Joint Learning of Policy with Unknown Temporal Constraints for Safe Reinforcement Learning
CoRR(2023)
摘要
In many real-world applications, safety constraints for reinforcement learning (RL) algorithms are either unknown or not explicitly defined. We propose a framework that concurrently learns safety constraints and optimal RL policies in such environments, supported by theoretical guarantees. Our approach merges a logically-constrained RL algorithm with an evolutionary algorithm to synthesize signal temporal logic (STL) specifications. The framework is underpinned by theorems that establish the convergence of our joint learning process and provide error bounds between the discovered policy and the true optimal policy. We showcased our framework in grid-world environments, successfully identifying both acceptable safety constraints and RL policies while demonstrating the effectiveness of our theorems in practice.
更多查看译文
关键词
reinforcement learning,unknown temporal constraints,policy,safe
AI 理解论文
溯源树
样例
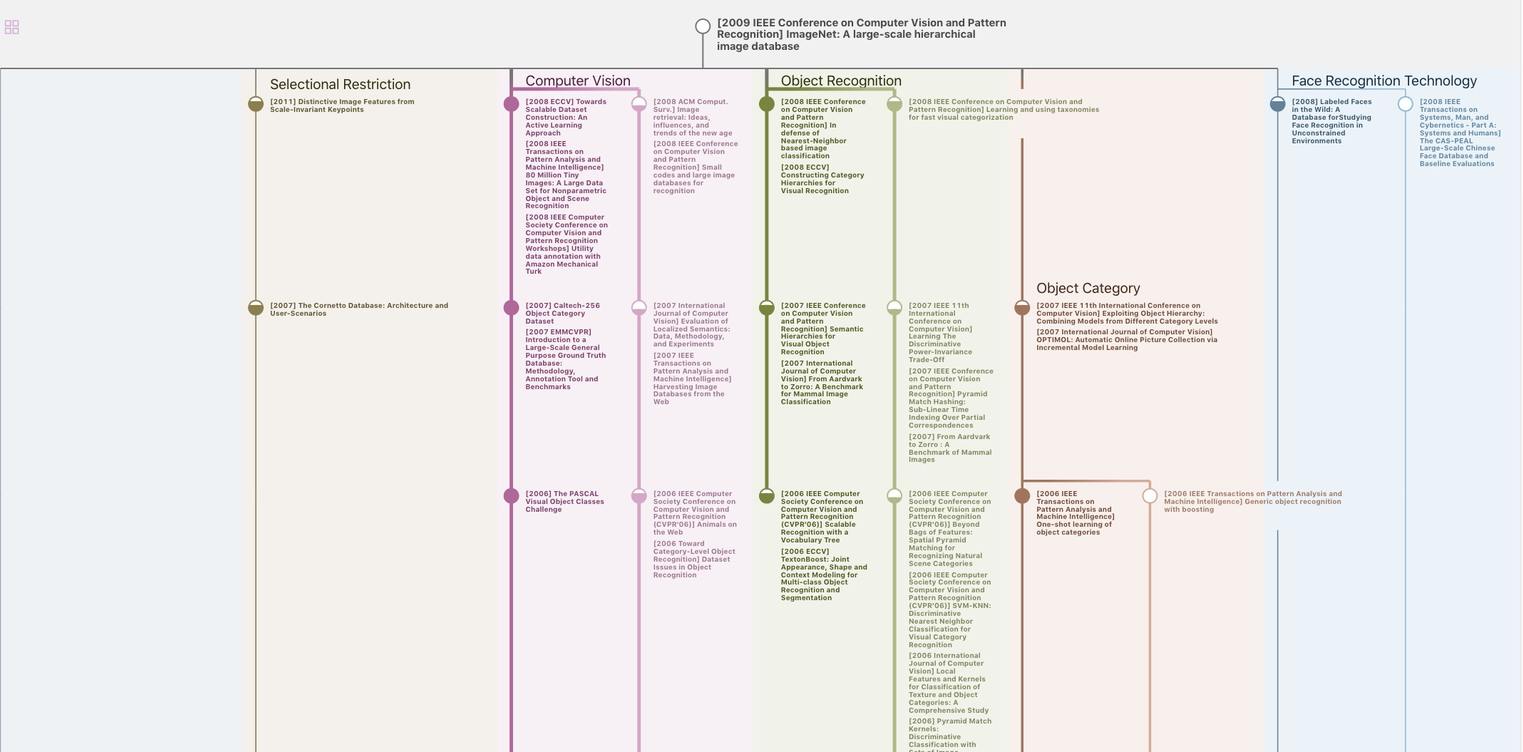
生成溯源树,研究论文发展脉络
Chat Paper
正在生成论文摘要