Robust unsupervised network intrusion detection with self-supervised masked context reconstruction.
Comput. Secur.(2023)
摘要
Modern network intrusion detection systems always utilize deep learning to improve their intelligence and feature learning abilities. To overcome the difficulties of accessing a large amount of labeled data and achieve early warning, lots of intrusion detection systems focus on unsupervised anomaly detection methods. However, most unsupervised anomaly detection methods ignore the temporal context and anomaly contamination in network intrusion data, which leads to suboptimal detection results. By considering the above practical problems, we propose a robust unsupervised intrusion detection system, i.e, RUIDS, by introducing a masked context reconstruction module into a transformer-based self-supervised learning scheme. The self-supervised learning scheme is designed to learn the intrinsic relationship within temporal contexts. And the masked context reconstruction module can learn more robust representations which are less sensitive to anomaly contamination. Extensive experiments on four intrusion datasets are conducted to show the effectiveness and robustness of RUIDS. Specifically, RUIDS achieves 9.04% and 9.58% improvements over the second-best method on the UNSW-NB15 and CICIDS-WED datasets in terms of AUC value respectively. We also test the robustness of our method with different anomaly contamination ratios, and our algorithm’s performance has hardly decreased. The ablation study confirmed the effectiveness of the self-supervised learning scheme and the masked context reconstruction module.
更多查看译文
关键词
Network intrusion detection, Unsupervised learning, Self -supervised learning, Temporal context, Anomaly detection
AI 理解论文
溯源树
样例
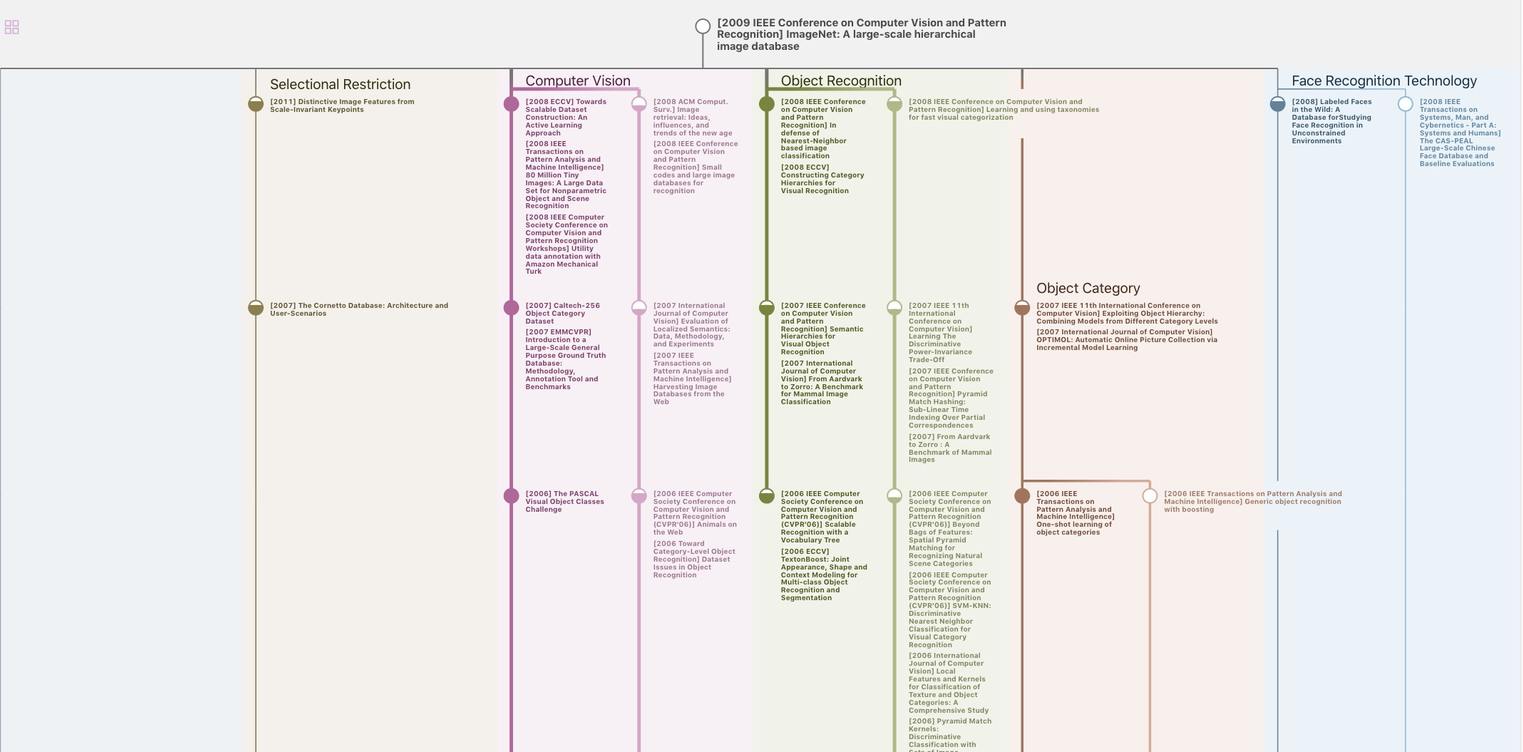
生成溯源树,研究论文发展脉络
Chat Paper
正在生成论文摘要