Continuous prediction of finger joint angles based on time series feature fusion CNN.
RICAI(2022)
Abstract
Continuous estimation of joints based on surface electromyography (sEMG) signals has been widely used in smooth motion of robots. Currently, deep learning techniques have become the main means of continuous motion estimation. Although the deep learning technique can reduce the workload of manual features by feature learning, its learned features lack the temporal information of continuous joint activity and cannot provide an effective description of the continuous joint trajectory. Therefore, this paper proposes a method for continuous finger joint angle prediction based on time series feature fusion convolutional neural network (CNN), which first extracts feature information of sEMG signals by manual features, then constructs time series, then inputs to convolutional neural network to extract deep features, and finally estimates finger joint angle. The experimental results show that the CNN method based on time series feature fusion is significantly better than the methods of CNN and multilayer perceptron (MLP). It is proved that the method in this study can effectively extract the features of sEMG signals as well as better prediction performance, which provides a new idea for continuous motion estimation methods.
MoreTranslated text
AI Read Science
Must-Reading Tree
Example
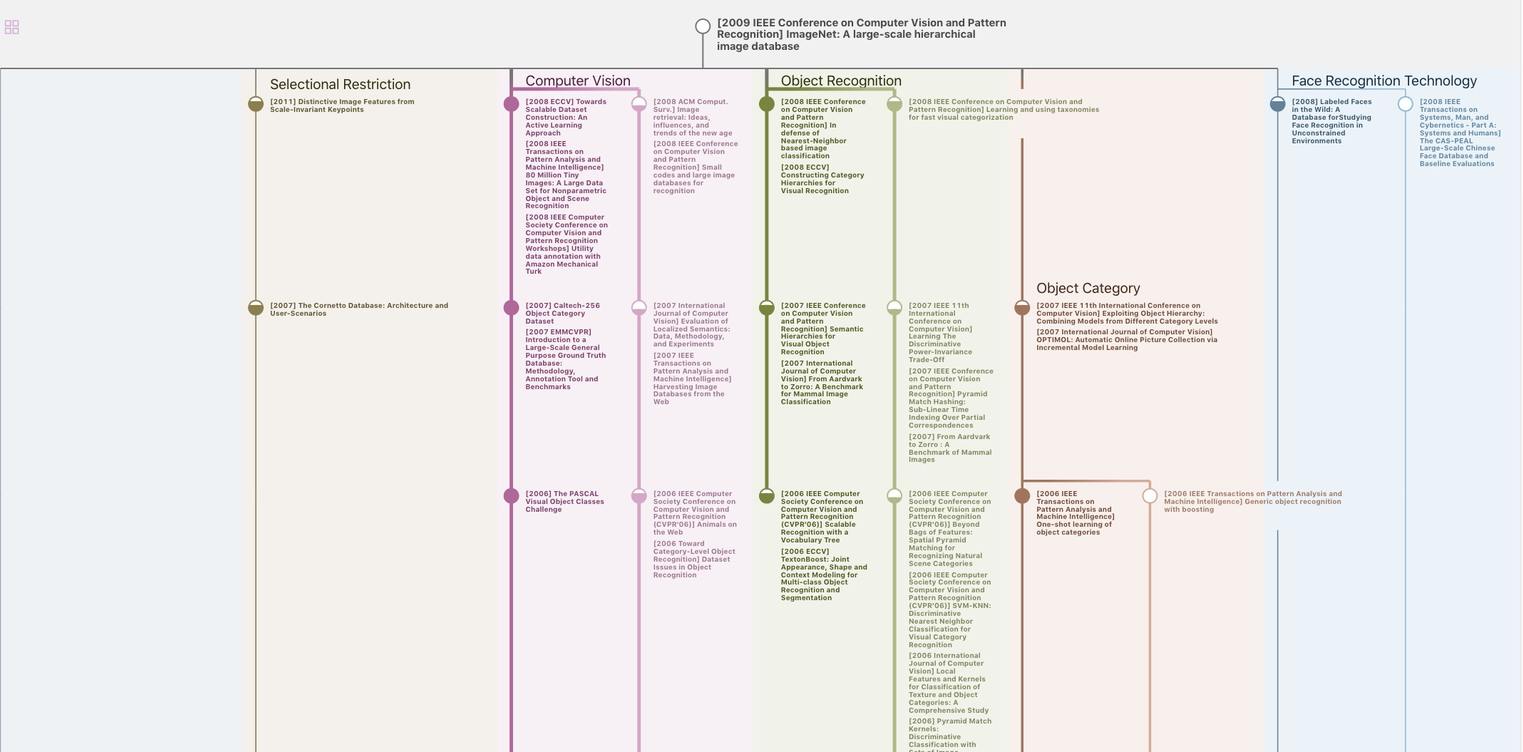
Generate MRT to find the research sequence of this paper
Chat Paper
Summary is being generated by the instructions you defined