In Situ Augmentation for Defending Against Adversarial Attacks on Text Classifiers.
ICONIP (3)(2022)
摘要
In text classification, recent research shows that adversarial attack methods can generate sentences that dramatically decrease the classification accuracy of state-of-the-art neural text classifiers. However, very few defense methods have been proposed against these generated high-quality adversarial sentences. In this paper, we propose LMAg (Language-Model-based Augmentation using Gradient Guidance), an in situ data augmentation method as a defense mechanism effective in two representative defense setups. Specifically, LMAg transforms input text during the test time. It uses the norm of the gradient to estimate the importance of a word to the classifier’s prediction, then replaces those words with alternatives proposed by a masked language model. LMAg is an additional protection layer on the classifier that counteracts the perturbations made by adversarial attack methods, thus can protect the classifier from adversarial attack without additional training. Experimental results show that LMAg can improve after-attack accuracy of BERT text classifier by $$51.5\%$$ and $$17.3\%$$ for two setups respectively.
更多查看译文
关键词
adversarial attacks,text classifiers,situ augmentation
AI 理解论文
溯源树
样例
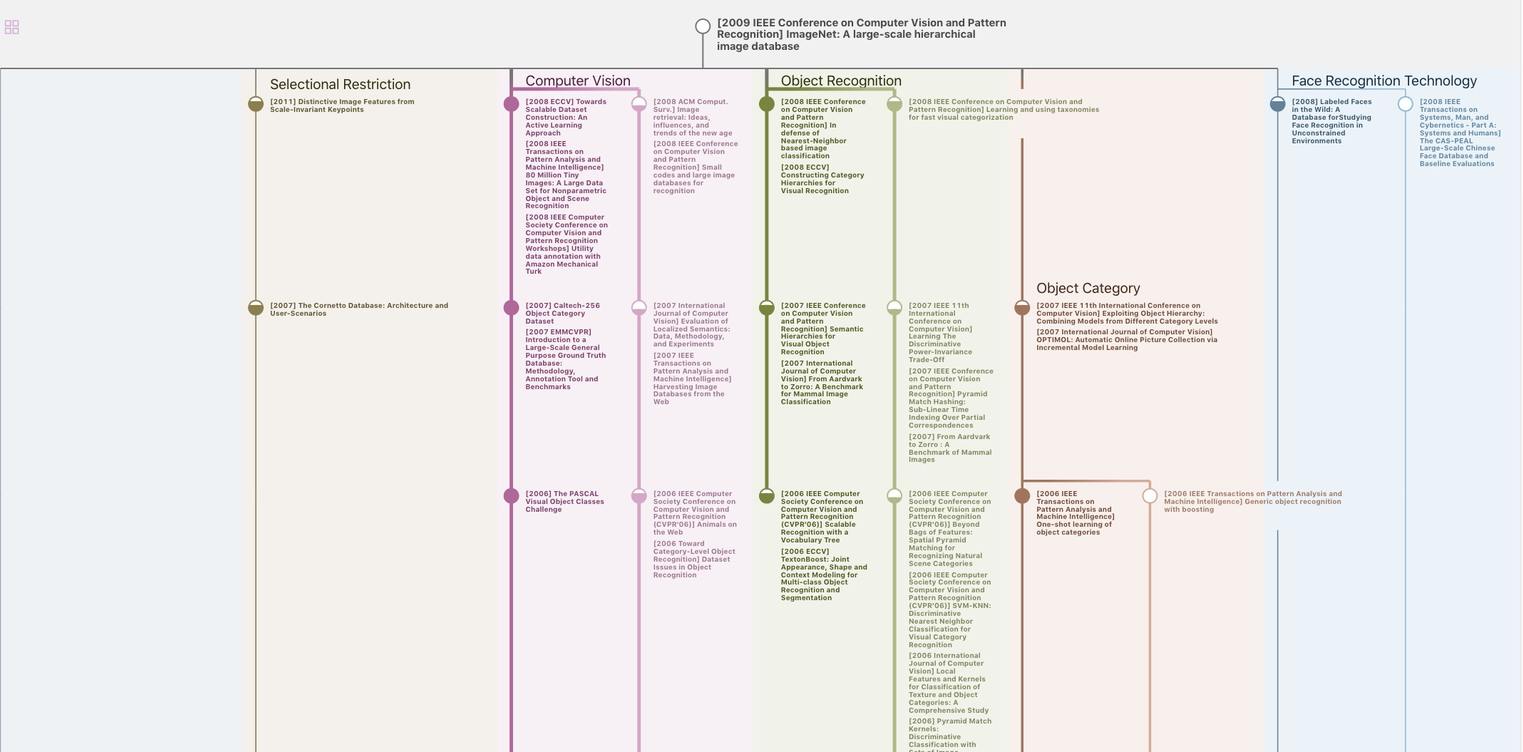
生成溯源树,研究论文发展脉络
Chat Paper
正在生成论文摘要