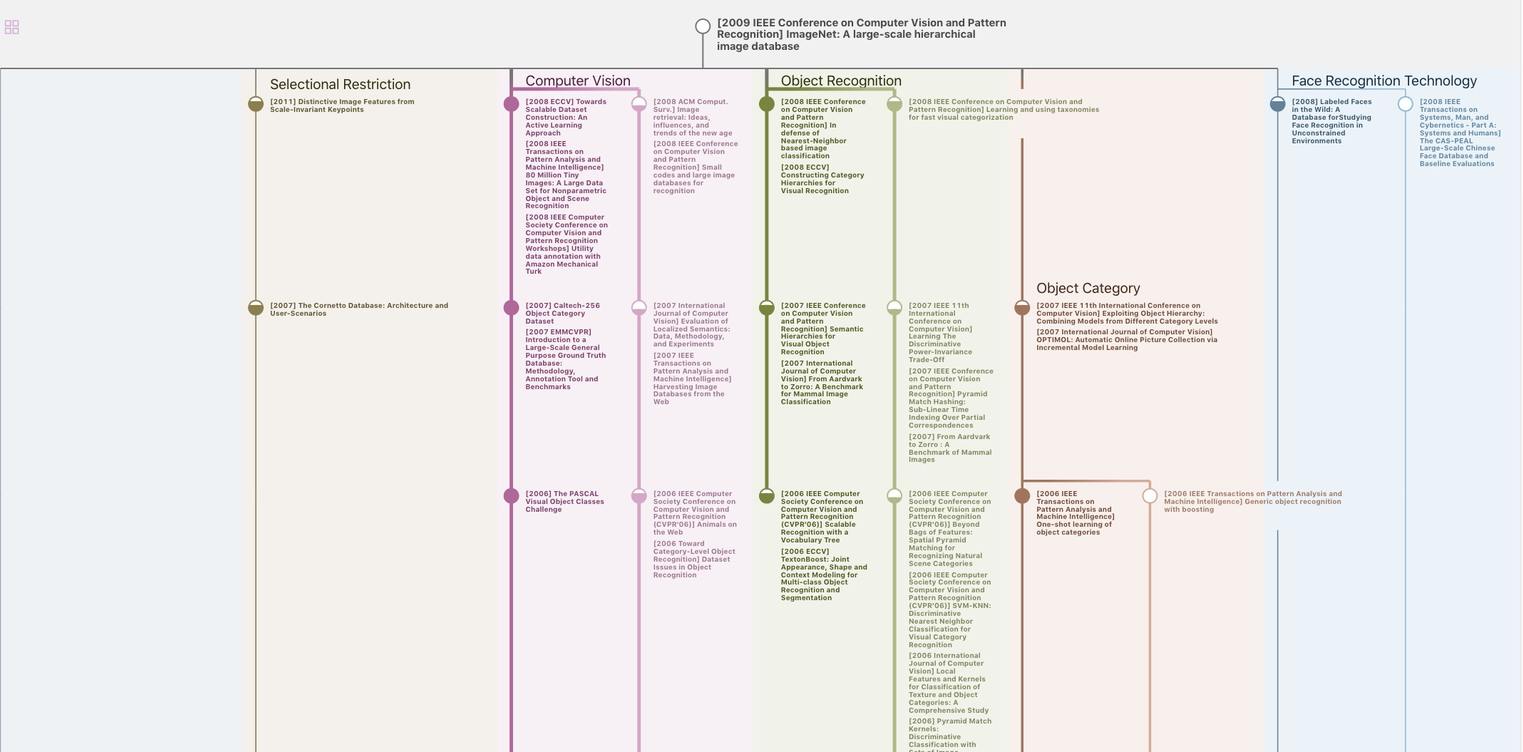
生成溯源树,研究论文发展脉络
Chat Paper
正在生成论文摘要