Adversarial Tradeoffs in Linear Inverse Problems and Robust State Estimation.
CoRR(2021)
摘要
Adversarially robust training has been shown to reduce the susceptibility of learned models to targeted input data perturbations. However, it has also been observed that such adversarially robust models suffer a degradation in accuracy when applied to unperturbed data sets, leading to a robustness-accuracy tradeoff. In this paper, we provide sharp and interpretable characterizations of such robustness-accuracy tradeoffs for linear inverse problems. In particular, we provide an algorithm to find the optimal adversarial perturbation given data, and develop tight upper and lower bounds on the adversarial loss in terms of the standard (non-adversarial) loss and the spectral properties of the resulting estimator. Further, motivated by the use of adversarial training in reinforcement learning, we define and analyze the \emph{adversarially robust Kalman Filtering problem.} We apply a refined version of our general theory to this problem, and provide the first characterization of robustness-accuracy tradeoffs in a setting where the data is generated by a dynamical system. In doing so, we show a natural connection between a filter's robustness to adversarial perturbation and underlying control theoretic properties of the system being observed, namely the spectral properties of its observability gramian.
更多查看译文
关键词
robust state
AI 理解论文
溯源树
样例
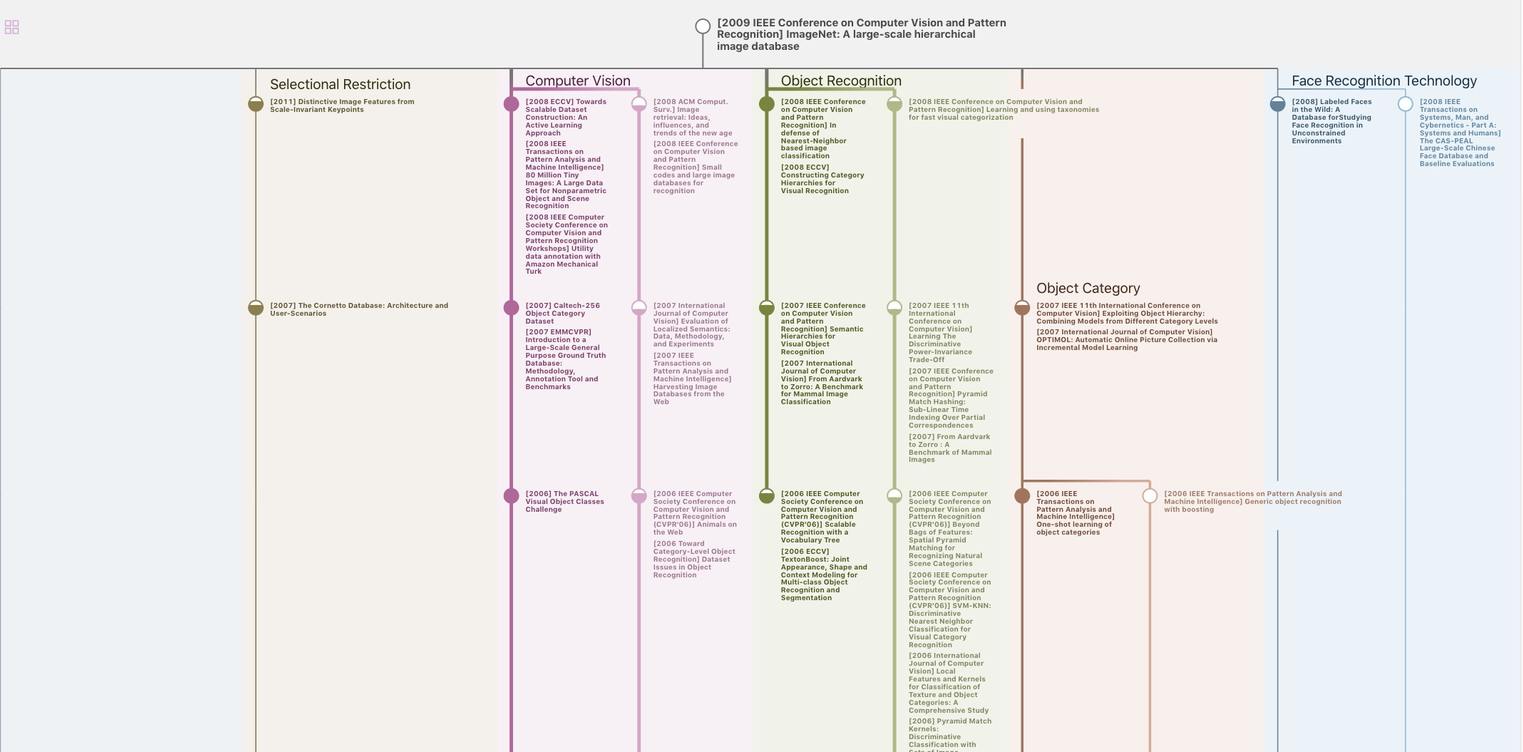
生成溯源树,研究论文发展脉络
Chat Paper
正在生成论文摘要