Weighted Multi-view Clustering Based on Internal Evaluation.
MMM (2)(2023)
摘要
As real-world data are often represented by multiple sets of features in different views, it is desirable to improve clustering results with respect to ordinary single-view clustering by making use of the consensus and complementarity among different views. For this purpose, weighted multi-view clustering is proposed to combine multiple individual views into one single combined view, which is used to generate the final clustering result. In this paper we present a simple yet effective weighted multi-view clustering algorithm based on internal evaluation of clustering results. Observing that an internal evaluation criterion can be used to estimate the quality of clustering results, we propose to weight different views to maximize the clustering quality in the combined view. We firstly introduce an implementation of the Dunn index and a heuristic method to determine the scale parameter in spectral clustering. Then an adaptive weight initialization and updating method is proposed to improve the clustering results iteratively. Finally we do spectral clustering in the combined view to generate the clustering result. In experiments with several publicly available image and text datasets, our algorithm compares favorably or comparably with some other algorithms.
更多查看译文
关键词
internal evaluation,multi-view
AI 理解论文
溯源树
样例
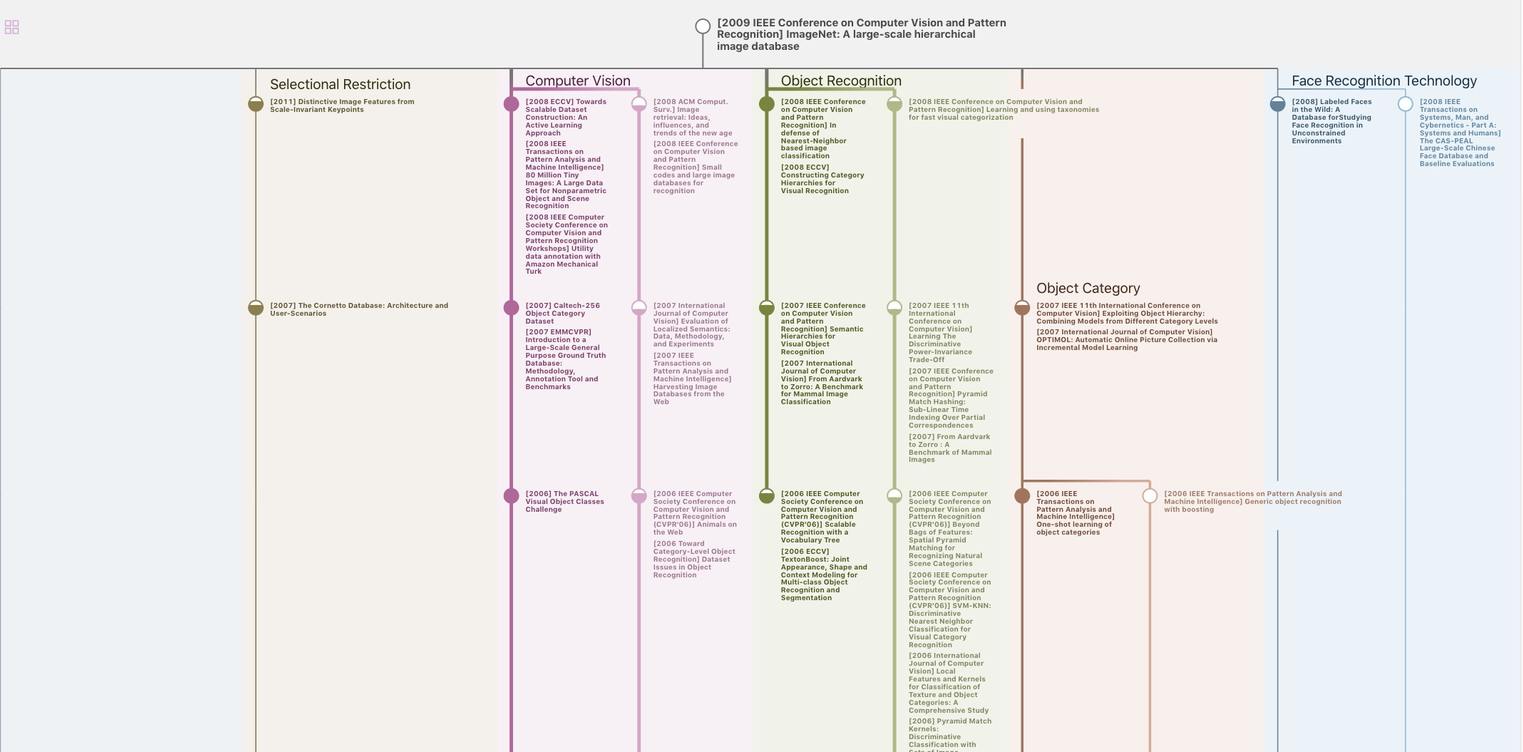
生成溯源树,研究论文发展脉络
Chat Paper
正在生成论文摘要