Multi-scale self-attention mixup for graph classification.
Pattern Recognit. Lett.(2023)
摘要
Data augmentation can effectively improve the generalization performance of neural networks. However, data augmentation for the graph domain is challenging due to the fact of irregular nature of the special non-Euclidean structure. In this paper, we propose a novel graph data augmentation solution, Multi-Scale Self-Attention Mixup (MSSA-Mixup), which extends the training distribution by interpolating multi-scale graph representation with self-attention. The MSSA-Mixup improves the generalization ability of graph neural networks (GNNs) effectively. Extensive experiments illustrate that the proposed method yields consistent and robust performance boost across graph classification tasks on the frequently-used bench-mark datasets.(c) 2023 Elsevier B.V. All rights reserved.
更多查看译文
关键词
Graph convolutional network,Self -Attention,Mixup,Graph classification
AI 理解论文
溯源树
样例
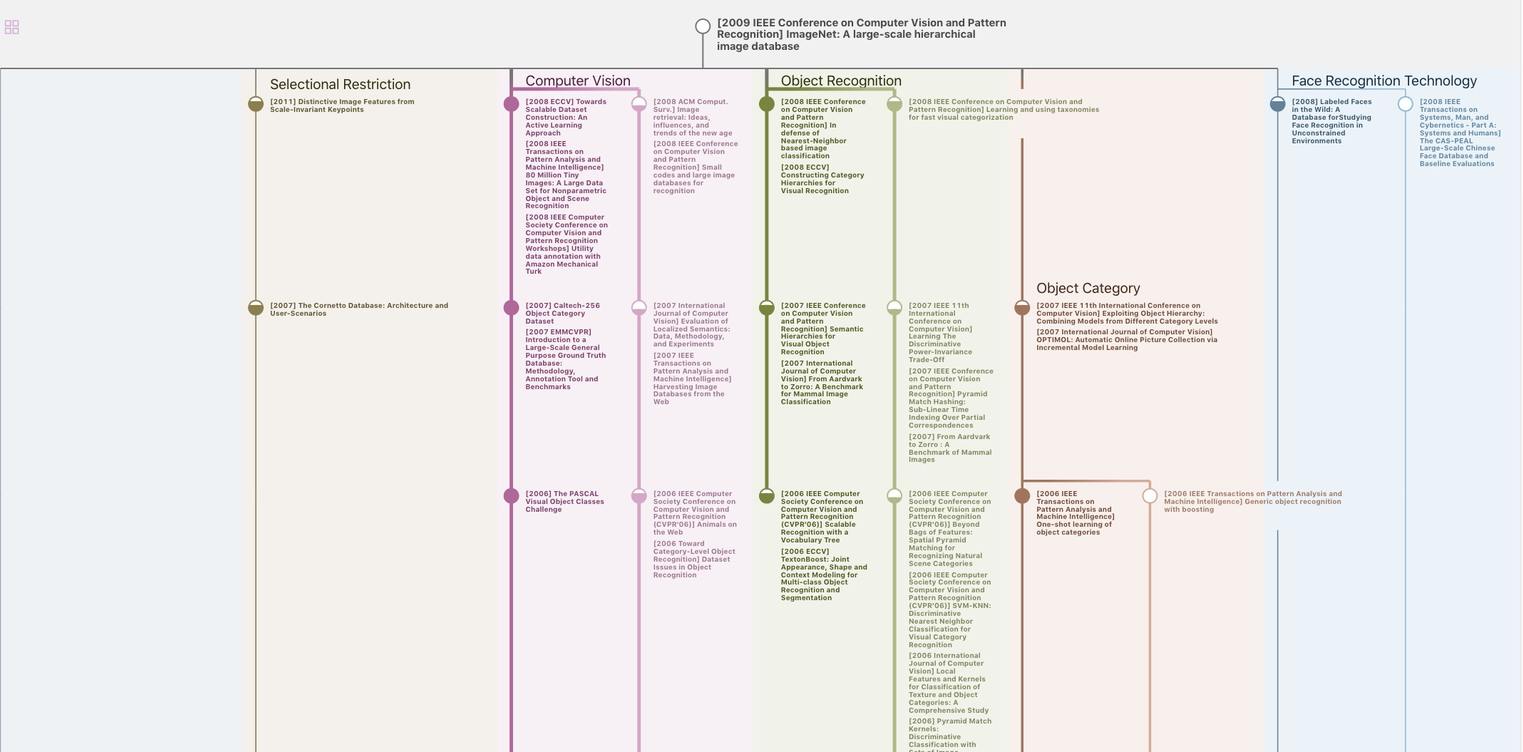
生成溯源树,研究论文发展脉络
Chat Paper
正在生成论文摘要