Deep learning based video surveillance for predicting vehicle density in real time scenario
J. Ambient Intell. Humaniz. Comput.(2023)
摘要
Video surveillance systems are often used for traffic monitoring and to characterize traffic load. However, most of the surveillance videos are low frame rated and extracting the right motion feature from them is a challenging task. Deep learning is a growing technology that extracts each object’s feature without human intervention. This paper proposes two different Convolutional Neural Networks (CNN) named Multi Scale Convolutional Neural networks (MSCNN) and Single Scale Convolutional Neural networks (SSCNN) to extract the features and prediction of traffic density. The experimental evaluation of the proposed models was carried out on the UCSD traffic dataset. Our method exploits the benefits of the redundant nature of traffic scenes that are captured from a top-down perspective showing vehicles on a road encircled by background objects. Though a variety of objects is present in each frame, our proposed method attempts to acquire information only about specific object features which helps to predict traffic density better in real-time scenarios. Based on the extracted features, each traffic scene is categorized into three different classes low, medium, and high. Also, the performance of the proposed architecture exhibits 12% more accuracy compared with the existing deep neural networks. Furthermore, in comparison with the MAE score, the proposed system is able to reduce the error from 1 to 0.3 than the existing models. In addition, the training and testing phases of the proposed approach consume considerably less time and optimized usage of CPU resources.
更多查看译文
关键词
vehicle density,video surveillance,deep learning
AI 理解论文
溯源树
样例
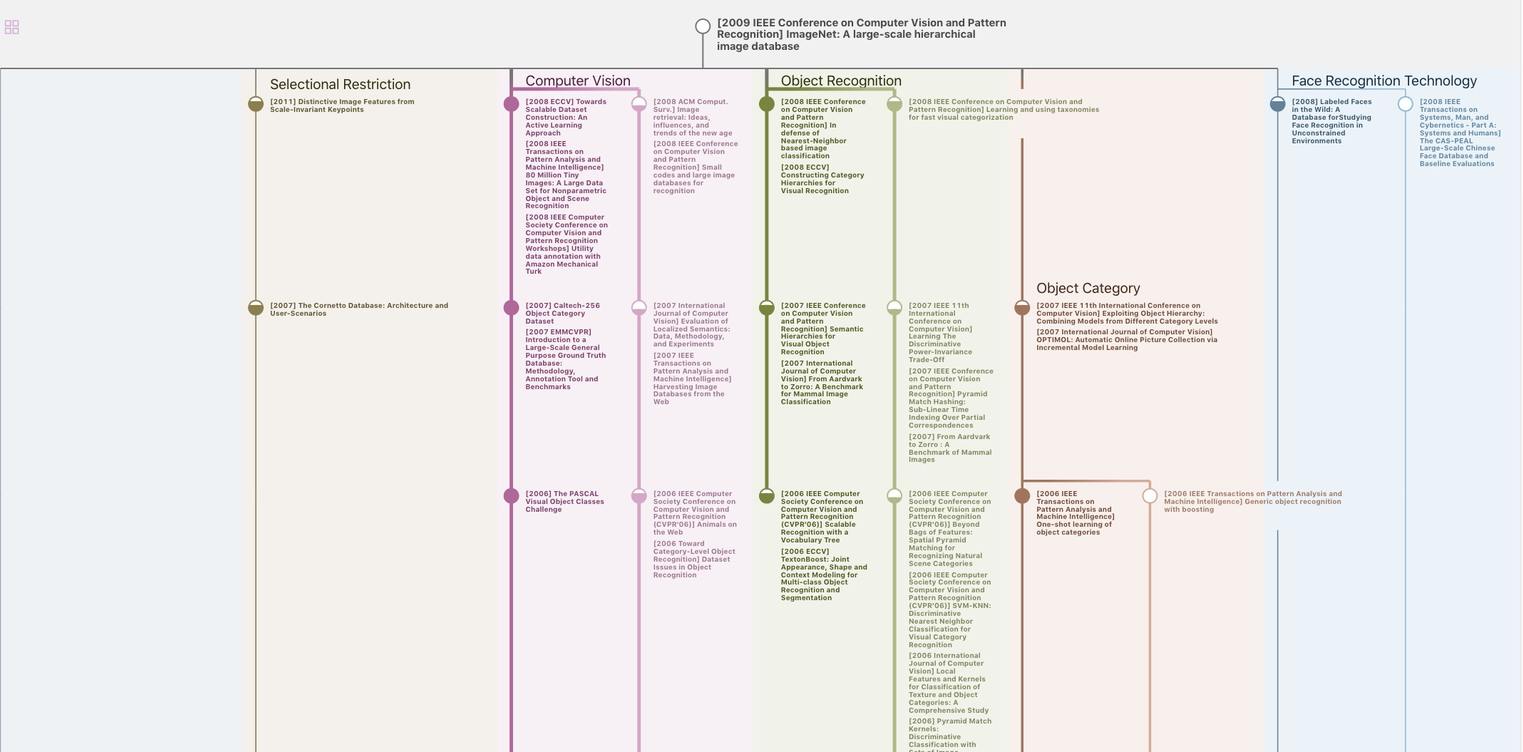
生成溯源树,研究论文发展脉络
Chat Paper
正在生成论文摘要