Accelerating Neural Self-Improvement via Bootstrapping
CoRR(2023)
摘要
Few-shot learning with sequence-processing neural networks (NNs) has recently attracted a new wave of attention in the context of large language models. In the standard N-way K-shot learning setting, an NN is explicitly optimised to learn to classify unlabelled inputs by observing a sequence of NK labelled examples. This pressures the NN to learn a learning algorithm that achieves optimal performance, given the limited number of training examples. Here we study an auxiliary loss that encourages further acceleration of few-shot learning, by applying recently proposed bootstrapped meta-learning to NN few-shot learners: we optimise the K-shot learner to match its own performance achievable by observing more than NK examples, using only NK examples. Promising results are obtained on the standard Mini-ImageNet dataset. Our code is public.
更多查看译文
关键词
self-improvement
AI 理解论文
溯源树
样例
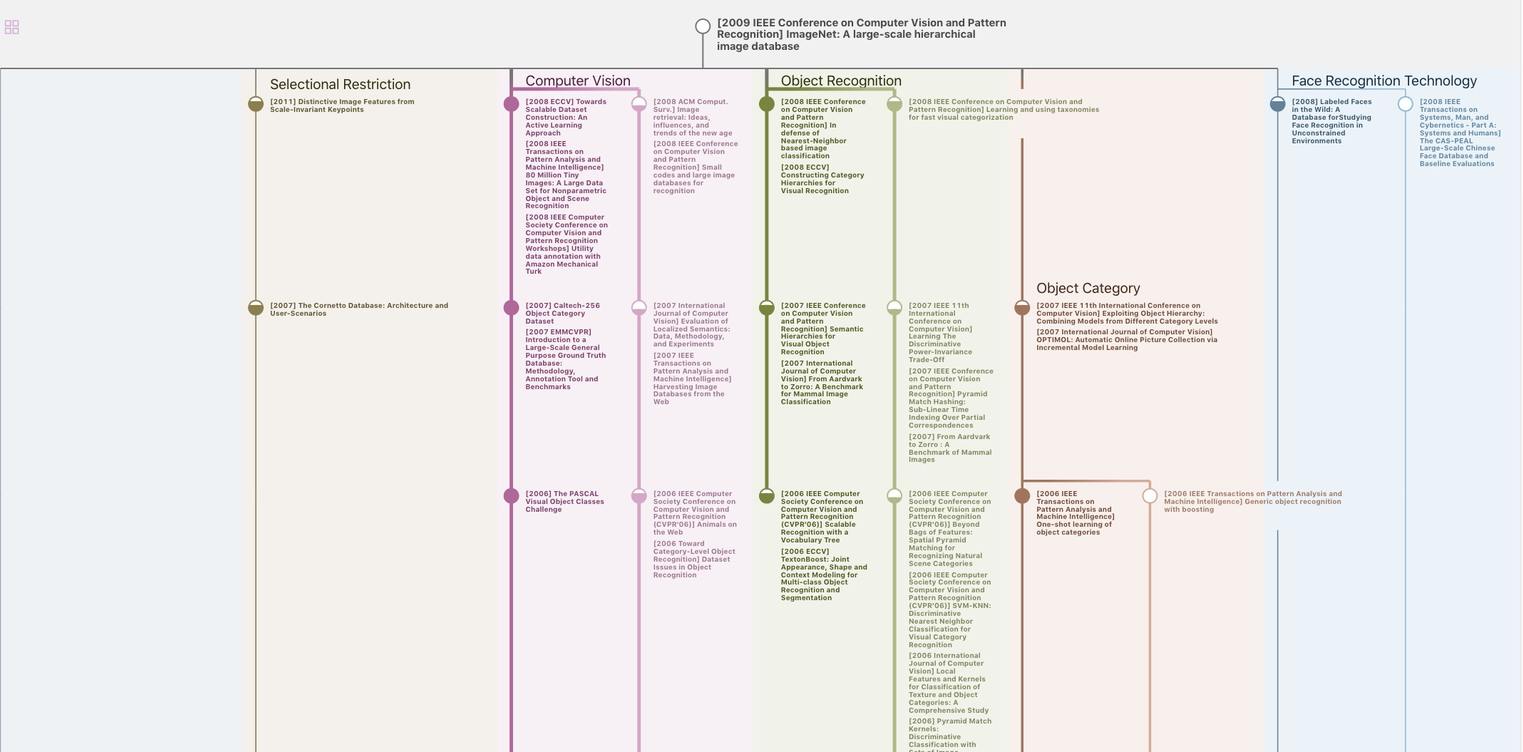
生成溯源树,研究论文发展脉络
Chat Paper
正在生成论文摘要