NVAutoNet: Fast and Accurate 360° 3D Visual Perception For Self Driving.
IEEE/CVF Winter Conference on Applications of Computer Vision(2024)
摘要
Achieving robust and real-time 3D perception is fundamental for autonomous vehicles. While most existing 3D perception methods prioritize detection accuracy, they often overlook critical aspects such as computational efficiency, onboard chip deployment friendliness, resilience to sensor mounting deviations, and adaptability to various vehicle types. To address these challenges, we present NVAutoNet: a specialized Bird’s-Eye-View (BEV) perception network tailored explicitly for automated vehicles. NVAutoNet takes synchronized camera images as input and predicts 3D signals like obstacles, freespaces, and parking spaces. The core of NVAutoNet’s architecture (image and BEV backbones) relies on efficient convolutional networks, optimized for high performance using TensorRT. Our image-to-BEV transformation employs simple linear layers and BEV lookup tables, ensuring rapid inference speed. Trained on an extensive proprietary dataset, NVAutoNet consistently achieves elevated perception accuracy, operating remarkably at 53 frames per second on the NVIDIA DRIVE Orin SoC. Notably, NVAutoNet demonstrates resilience to sensor mounting deviations arising from diverse car models. Moreover, NVAutoNet excels in adapting to varied vehicle types, facilitated by inexpensive model fine-tuning procedures that expedite compatibility adjustments.
更多查看译文
关键词
Applications,Autonomous Driving,Algorithms,3D computer vision,Algorithms,Image recognition and understanding
AI 理解论文
溯源树
样例
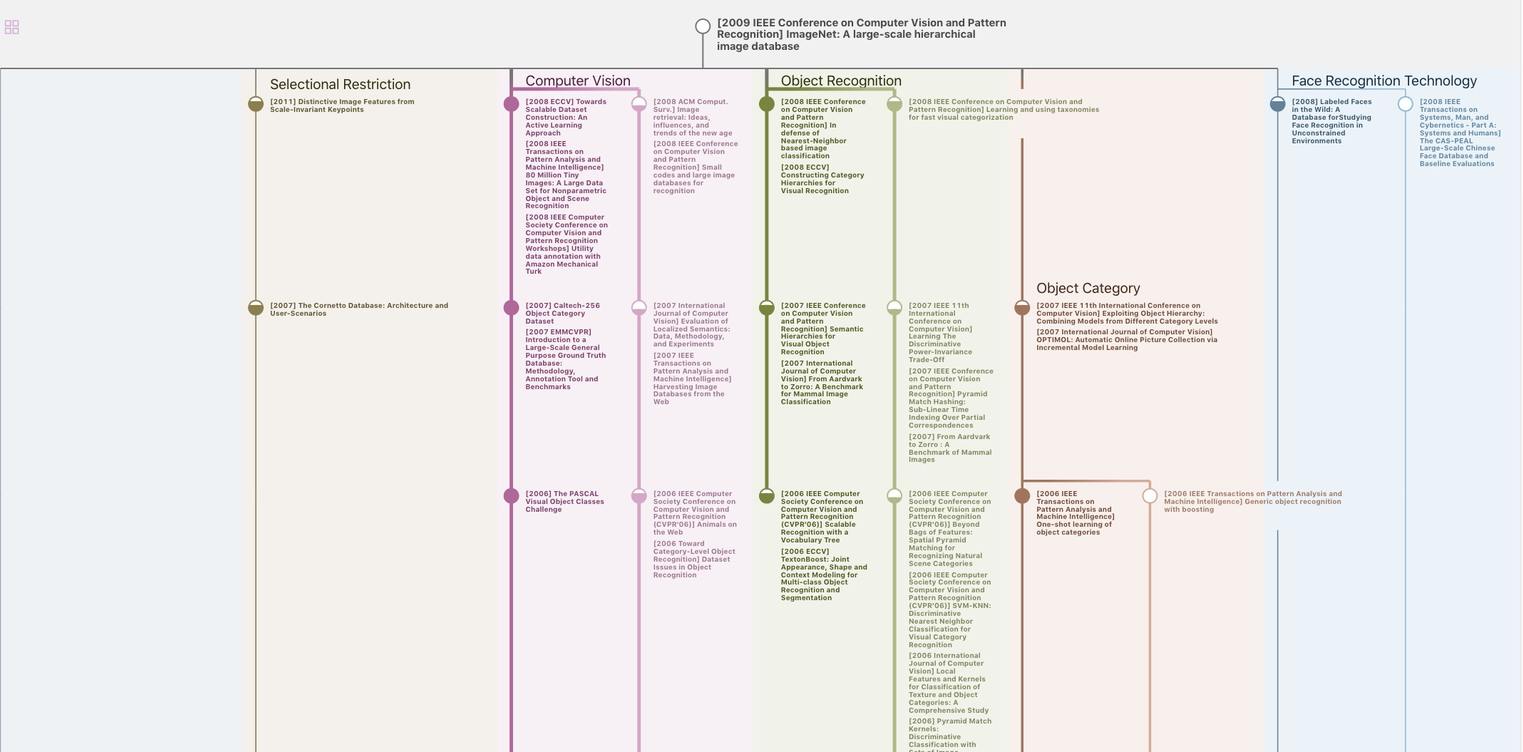
生成溯源树,研究论文发展脉络
Chat Paper
正在生成论文摘要