U-Net Based Discriminator for Real-World Super-Resolution.
ICTAI(2022)
摘要
In recent years, single image super-resolution (SISR) deep learning techniques have achieved remarkable improvements in recovering a high-resolution (HR) image from an observed low-resolution (LR) input. Nevertheless, these proposed methods fail in many real-world scenarios since their models are usually trained using a pre-defined degradation process from HR ground truth images to LR ones. To address this issue, new architectures have been proposed focusing on adopting more complicated degradation models to emulate real-world degradation achieving prominent performance but still limited to certain kinds of inputs and dropping considerably in other cases. In this paper, we present a GAN structure for blind super-resolution tasks, applying a technique that has not been very commonly used in SISR proposals: a U-Net architecture as a discriminator of the GAN network. Adding this structural change will encourage the discriminator to focus more on semantic and structural changes between real and fake images and to attend less to domain-preserving perturbations. In addition, the loss function of the generator was modified by adding the LPIPS loss function for the perceptual loss and a per-pixel consistency regularization technique based on the CutMix data augmentation. Numerous novel solutions that have been proposed recently involve powerful deep learning techniques. The proposed model was trained using the DF2K dataset employing a degradation framework for real-world images by estimating blur kernels and real noise distributions to obtain more realistic LR samples. Finally, we present a benchmark comparing our results with other methods in the state-of-theart. The commonly-used evaluation metrics for image restoration PSNR, SSIM, and LPIPS were used for this evaluation.
更多查看译文
关键词
Deep Learning, Degradation Modeling, Image Super-Resolution, Loss Functions, U-Net Discriminator
AI 理解论文
溯源树
样例
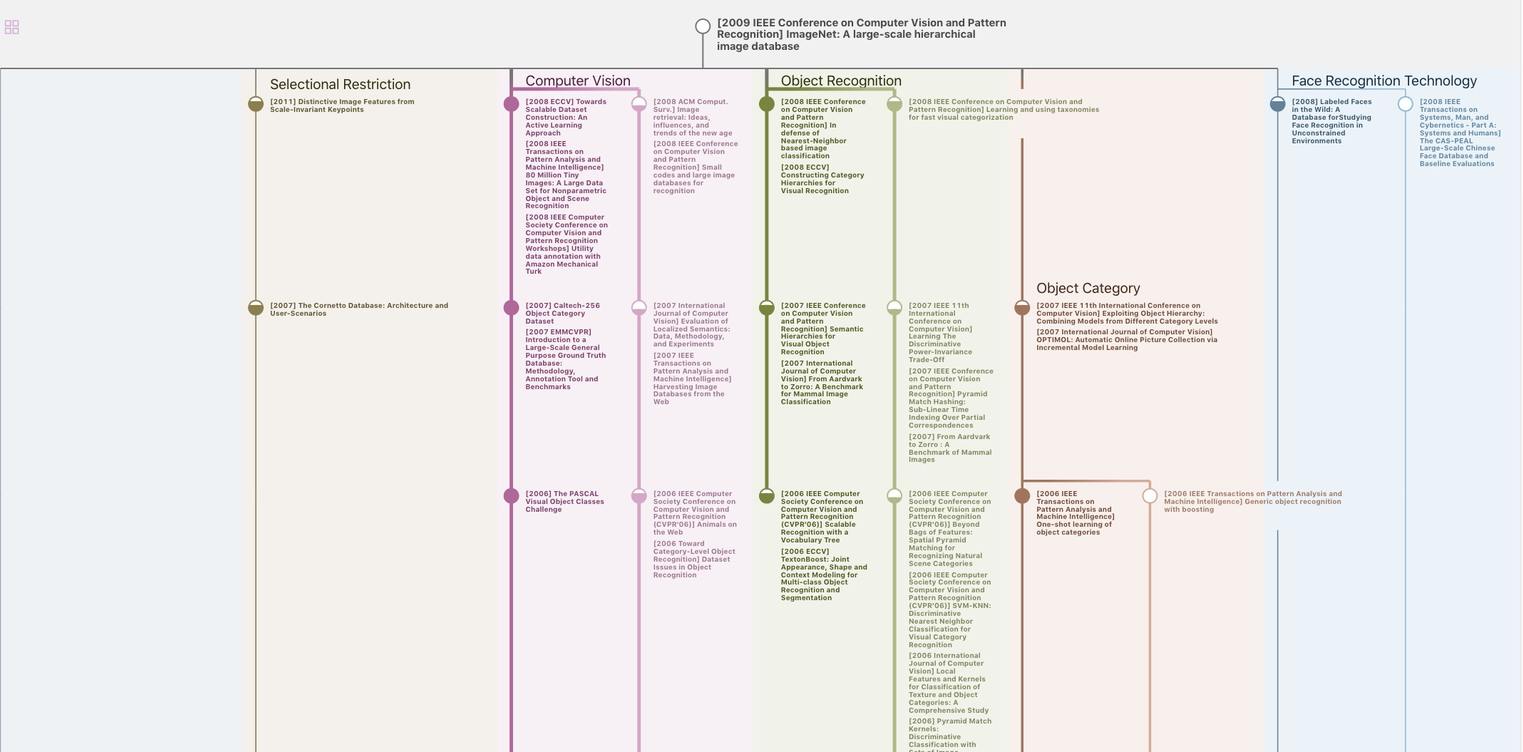
生成溯源树,研究论文发展脉络
Chat Paper
正在生成论文摘要