Fine-grained Spatiotemporal Features-Based for Anomaly Detection in Microservice Systems.
HPCC/DSS/SmartCity/DependSys(2022)
Abstract
More and more applications use microservice architecture. Protecting the reliability of the microservice system is very important for the stable operation of applications. However, the complexity of microservice systems poses a great challenge to operation and maintenance. Researchers have proposed a series of anomaly detection algorithms, which can automatically detect the anomalies of cloud systems in time. However, for the microservice system with a complex spatial structure, there is no effective method to represent the fine-grained features of the internal metric level of the microservice. To solve this problem, we propose a fine-grained metric-level spatial feature graph TopoMetrics and use a spatiotemporal neural network STAD to obtain the spatiotemporal features of microservices, which can accurately detect the anomalies of complex microservices. We compare STAD with the most advanced algorithms in three open microservice workloads. The experimental results show that the average precision of STAD is significantly higher than that of the most advanced baseline method.
MoreTranslated text
Key words
Anomaly Detection,Graph Neural Networks,Microservice Systems
AI Read Science
Must-Reading Tree
Example
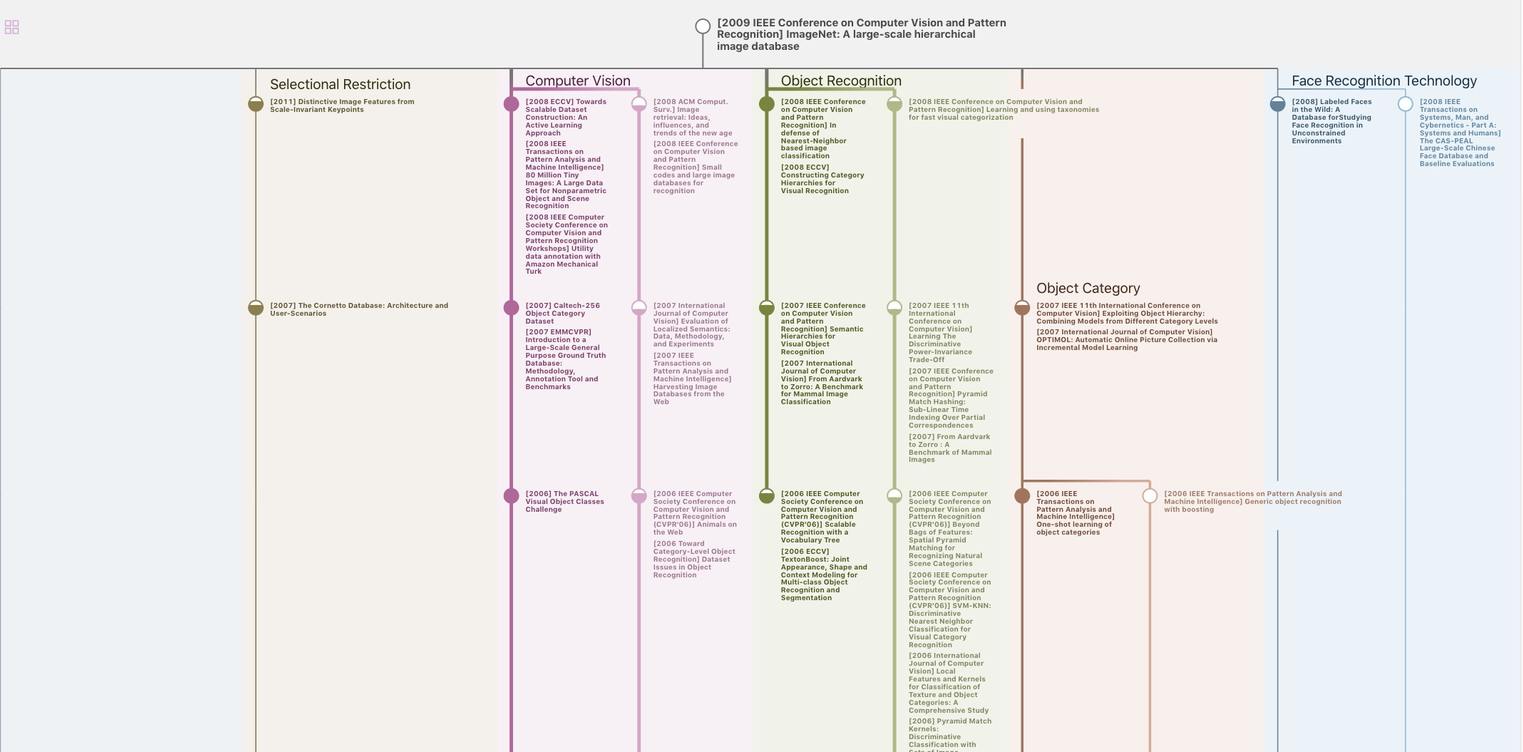
Generate MRT to find the research sequence of this paper
Chat Paper
Summary is being generated by the instructions you defined