On the Security of the One-and-a-Half-Class Classifier for SPAM Feature-Based Image Forensics
IEEE Trans. Inf. Forensics Secur.(2023)
摘要
Combining multiple classifiers is a promising approach to hardening forensic detectors against adversarial evasion attacks. The key idea is that an attacker must fool all individual classifiers to evade detection. The 1.5C classifier is one of these multiple-classifier detectors that is attack-agnostic, and thus even increases the difficulty for an omniscient attacker. Recent work evaluated the 1.5C classifier with SPAM features for image manipulation detection. Despite showing promising results, their security analysis leaves several aspects unresolved. Surprisingly, the results reveal that fooling only one component is often sufficient to evade detection. Additionally, the authors evaluate classifier robustness with only a black-box attack because, currently, there is no white-box attack against SPAM feature-based classifiers. This paper addresses these shortcomings and complements the previous security analysis. First, we develop a novel white-box attack against SPAM feature-based detectors. The proposed attack produces adversarial images with lower distortion than the previous attack. Second, by analyzing the 1.5C classifier’s acceptance region, we identify three pitfalls that explain why the current 1.5C classifier is less robust than a binary classifier in some settings. Third, we illustrate how to mitigate these pitfalls with a simple axis-aligned split classifier. Our experimental evaluation demonstrates the increased robustness of the proposed detector for SPAM feature-based image manipulation detection.
更多查看译文
关键词
Image forensics,counter-forensics,adversarial examples,one-and-a-half-class classifier
AI 理解论文
溯源树
样例
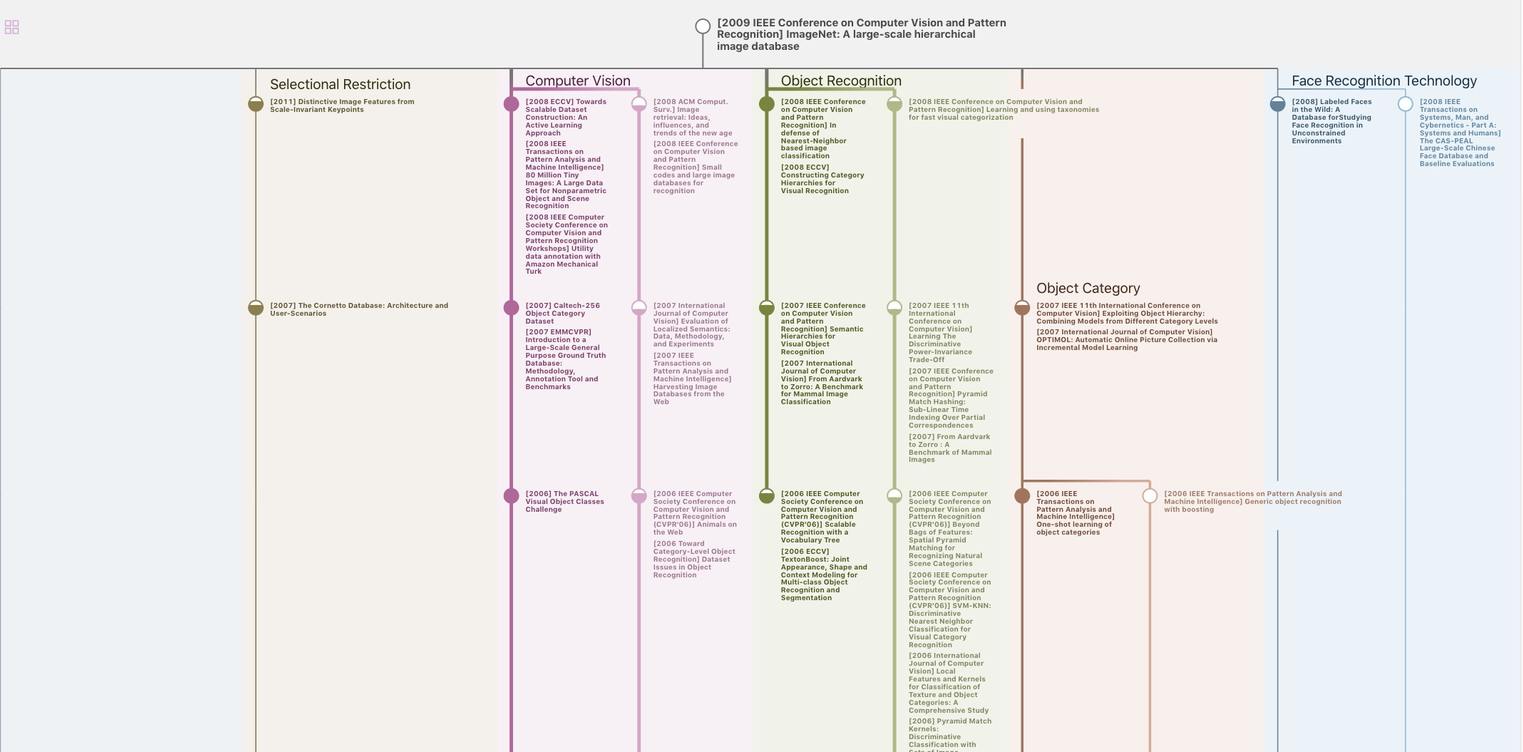
生成溯源树,研究论文发展脉络
Chat Paper
正在生成论文摘要