Instrumental Conditioning with Neuromodulated Plasticity on SpiNNaker.
ICONIP (2)(2022)
Abstract
We present a work-in-progress on implementing reinforcement learning by instrumental conditioning on SpiNNaker. Animals learn to behave by exploring the changing environment around them such that, over a period of time, their behaviour gives a good outcome (reward) i.e. a perception of ‘satisfaction’. While inspired by animal learning, reinforcement learning adopts a goal-directed strategy of maximising rewards in a dynamic environment. Instrumental conditioning is a strategy to strengthen the association between an action and the environmental state when the state-action pair is rewarded i.e. the reward is instrumental in forming the association. However, in the real world, the delivery of a reward is often delayed in time, known as the distal reward problem. Using the concept of eligibility traces and spike-time dependant plasticity (STDP), Izhikevich (2007) simulated both classical and instrumental conditioning in a spiking neural network with Dopamine (DA)-modulated STDP. The current implementation of DA-modulated plasticity on SpiNNaker using trace-based STDP is reported by Mikaitas et al. (2018), who demonstrated classical conditioning with a similar experimental set up as Izhikevich. Our results show that using delayed DA-modulation of STDP on SpiNNaker, we can condition a neural population to maximise its reward over a period of time by firing at a higher rate than another competing population. Ongoing work is looking into a dynamic conditioning scenario where different actions can be selected within the same run as is the case in real world scenarios.
MoreTranslated text
Key words
neuromodulated plasticity,instrumental conditioning
AI Read Science
Must-Reading Tree
Example
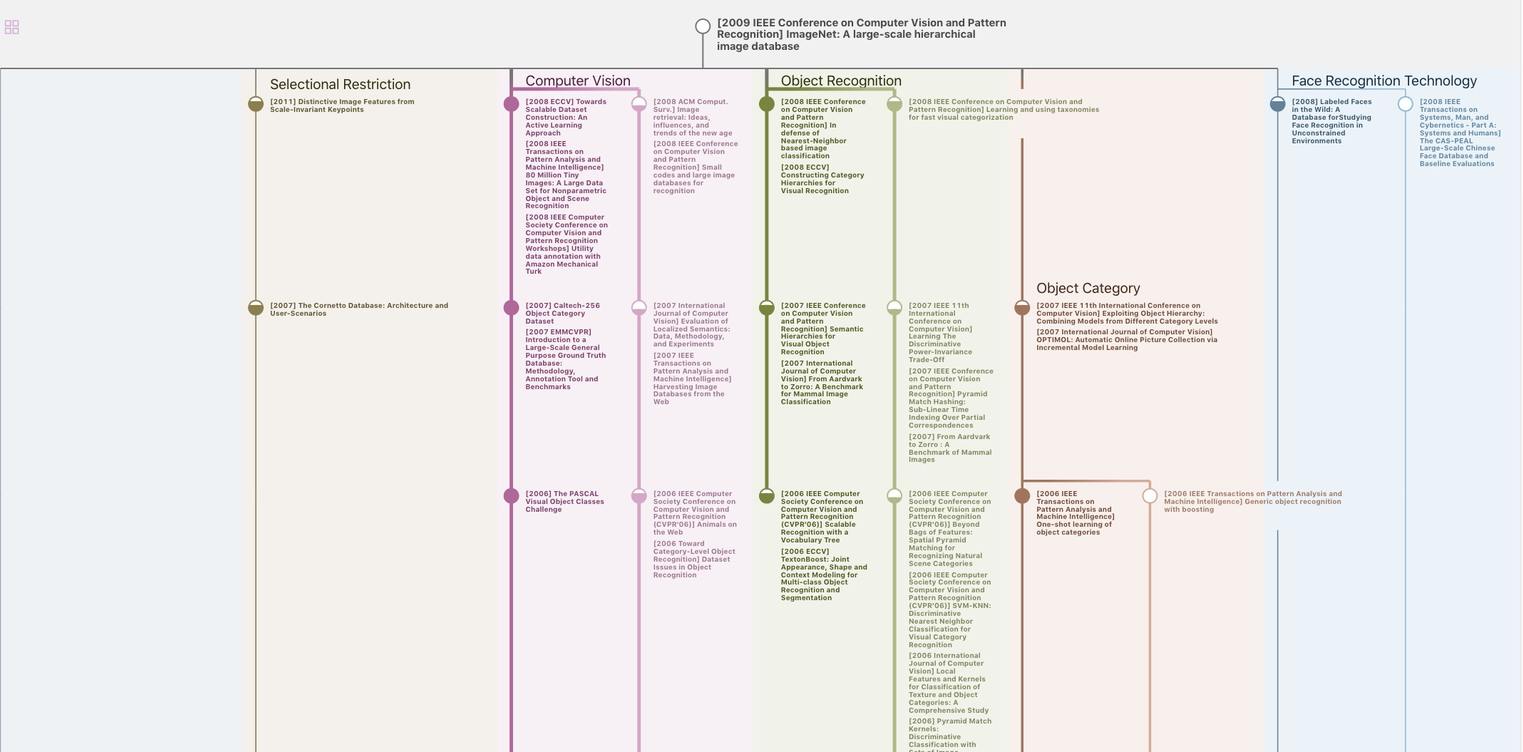
Generate MRT to find the research sequence of this paper
Chat Paper
Summary is being generated by the instructions you defined