Predicting regrowth of low-grade gliomas after radiotherapy.
PLoS Comput. Biol.(2023)
摘要
Diffuse low grade gliomas are invasive and incurable brain tumors that inevitably transform into higher grade ones. A classical treatment to delay this transition is radiotherapy (RT). Following RT, the tumor gradually shrinks during a period of typically 6 months to 4 years before regrowing. To improve the patient's health-related quality of life and help clinicians build personalized follow-ups, one would benefit from predictions of the time during which the tumor is expected to decrease. The challenge is to provide a reliable estimate of this regrowth time shortly after RT (i.e. with few data), although patients react differently to the treatment. To this end, we analyze the tumor size dynamics from a batch of 20 high-quality longitudinal data, and propose a simple and robust analytical model, with just 4 parameters. From the study of their correlations, we build a statistical constraint that helps determine the regrowth time even for patients for which we have only a few measurements of the tumor size. We validate the procedure on the data and predict the regrowth time at the moment of the first MRI after RT, with precision of, typically, 6 months. Using virtual patients, we study whether some forecast is still possible just three months after RT. We obtain some reliable estimates of the regrowth time in 75% of the cases, in particular for all "fast-responders". The remaining 25% represent cases where the actual regrowth time is large and can be safely estimated with another measurement a year later. These results show the feasibility of making personalized predictions of the tumor regrowth time shortly after RT. Author summaryThis work addresses the question of making predictions on the remission time patients suffering from brain tumors (gliomas) may expect after a radiotherapy treatment. It is a very crucial question often asked by patient's to their practician in order to plan some important life projects (as traveling, retiring, having children) but that is difficult to answer since there is a large variability among patient reactions to radiotherapy. We then build a statistical model using the recorded evolution of the glioma size of previously treated patients. It allows, for the first time, to make these predictions, with a few months accuracy.
更多查看译文
关键词
radiotherapy,regrowth,low-grade
AI 理解论文
溯源树
样例
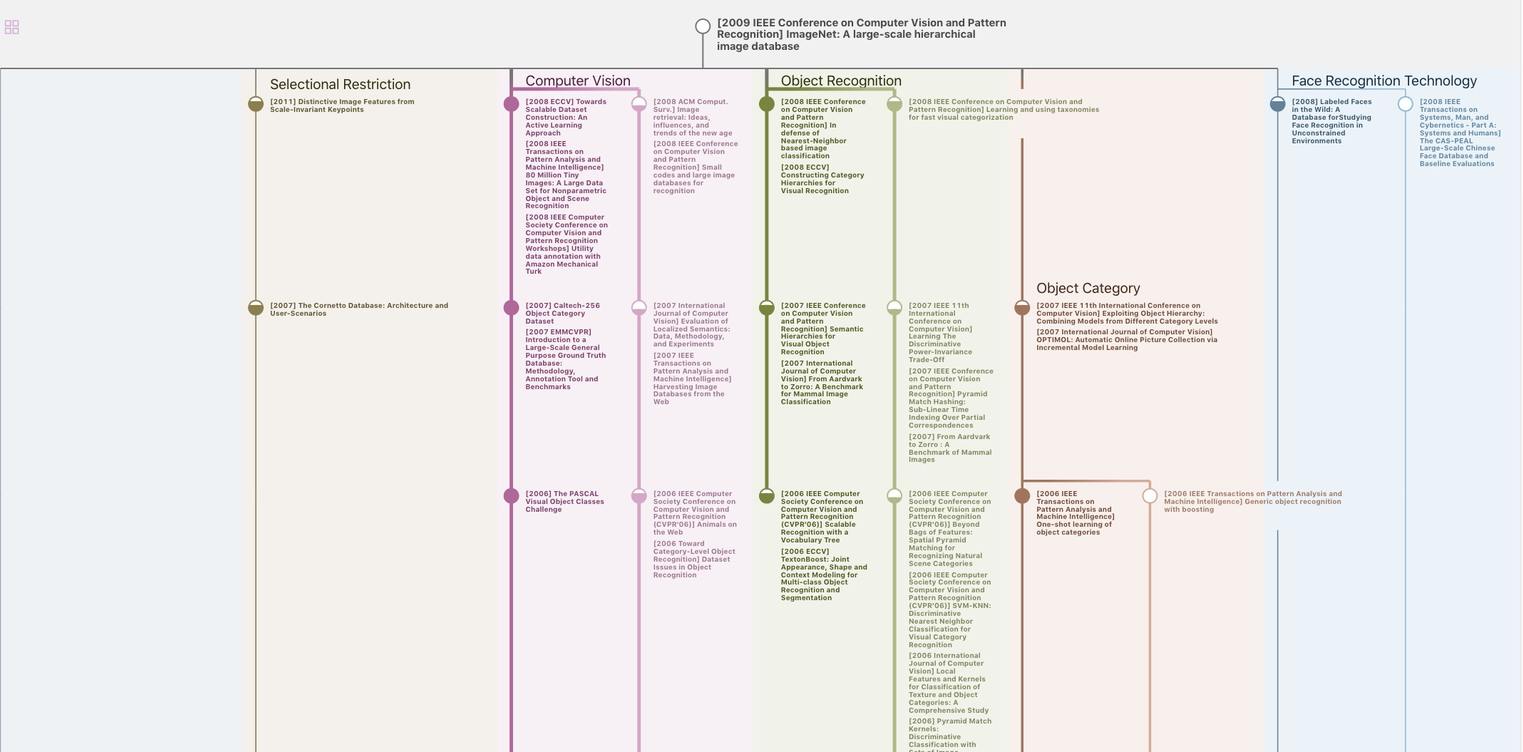
生成溯源树,研究论文发展脉络
Chat Paper
正在生成论文摘要