Edge Assisted Asymmetric Convolution Network for MR Image Super-Resolution.
MMM (2)(2023)
摘要
High-resolution magnetic resonance (MR) imaging is beneficial for accurate disease diagnosis and subsequent analysis. Currently, the single image super-resolution (SR) technique is an effective and less costly alternative technique to improve the spatial resolution of MR images. Structural information in MR images is crucial during clinical diagnosis, but it is often ignored by existing deep learning MR image SR technique. Consequently, we propose edge assisted feature extraction block (EAFEB), which can efficiently extract the content and edge features from low-resolution (LR) images, allowing the network to focus on both content and geometric structure. To fully utilize the features extracted by EAFEB, an asymmetric convolutional group (ACG) is proposed, which can balance structural feature preservation and content feature extraction. Moreover, we design a novel contextual spatial attention (CSA) method to facilitate the network focus on critical information. Experiment results in various MR image sequences, including T1, T2, and PD, show that our Edge Assisted Asymmetric Convolution Network (EAACN) has superior results relative to recent leading SR models.
更多查看译文
关键词
Magnetic Resonance Imaging, Super-Resolution, Edge Assisted, Asymmetric Convolution, Contextual Spatial Attention
AI 理解论文
溯源树
样例
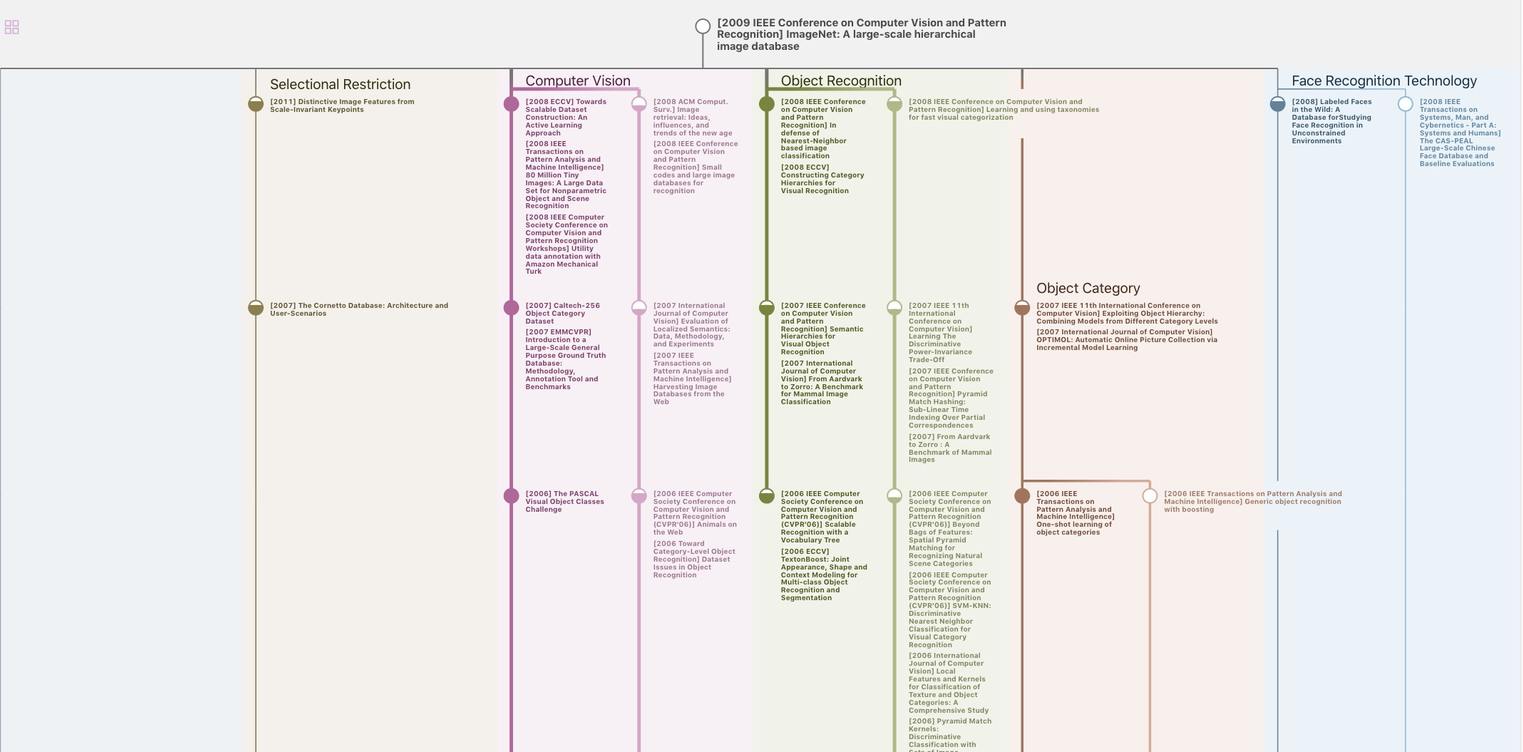
生成溯源树,研究论文发展脉络
Chat Paper
正在生成论文摘要