Learning Gaussian Mixtures with Generalised Linear Models: Precise Asymptotics in High-dimensions.
CoRR(2021)
摘要
Generalised linear models for multi-class classification problems are one of the fundamental building blocks of modern machine learning tasks. In this manuscript, we characterise the learning of a mixture of K Gaussians with generic means and covariances via empirical risk minimisation (ERM) with any convex loss and regularisation. In particular, we prove exact asymptotics characterising the ERM estimator in high-dimensions, extending several previous results about Gaussian mixture classification in the literature. We exemplify our result in two tasks of interest in statistical learning: a) classification for a mixture with sparse means, where we study the efficiency of l(1) penalty with respect to l(2); b) max-margin multi-class classification, where we characterise the phase transition on the existence of the multi-class logistic maximum likelihood estimator for K > 2. Finally, we discuss how our theory can be applied beyond the scope of synthetic data, showing that in different cases Gaussian mixtures capture closely the learning curve of classification tasks in real data sets.
更多查看译文
关键词
gaussian mixtures,generalised linear models,linear models,high-dimensions
AI 理解论文
溯源树
样例
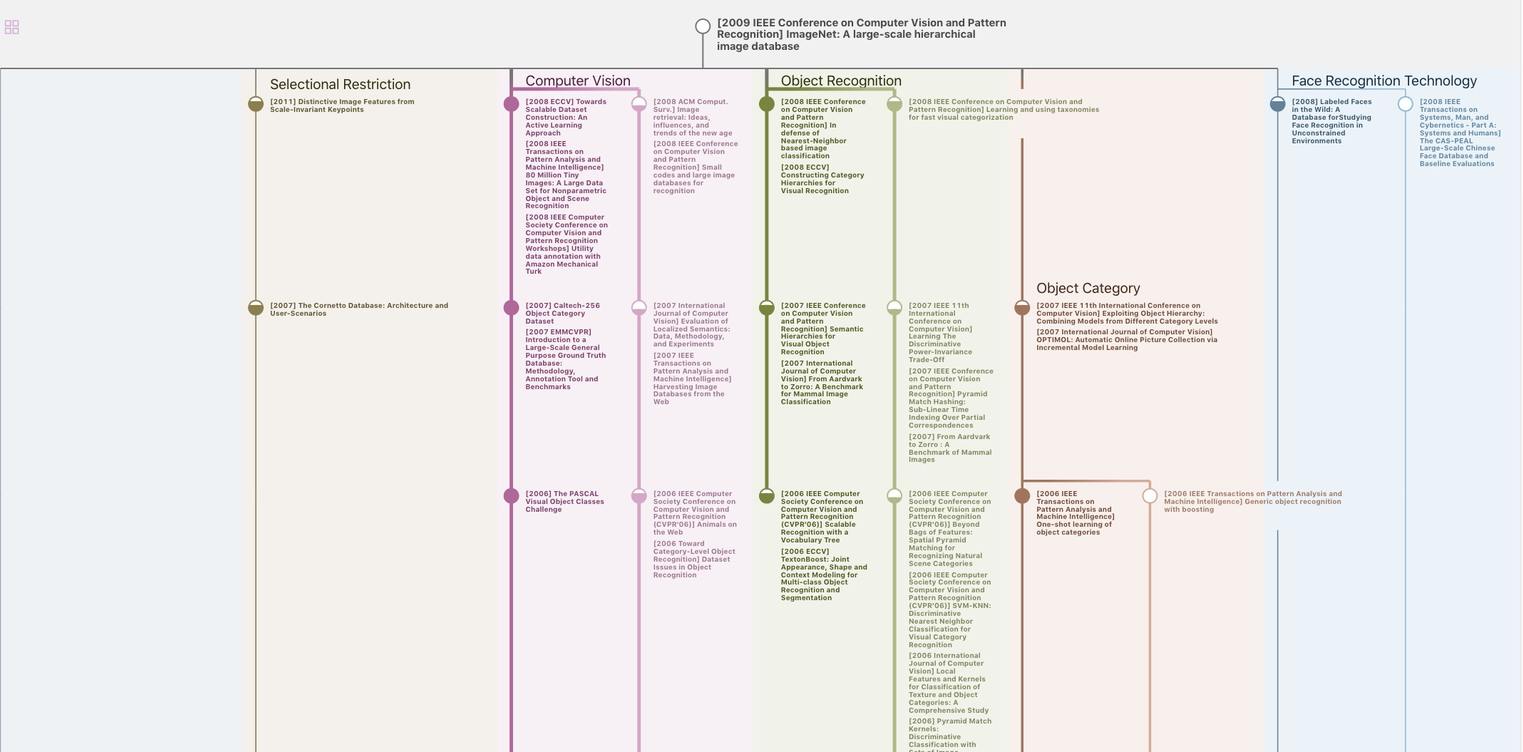
生成溯源树,研究论文发展脉络
Chat Paper
正在生成论文摘要