Joint Receiver Design with Natural Redundancy.
ICCT(2022)
Abstract
A high-speed information transmission system demands a more powerful communication receiver. In this paper, we integrate deep learning algorithms into constructing waveform level receiver, which is capable of harnessing natural redundancy (NR) in raw message sources. Owing to end-to-end learning paradigm of neural network (NN), the proposed NN receiver can accomplish joint symbol timing synchronization and demodulation based on oversampled waveform sequences directly. The prevalent Transformer structures, suitable for excavating sequential correlation of complex sources and waveform sequences, are considered as the backbone of NN receivers. Under the cases of clean label and noisy label, we conceive two novel training algorithms and interpret their principles in theory. The clean label bits are accessible from original source encoding schemes, while noisy label bits are available from the decision results of canonical receivers with independently noised waveform sequences. When noisy labels possess sufficiently small error rates, optimized NN receiver is competitive with that adopting the accurate labels. As for natural language text sources, simulation results show that the proposed NN receivers' performances are superior to the classical receivers without a priori knowledge, especially when high spectral efficiency modulation schemes are encountered and random noise is significant.
MoreTranslated text
AI Read Science
Must-Reading Tree
Example
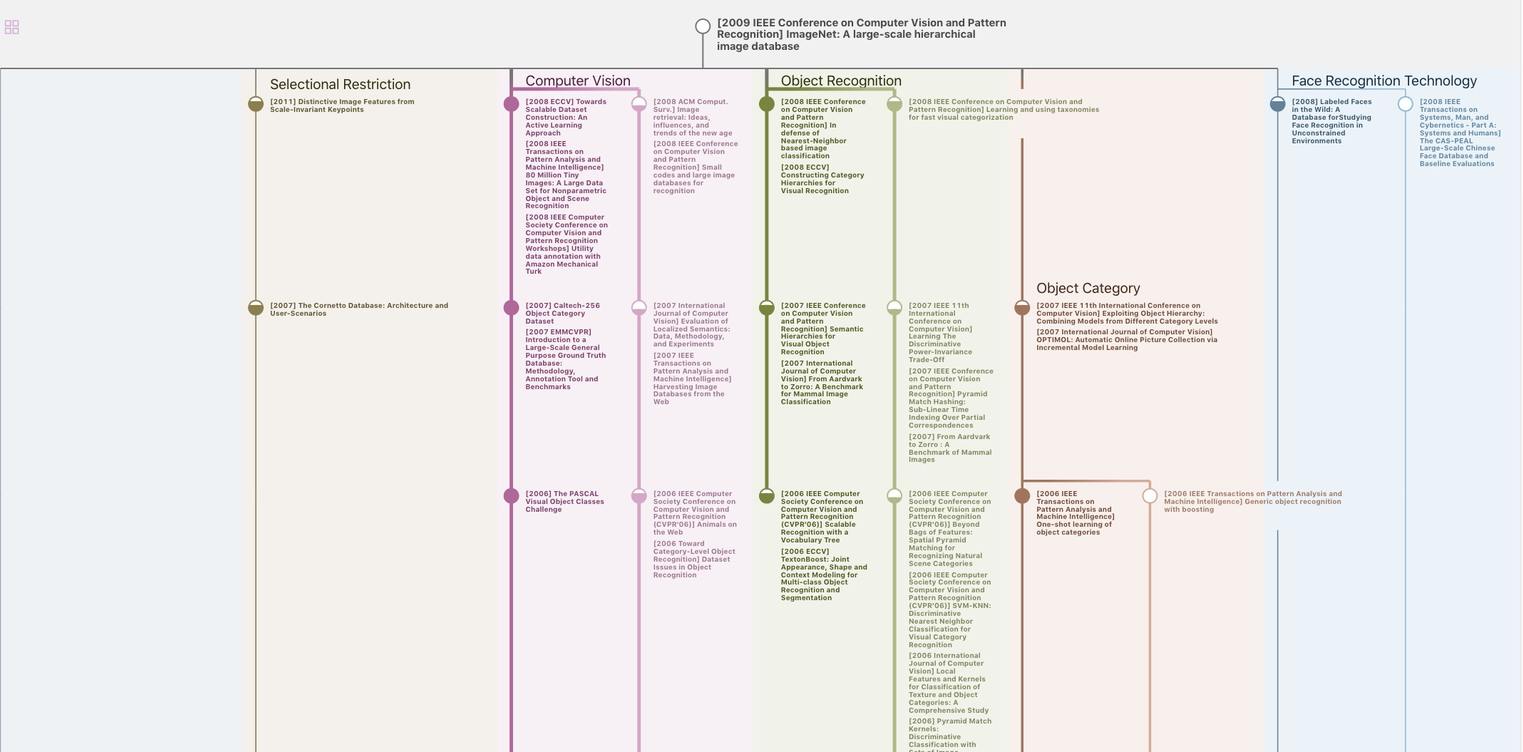
Generate MRT to find the research sequence of this paper
Chat Paper
Summary is being generated by the instructions you defined