An Automatic Generation of Heterogeneous Knowledge Graph for Global Disease Support: A Demonstration of a Cancer Use Case.
Big Data Cogn. Comput.(2023)
摘要
Semantic data integration provides the ability to interrelate and analyze information from multiple heterogeneous resources. With the growing complexity of medical ontologies and the big data generated from different resources, there is a need for integrating medical ontologies and finding relationships between distinct concepts from different ontologies where these concepts have logical medical relationships. Standardized Medical Ontologies are explicit specifications of shared conceptualization, which provide predefined medical vocabulary that serves as a stable conceptual interface to medical data sources. Intelligent Healthcare systems such as disease prediction systems require a reliable knowledge base that is based on Standardized medical ontologies. Knowledge graphs have emerged as a powerful dynamic representation of a knowledge base. In this paper, a framework is proposed for automatic knowledge graph generation integrating two medical standardized ontologies- Human Disease Ontology (DO), and Symptom Ontology (SYMP) using a medical online website and encyclopedia. The framework and methodologies adopted for automatically generating this knowledge graph fully integrated the two standardized ontologies. The graph is dynamic, scalable, easily reproducible, reliable, and practically efficient. A subgraph for cancer terms is also extracted and studied for modeling and representing cancer diseases, their symptoms, prevention, and risk factors.
更多查看译文
关键词
heterogeneous knowledge graph,global disease support,cancer
AI 理解论文
溯源树
样例
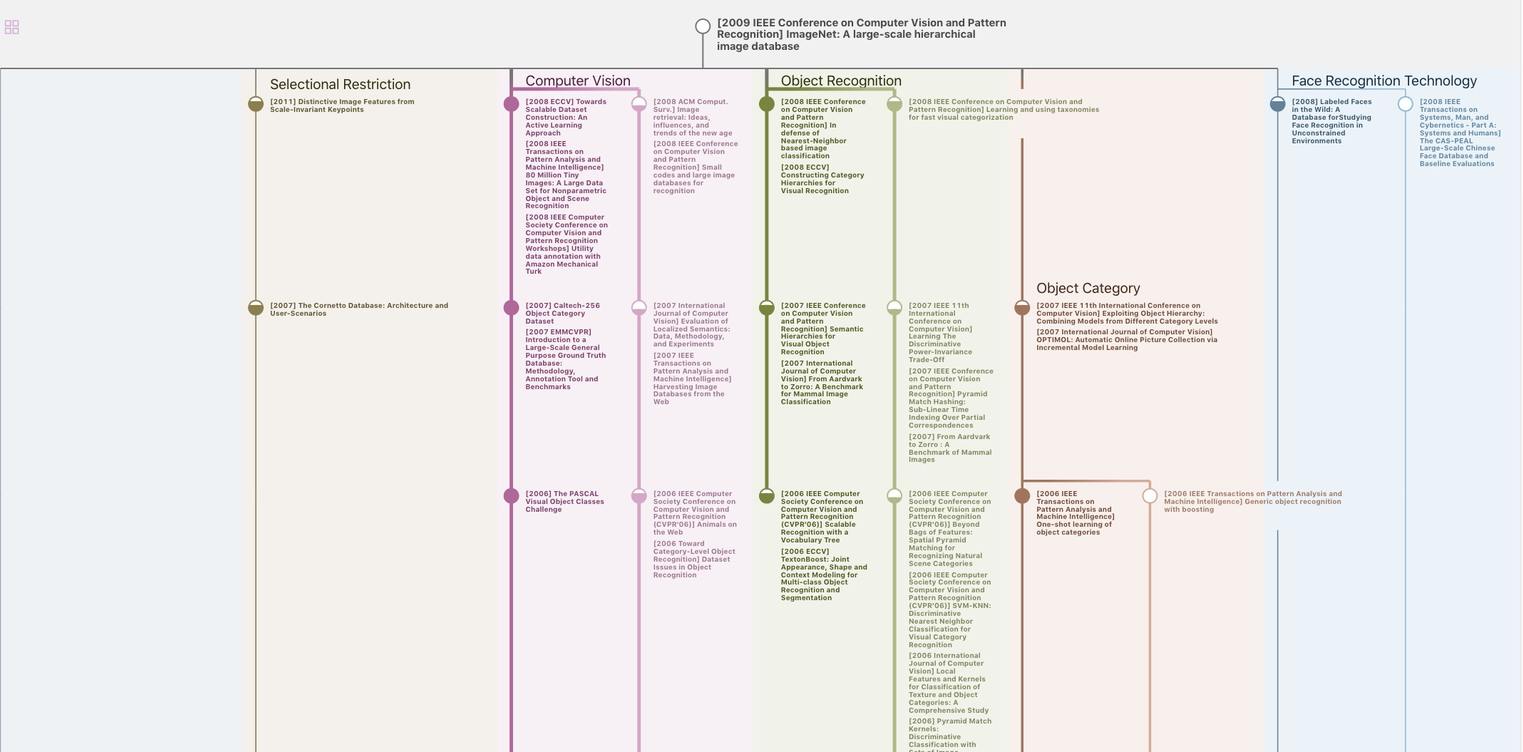
生成溯源树,研究论文发展脉络
Chat Paper
正在生成论文摘要