Adaptive Graph Recurrent Network for Multivariate Time Series Imputation.
ICONIP (5)(2022)
摘要
Multivariate time series inherently involve missing values for various reasons, such as incomplete data entry, equipment malfunctions, and package loss in data transmission. Filling missing values is important for ensuring the performance of subsequent analysis tasks. Most existing methods for missing value imputation neglect inter-variable relations in time series. Although graph-based methods can capture such relations, the design of graph structures commonly requires domain knowledge. In this paper, we propose an adaptive graph recurrent network (AGRN) that combines graph and recurrent neural networks for multivariate time series imputation. Our model can learn variable- and time-specific dependencies effectively without extra information such as domain knowledge. Our extensive experiments on real-world datasets demonstrate our model’s superior performance to state-of-the-art methods.
更多查看译文
关键词
multivariate time series imputation,graph,adaptive
AI 理解论文
溯源树
样例
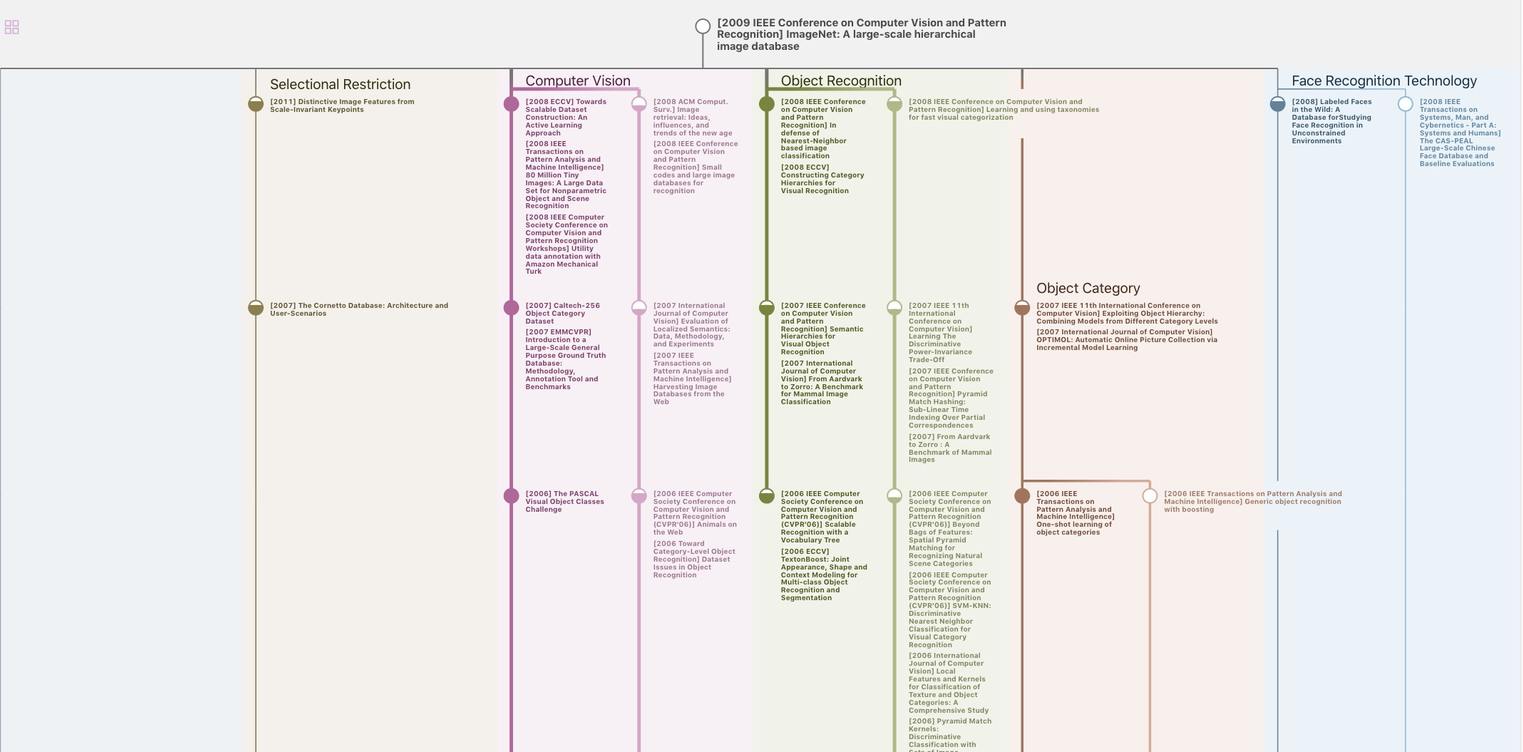
生成溯源树,研究论文发展脉络
Chat Paper
正在生成论文摘要