IndoorSim-to-OutdoorReal: Learning to Navigate Outdoors Without Any Outdoor Experience
IEEE ROBOTICS AND AUTOMATION LETTERS(2024)
摘要
We present IndoorSim-to-OutdoorReal (I2O), an end-to-end learned visual navigation approach, trained solely in simulated short-range indoor environments, and demonstrate zero-shot sim-to-real transfer to the outdoors for long-range navigation on the Spot robot. Our method uses zero real-world experience (indoor or outdoor), and requires the simulator to model no predominantly-outdoor phenomenon (sloped grounds, sidewalks, etc). The key to I2O transfer is in providing the robot with additional context of the environment (i.e. a satellite map, a rough sketch of a map by a human, etc.) to guide the robot's navigation in the real-world. The provided context-maps do not need to be accurate or complete- real-world obstacles (e.g. trees, bushes, pedestrians, etc.) are not drawn on the map, and openings are not aligned with where they are in the real-world. Crucially, these inaccurate context-maps provide a hint to the robot about a route to take to the goal. We find that our method that leverages Context-Maps is able to successfully navigate over a hundred meters in novel environments, avoiding novel obstacles on its path, to a distant goal without a single collision or human intervention. In comparison, policies without the additional context fail completely. We additionally find that the Context-Map policy is surprisingly robust to noise. In the presence of significantly inaccurate maps in simulation (corrupted with 50% noise, or entirely blank maps), the policy gracefully regresses to the behavior of a policy with no context.
更多查看译文
关键词
Robots,Robot sensing systems,Satellite navigation systems,Indoor environment,Collision avoidance,Visualization,Legged locomotion,AI-enabled robotics,vision-based navigation,reinforcement learning
AI 理解论文
溯源树
样例
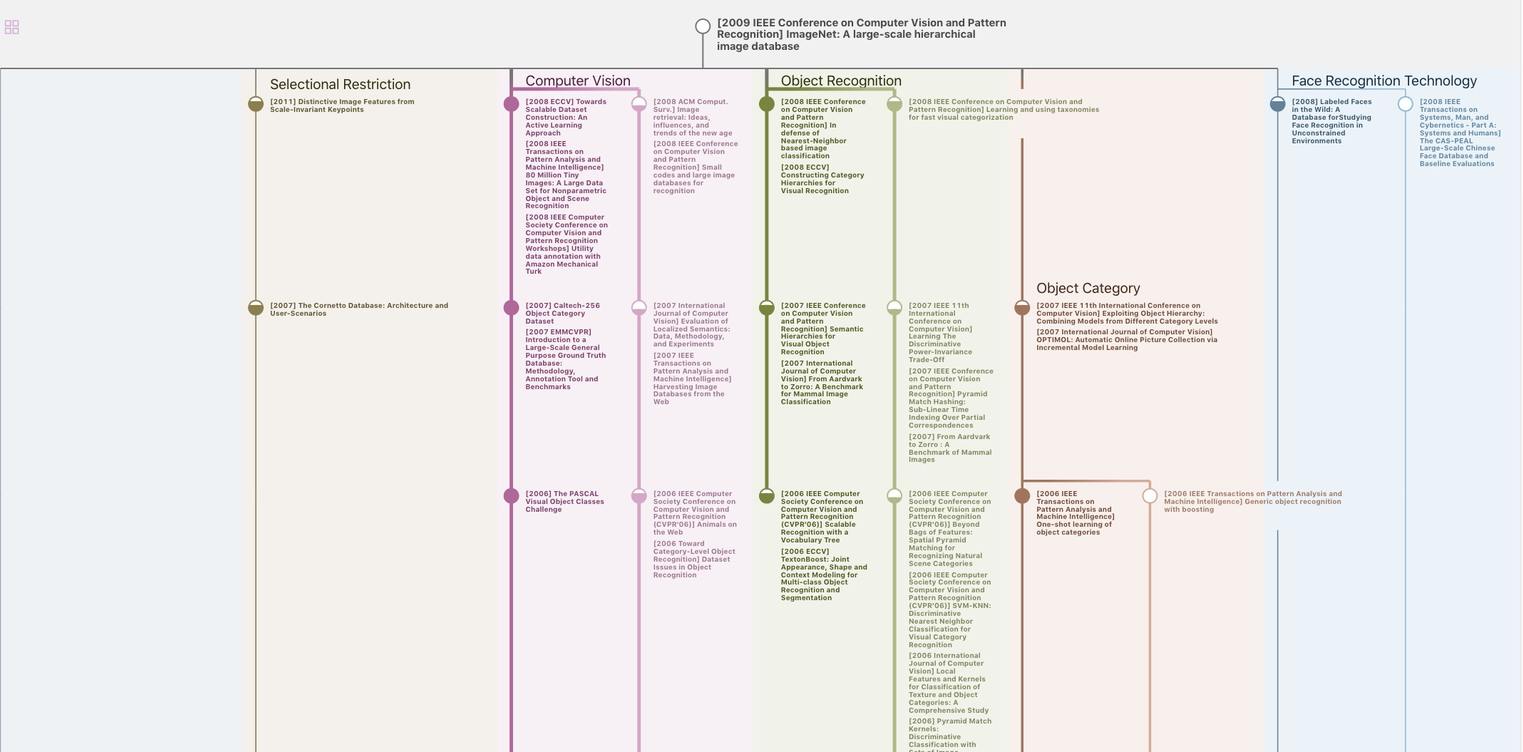
生成溯源树,研究论文发展脉络
Chat Paper
正在生成论文摘要