VAAC: V-value Attention Actor-Critic for Cooperative Multi-agent Reinforcement Learning.
ICONIP (1)(2022)
Abstract
This paper explores value-decomposition methods in cooperative multi-agent reinforcement learning (MARL) under the paradigm of centralized training with decentralized execution. These methods decompose a global shared value into individual ones to guide the learning of decentralized policies. While Q-value decomposition methods such as QMIX show state-of-the-art performance, V-value decomposition methods are proposed to obtain a reasonable trade-off between training efficiency and algorithm performance under the A2C training paradigm. However, existing V-value decomposition methods lack theoretical analysis of the relation between the global V-value and local V-values, and do not explicitly consider the influence of individuals on the total system, which degrades their performance. To address these problems, this paper proposes a novel approach called V-value Attention Actor-Critic (VAAC) for cooperative MARL. We theoretically derive a general decomposing formulation of the global V-value in terms of local V-values of individual agents, and implement it with a multi-head attention formation to model the impact of individuals on the whole system for interpretability of decomposition. Evaluations on the challenging StarCraft II micromanagement task show that VAAC achieves a better trade-off between training efficiency and algorithm performance, and provides interpretability for its decomposition process.
MoreTranslated text
Key words
reinforcement learning,attention,v-value,actor-critic,multi-agent
AI Read Science
Must-Reading Tree
Example
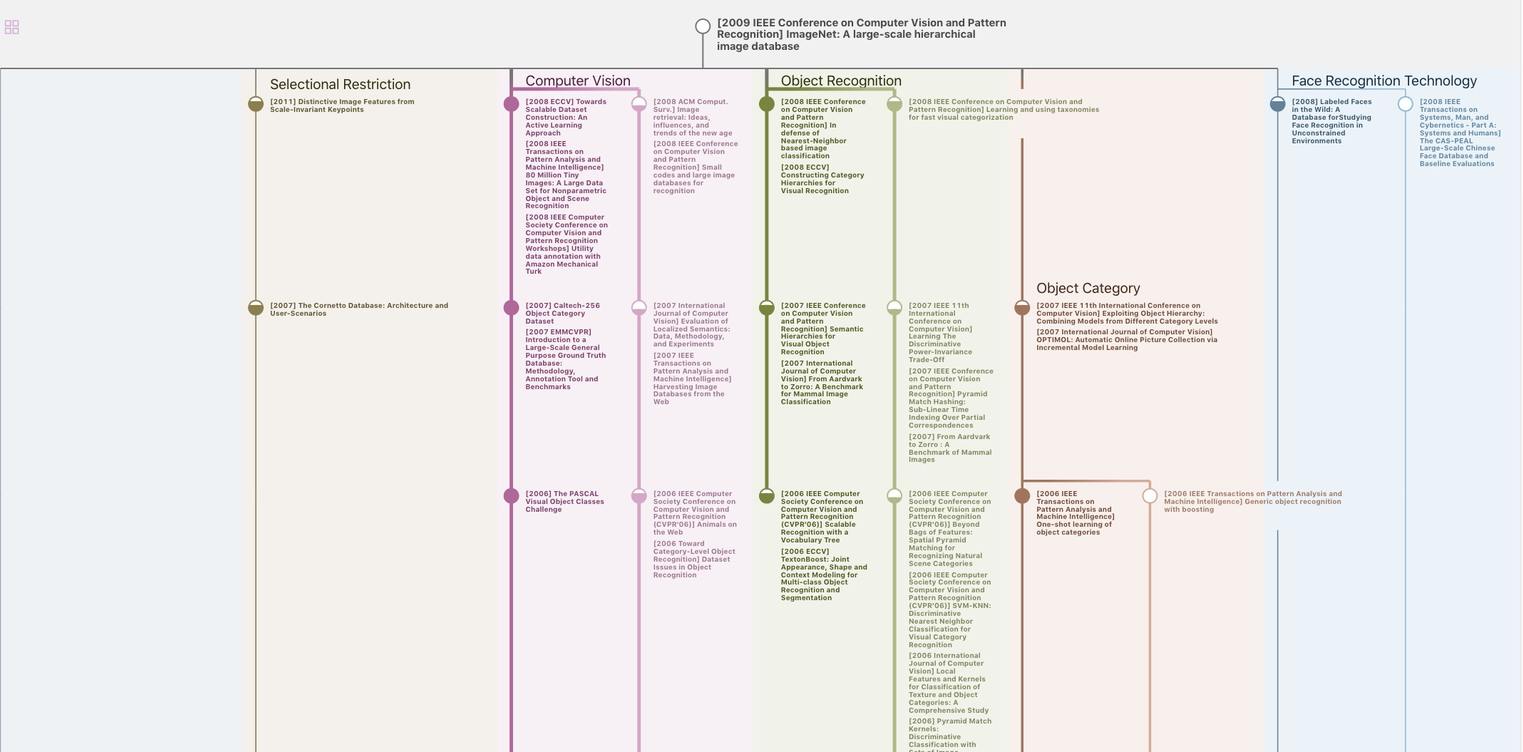
Generate MRT to find the research sequence of this paper
Chat Paper
Summary is being generated by the instructions you defined