PetPS: Supporting Huge Embedding Models with Persistent Memory.
Proc. VLDB Endow.(2023)
摘要
Embedding models are effective for learning high-dimensional sparse data. Traditionally, they are deployed in DRAM parameter servers (PS) for online inference access. However, the ever-increasing model capacity makes this practice suffer from both high storage costs and long recovery time. Rapidly developing Persistent Memory (PM) offers new opportunities to PSs owing to its large capacity at low costs, as well as its persistence, while the application of PM also faces two challenges including high read latency and heavy CPU burden. To provide a low-cost but still high-performance parameter service for online inferences, we introduce PetPS, the first production-deployed PM parameter server. (1) To escape with high PM latency, PetPS introduces a PM hash index tailored for embedding model workloads, to minimize PM access. (2) To alleviate the CPU burden, PetPS offloads parameter gathering to NICs, to avoid CPU stalls when accessing parameters on PM and thus improve CPU efficiency. Our evaluation shows that PetPS can boost throughput by 1.3 -- 1.7X compared to PSs that use state-of-the-art PM hash indexes, or get 2.9 -- 5.5X latency reduction with the same throughput. Since 2020, PetPS has been deployed in Kuaishou, one world-leading short video company, and successfully reduced TCO by 30% without performance degradation.
更多查看译文
关键词
persistent memory,embedding,models
AI 理解论文
溯源树
样例
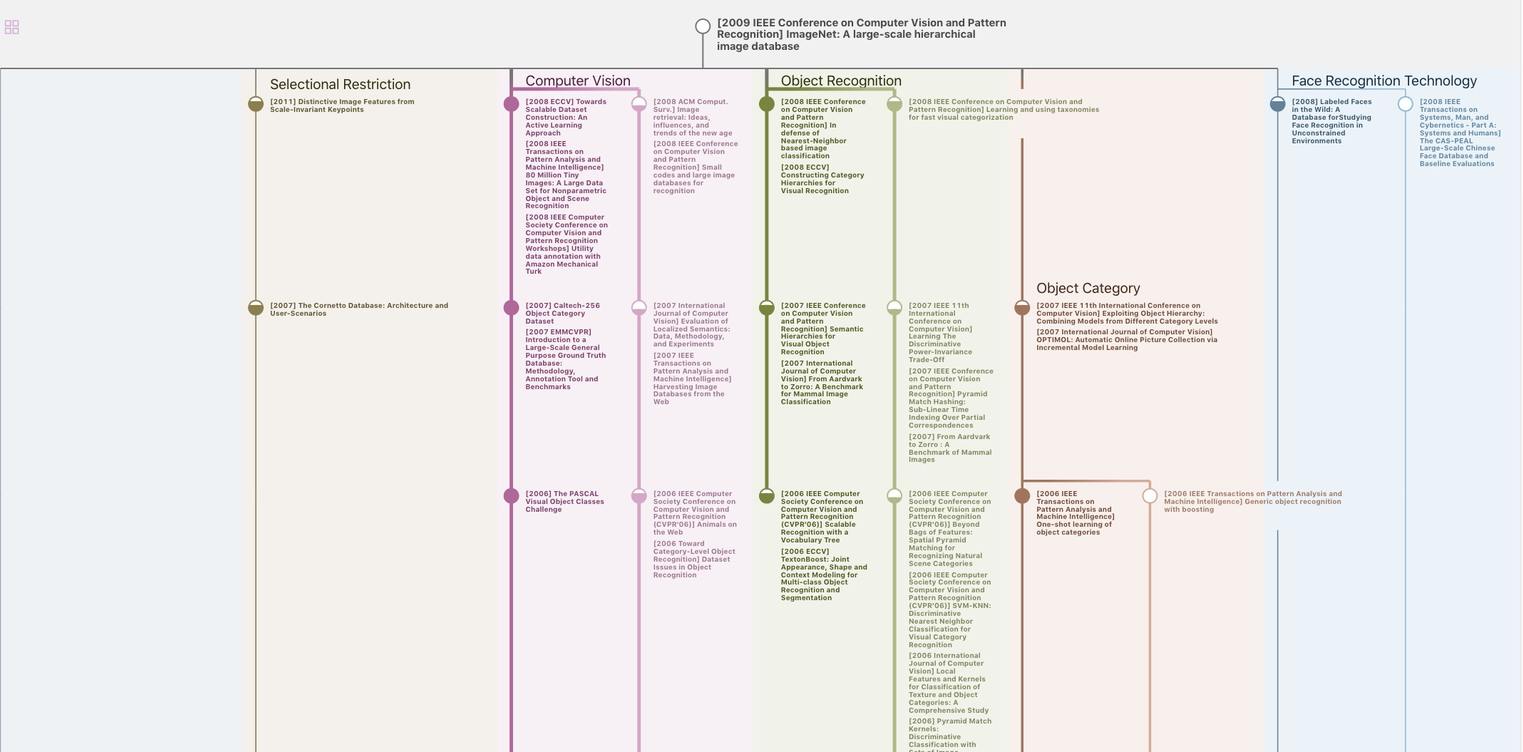
生成溯源树,研究论文发展脉络
Chat Paper
正在生成论文摘要