An optimal transport analogue of the rudin-osher-fatemi model and its corresponding multiscale theory
SIAM JOURNAL ON MATHEMATICAL ANALYSIS(2024)
摘要
In the first part of this paper we develop a theory for image restoration with a learned regularizer that is analogous to that of Meyer's geometric characterization of solutions of the classical variational method of Rudin-Osher-Fatemi (ROF). The learned regularizer we use is a Kantorovich potential for an optimal transport problem of mapping a distribution of noisy images onto clean ones, as first proposed by Lunz, \"Oktem, and Scho"\nlieb. We show that the effect of their restoration method on the distribution of the images is an explicit Euler discretization of a gradient flow on probability space, while our variational problem, dubbed Wasserstein ROF (WROF), is the corresponding implicit Euler discretization. We obtain our geometric characterization of the solution in this learned regularizer setting by first proving a much more general convex analysis theorem for variational problems having solutions characterized by projections. We then use optimal transport arguments to obtain the corresponding theorem for WROF from this general result, as well as a natural decomposition of a transport map into large scale ``features"" and small scale ``details,"" where scale refers to the magnitude of the transport distance. In the second part of the paper we leverage our theory for restoration with learned regularizers to analyze two algorithms which iterate WROF. We refer to these as iterative regularization and multiscale transport. For the former we obtain a proof of convergence to the clean data. For the latter we produce successive approximations to the target distribution that match it up to finer and finer scales. These two algorithms are in complete analogy to well-known effective methods based on ROF for iterative denoising, respectively hierarchical image decomposition. We also obtain an analogue of the Tadmor--Nezzar--Vese energy identity, which decomposes the Wasserstein 2 distance between two measures into a sum of nonnegative terms that correspond to transport costs at different scales.
更多查看译文
关键词
variational image restoration,learned regularizers,optimal transport,multiscale optimal transport
AI 理解论文
溯源树
样例
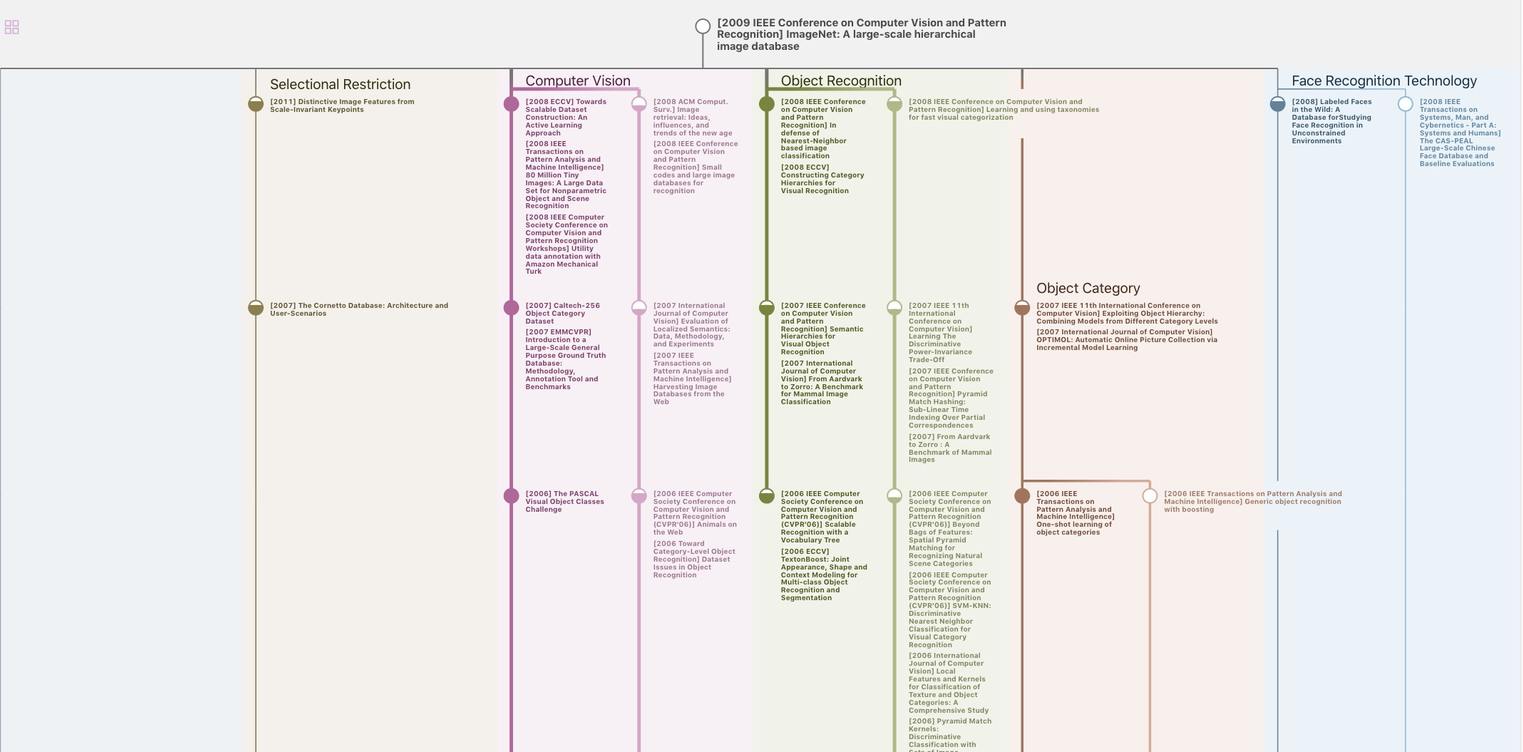
生成溯源树,研究论文发展脉络
Chat Paper
正在生成论文摘要