QE-DAL: A quantum image feature extraction with dense distribution-aware learning framework for object counting and localization.
Appl. Soft Comput.(2023)
摘要
Object counting and localization (OCL) was an essential problem in intelligent transportation fields. The convolutional neural network (CNN)-based models transformed the OCL problems into a regression task. However, the abundant semantic information of the crowd scenes may lead the CNN framework hard to extract adequate features in order to ensure good precision In this work, a Quantum Image Feature Extraction with Dense Distribution-Aware Learning (QE-DAL) framework was proposed to handle this problem. The crowd features were extracted by Quantum layers, which were extracted by encoding, quantum circuits and decode procedures based on the multi-scale architecture. For handling objects, the refined distance compensating operator was adopted to fuse the multi-scale architecture. To relieve the computation burden, a Gaussian distribution estimation mechanism was proposed to initiate and update the bounding sizes of the objects via a point-supervised manner. Finally, the joint loss function, which describes pixel classes, density maps and offset bounding boxes, was built for QE-DAL. The ablation experiment results demonstrated that the effectiveness of the quantum feature extraction architecture and the Gaussian distribution estimation mechanism of QE-DAL was validated to show superior performance than the other state-of-the-art framework. Moreover, the generalization of the QE-DAL was evidenced by the Cross-scene learning evaluation.
更多查看译文
关键词
quantum image feature extraction,object counting,qe-dal,distribution-aware
AI 理解论文
溯源树
样例
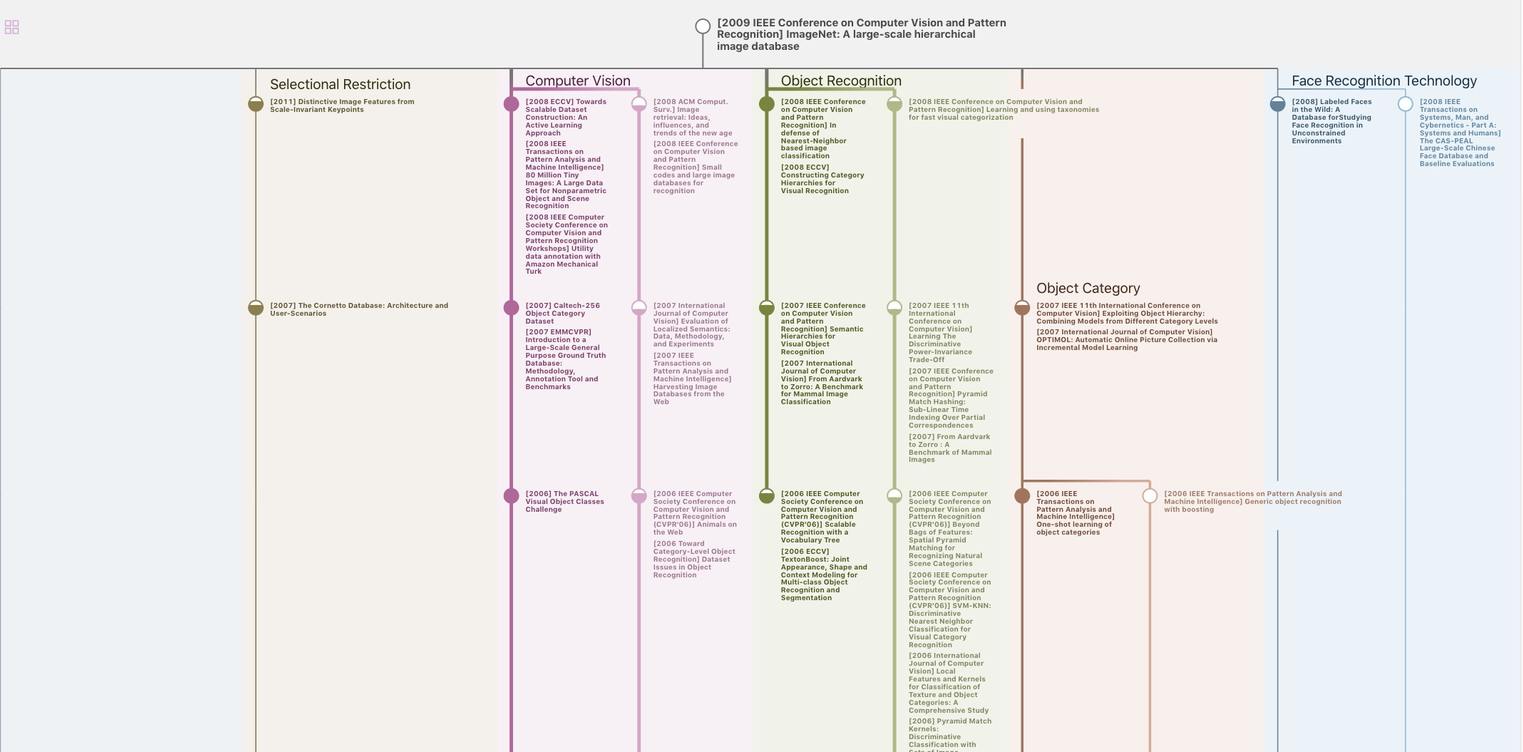
生成溯源树,研究论文发展脉络
Chat Paper
正在生成论文摘要