A Comprehensive Study of Clustering-Based Techniques for Detecting Abnormal Vessel Behavior.
Remote. Sens.(2023)
Abstract
Abnormal behavior detection is currently receiving much attention because of the availability of marine equipment and data allowing maritime agents to track vessels. One of the most popular tools for developing an efficient anomaly detection system is the Automatic Identification System (AIS). The aim of this paper is to explore the performance of existing well-known clustering methods for detecting the two most dangerous abnormal behaviors based on the AIS. The methods include K-means, Density-Based Spatial Clustering of Applications with Noise (DBSCAN), Affinity Propagation (AP), and the Gaussian Mixtures Model (GMM). In order to evaluate the performance of the clustering methods, we also used the AIS data of vessels, which were collected through the Finnish transport agency from the whole Baltic Sea for three months. Although most existing studies focus on ocean route recognition, deviations from regulated ocean routes, or irregular speed, we focused on dark ships or those sets of vessels that turn off the AIS to perform illegal activities and spiral vessel movements. The experimental results demonstrate that the K-means clustering method can effectively detect dark ships and spiral vessel movements, which are the most threatening events for maritime safety.
MoreTranslated text
Key words
maritime surveillance system,clustering methods,abnormal vessel behavior,machine learning,situational awareness,AIS data
AI Read Science
Must-Reading Tree
Example
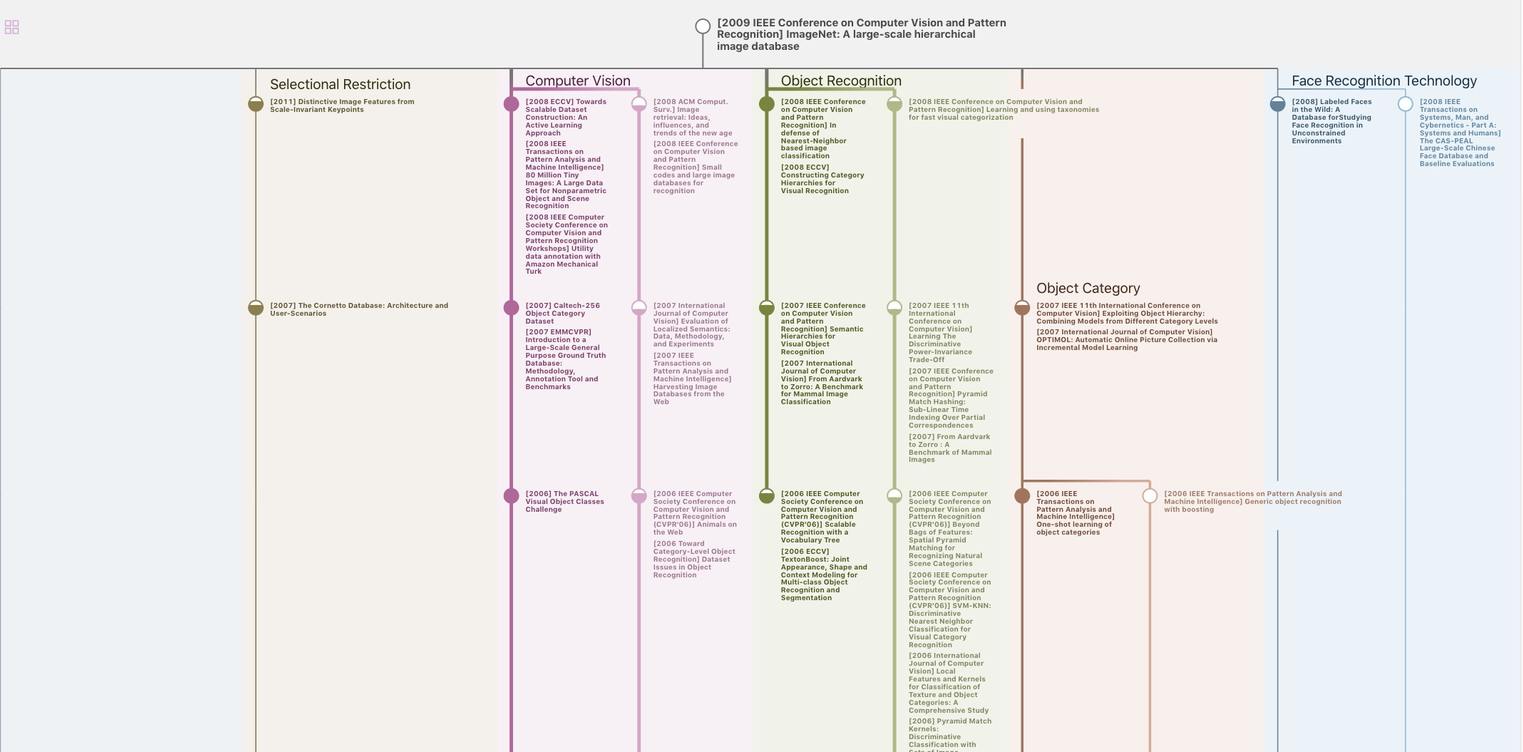
Generate MRT to find the research sequence of this paper
Chat Paper
Summary is being generated by the instructions you defined