Commentary on explainable artificial intelligence methods: SHAP and LIME
CoRR(2023)
摘要
eXplainable artificial intelligence (XAI) methods have emerged to convert the black box of machine learning models into a more digestible form. These methods help to communicate how the model works with the aim of making machine learning models more transparent and increasing the trust of end-users into their output. SHapley Additive exPlanations (SHAP) and Local Interpretable Model Agnostic Explanation (LIME) are two widely used XAI methods particularly with tabular data. In this commentary piece, we discuss the way the explainability metrics of these two methods are generated and propose a framework for interpretation of their outputs, highlighting their weaknesses and strengths.
更多查看译文
关键词
explainable artificial intelligence methods,artificial intelligence,shap
AI 理解论文
溯源树
样例
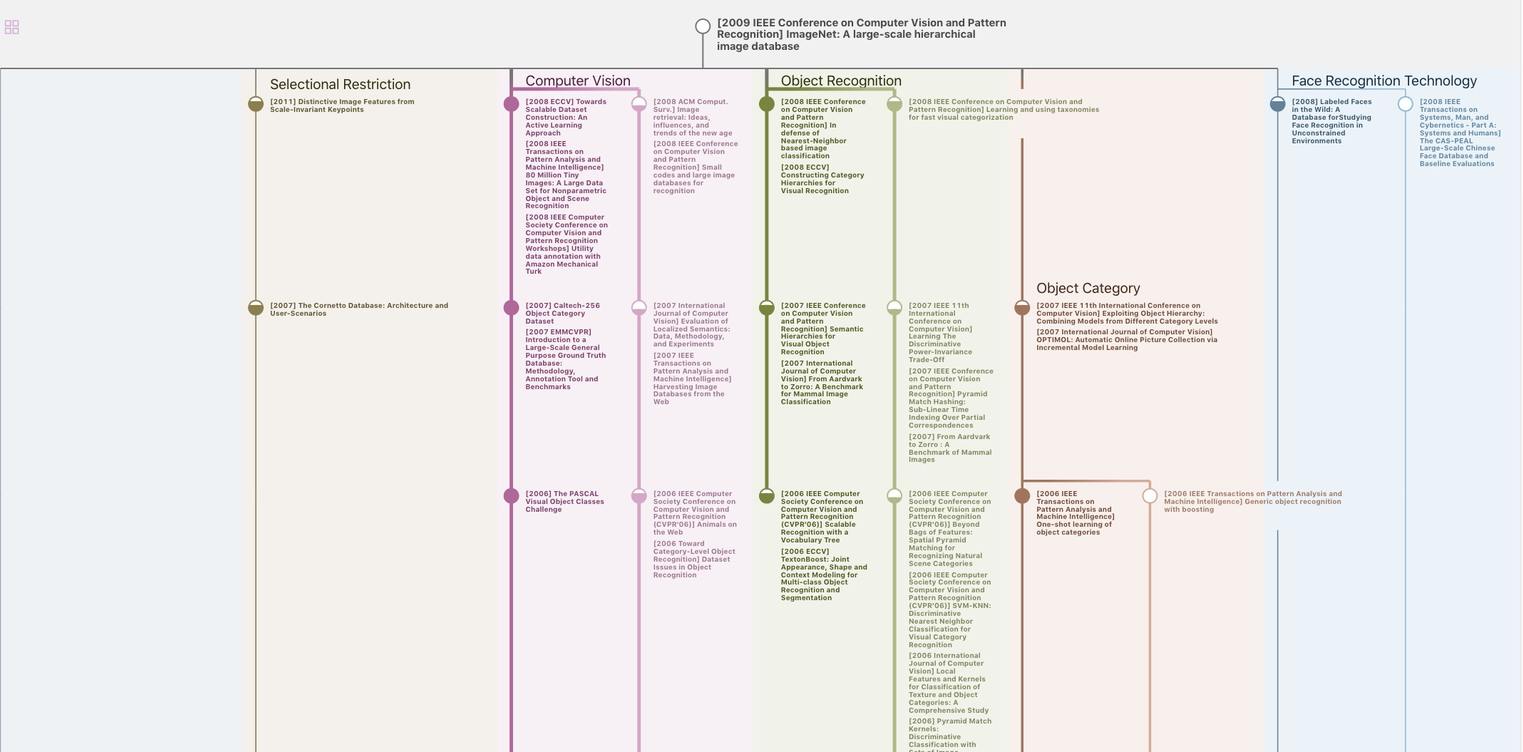
生成溯源树,研究论文发展脉络
Chat Paper
正在生成论文摘要