ItoV: Efficiently Adapting Deep Learning-Based Image Watermarking to Video Watermarking
CoRR(2023)
摘要
Robust watermarking tries to conceal information within a cover imag e/video imperceptibly that is resistant to various distortions. Recently, deep learning-based approaches for image watermarking have made significant advancements in robustness and invisibility. However, few studies focused on video watermarking using deep neural networks due to the high complexity and computational costs. Our paper aims to answer this research question: Can well-designed deep learning-based image watermarking be efficiently adapted to video watermarking? Our answer is positive. First, we revisit the workflow of deep learning-based watermarking methods that leads to a critical insight: temporal information in the video may be essential for general computer vision tasks but not for specific video watermarking. Inspired by this insight, we propose a method named ITOV for efficiently adapting deep learning-based Image watermarking to Video watermarking. Specifically, ITOV merges the temporal dimension of the video with the channel dimension to enable deep neural networks to treat videos as images. We further explore the effects of different convolutional blocks in video watermarking. We find that spatial convolution is the influential primary component in video watermarking, and depthwise convolutions significantly reduce computational costs with negligible impact on performance. In addition, we propose a new frame loss to constrain that the watermark intensity in each video clip frame is consistent, significantly improving the invisibility. Extensive experiments show the superior performance of the adapted video watermarking method compared with the state-of-the-art methods on Kinetics-600 and Inter4K datasets, which demonstrates the efficacy of our method ITOV.
更多查看译文
关键词
Watermarking,Video Watermarking,Convolutional Neural Networks,Robustness,Invisibility
AI 理解论文
溯源树
样例
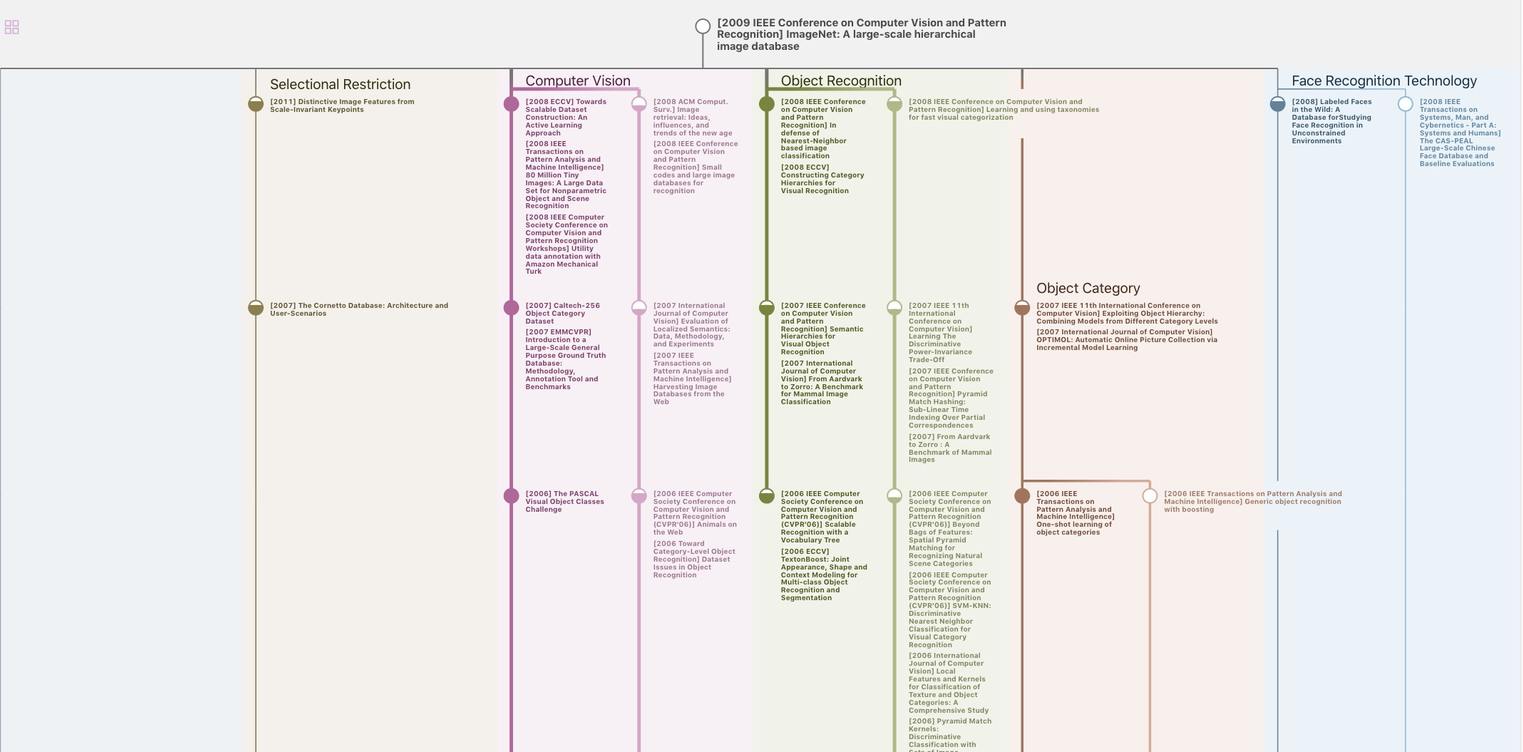
生成溯源树,研究论文发展脉络
Chat Paper
正在生成论文摘要