Deep Transferable Intelligence for Spatial Variability Characterization and Data-Efficient Learning in Biomechanical Measurement.
IEEE Trans. Instrum. Meas.(2023)
摘要
Biomechanical measurement is of promising value for rehabilitation, assisted living, and lifestyle management applications. Nevertheless, the understanding is still limited on the spatial variability of biomechanical dynamics that is essential for optimal motion sensor configuration. Besides, training physical activity detectors is usually data-heavy and time-consuming. Targeting these two challenges, in this study, we propose a novel deep transfer intelligence framework, which leverages deep learning to characterize the spatial variability of different motion sensors on diverse body locations, and further leverages intersubject transfer learning to maximize data efficiency in challenging scarce data learning. More specifically, to characterize the spatial variability, we propose deep convolutional neural networks (CNNs) to investigate the capabilities of both different sensor locations and channels on physical activity measurement. The characterization determines both optimal sensor configuration and optimal channel configuration. Further, we propose a transfer learning approach to mine intersubject similarity and then share learned knowledge among subjects, thereby minimizing the training effort and maximizing the data efficiency in the wearable scarce data learning scenario. Our evaluation experiments have determined the optimal sensor location from seven options as thigh, and the optimal sensor and channel configuration from 42 options as thigh-accelerometer-axis -Y. Our experiments have further demonstrated that, with transfer learning under the optimal sensor and channel configuration, only 10% of data from the target subject for model fine-tuning can yield a physical activity detection (PAD) accuracy of up to 91.6%, with a performance boosting of 9% compared with direct learning without transfer learning. Therefore, the deep transferable learning framework will greatly advance spatial variability characterization for optimal sensor and channel configuration and efficient scarce data learning in biomedical measurement.
更多查看译文
关键词
biomechanical measurement,deep transferable intelligence,spatial variability characterization,data-efficient
AI 理解论文
溯源树
样例
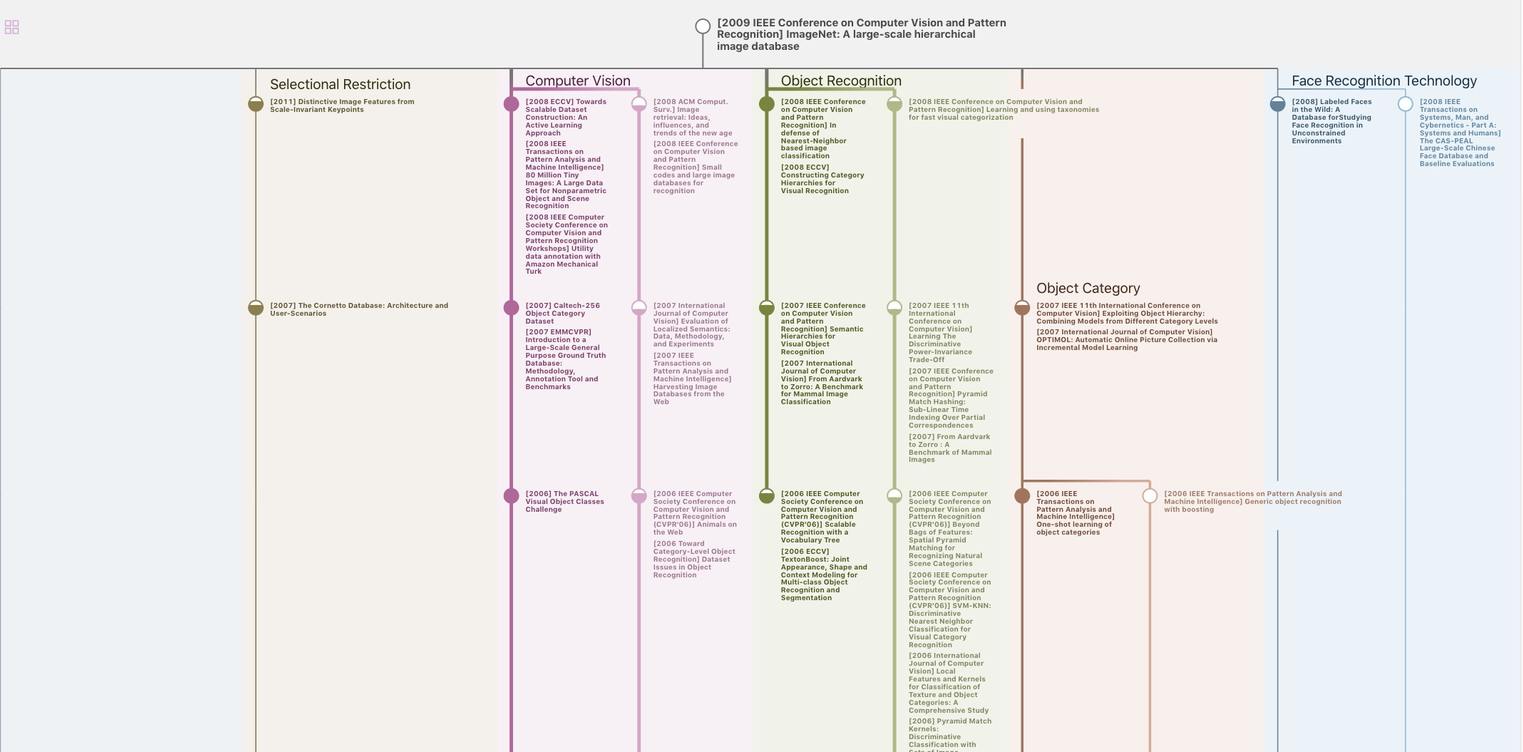
生成溯源树,研究论文发展脉络
Chat Paper
正在生成论文摘要