Parameter Learning for the Nonlinear System Described by a Class of Hammerstein Models
Circuits Syst. Signal Process.(2022)
摘要
A novel parameter learning scheme using multi-signals is proposed for estimating parameters of the Hammerstein nonlinear model in this research. The Hammerstein nonlinear model consists of a static nonlinear block and a dynamic linear block, and the designed multi-signals that composed of separable signals and random signals are used for learning separately the nonlinear block parameters and the linear block parameters. Firstly, the linear block parameters are learned by virtue of correlation analysis algorithm based on input–output data of separable signals, which effectively deals with the unmeasurable variable of the Hammerstein model. In addition, auxiliary error-based probability density function strategy is employed to estimate the nonlinear block parameters with the help of random signals, which makes the model error present normal distribution, as well as improves model accuracy effectively. Studies with a numerical simulation and a typical industrial process demonstrate that the developed approach obtains high learning accuracy and small modeling error, which verifies the accuracy and efficiency of the developed parameter learning scheme.
更多查看译文
关键词
Hammerstein nonlinear model,Correlation analysis,Probability density function,Parameter learning
AI 理解论文
溯源树
样例
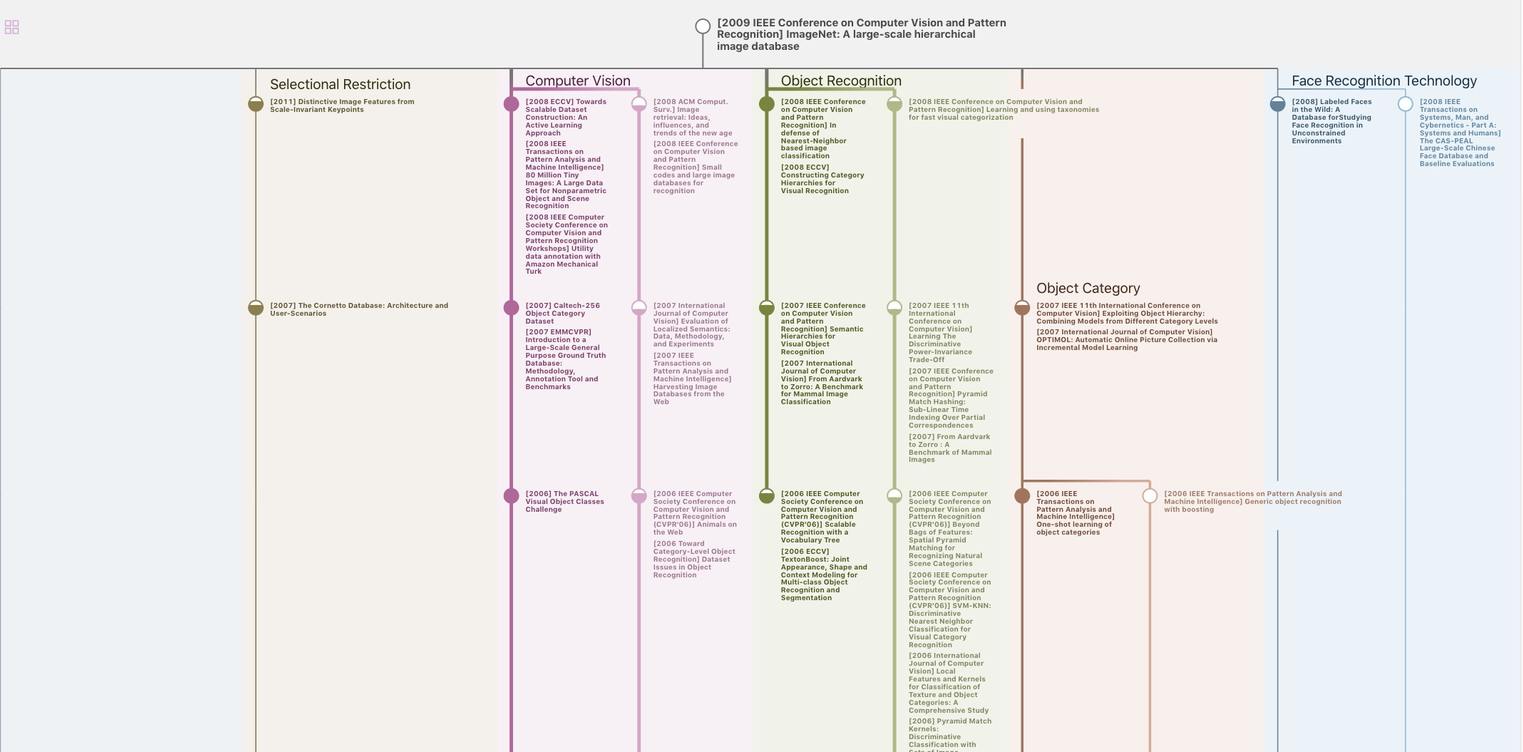
生成溯源树,研究论文发展脉络
Chat Paper
正在生成论文摘要