Stable Causal Feature Selection based on Direct Causal Effect Estimation.
HPCC/DSS/SmartCity/DependSys(2022)
摘要
Feature selection is an important technique and tool for selecting relevant and critical features from the high-dimensional data in many tasks of data mining and machine learning. However, existing algorithms mainly rely on the associations between features and response variables such that they lack stability and may result in wrong conclusions. To improve the interpretability and stability of feature selection algorithms, we propose a stable causal feature selection algorithm based on the direct causal effect estimation, which achieves interpretable feature selection by considering the causal relationship between features and response variables. Specifically, we develop a theorem based on the graphical causal model to estimate the direct causal effects for supporting the proposed stable causal feature selection algorithm. Moreover, the causal feature selection algorithm is applied for a real-world application, i.e. earthquake prediction by utilising a neural network model, BiLSTM. Abundant experiments carried out on synthetic and real-world data sets demonstrate that the proposed stable causal feature selection algorithm has better performance than the baselines.
更多查看译文
关键词
Stable Feature Selection,Markov Blanket,Direct Causal Effect Estimation,Earthquake Prediction
AI 理解论文
溯源树
样例
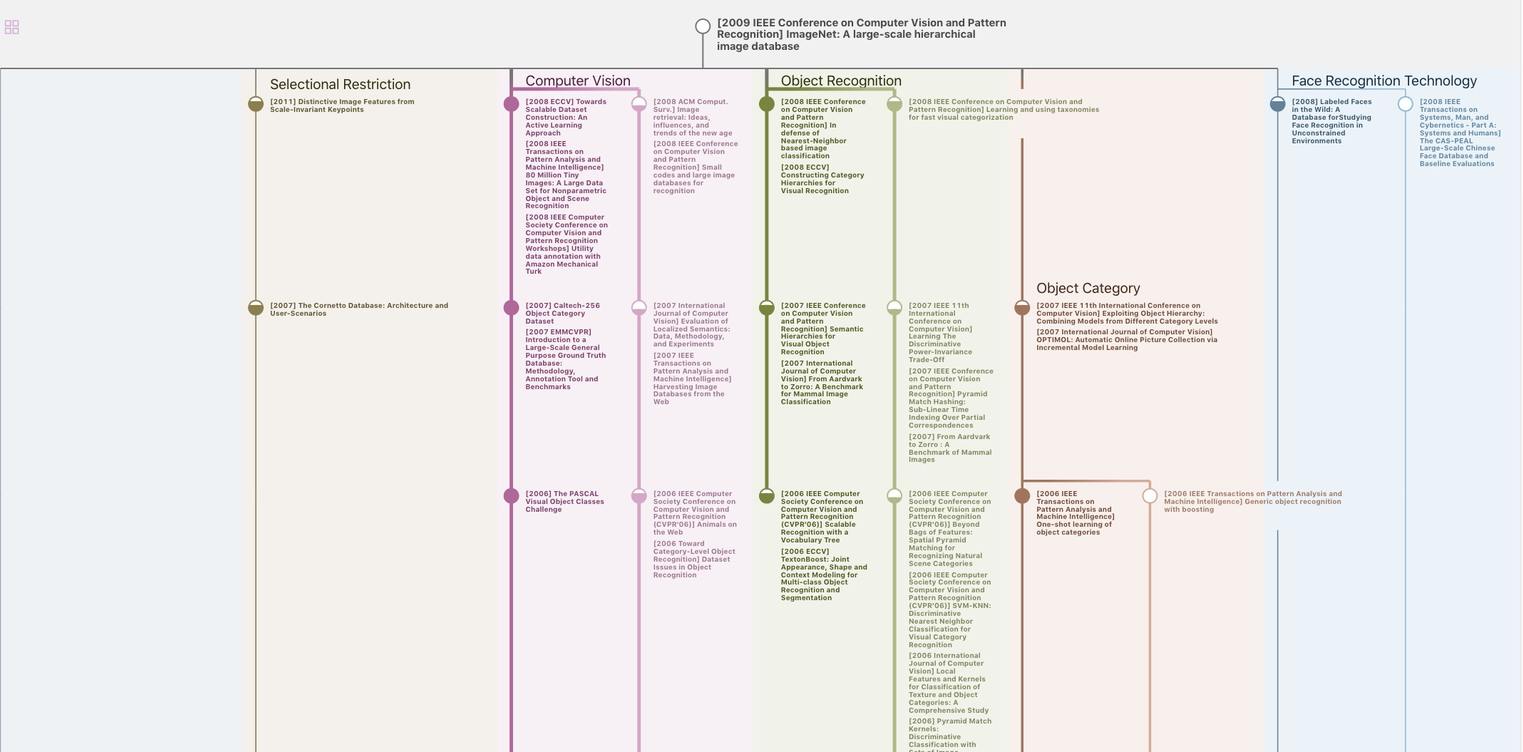
生成溯源树,研究论文发展脉络
Chat Paper
正在生成论文摘要