An Adaptive Algorithm for Learning with Unknown Distribution Drift
NeurIPS(2023)
摘要
We develop and analyze a general technique for learning with an unknown distribution drift. Given a sequence of independent observations from the last $T$ steps of a drifting distribution, our algorithm agnostically learns a family of functions with respect to the current distribution at time $T$. Unlike previous work, our technique does not require prior knowledge about the magnitude of the drift. Instead, the algorithm adapts to the sample data. Without explicitly estimating the drift, the algorithm learns a family of functions with almost the same error as a learning algorithm that knows the magnitude of the drift in advance. Furthermore, since our algorithm adapts to the data, it can guarantee a better learning error than an algorithm that relies on loose bounds on the drift.
更多查看译文
关键词
adaptive algorithm,drift,learning,distribution
AI 理解论文
溯源树
样例
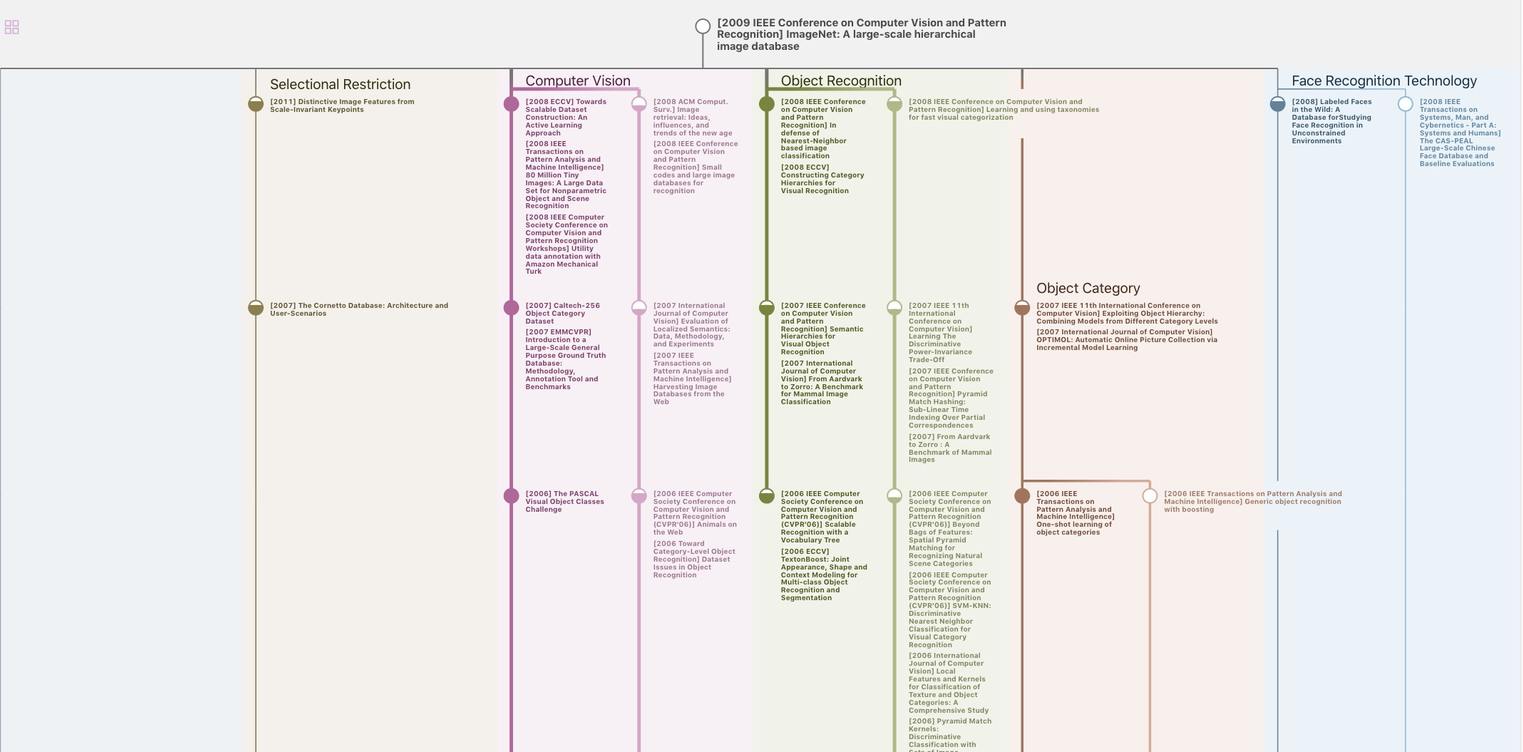
生成溯源树,研究论文发展脉络
Chat Paper
正在生成论文摘要