Sequential Markov Chain Monte Carlo for Lagrangian Data Assimilation with Applications to Unknown Data Locations
Quarterly Journal of the Royal Meteorological Society(2023)
摘要
We consider a class of high-dimensional spatial filtering problems, where the
spatial locations of observations are unknown and driven by the partially
observed hidden signal. This problem is exceptionally challenging as not only
is high-dimensional, but the model for the signal yields longer-range time
dependencies through the observation locations. Motivated by this model we
revisit a lesser-known and provably convergent computational methodology
from that uses sequential Markov Chain Monte
Carlo (MCMC) chains. We extend this methodology for data filtering problems
with unknown observation locations. We benchmark our algorithms on Linear
Gaussian state space models against competing ensemble methods and demonstrate
a significant improvement in both execution speed and accuracy. Finally, we
implement a realistic case study on a high-dimensional rotating shallow water
model (of about 10^4-10^5 dimensions) with real and synthetic data. The data
is provided by the National Oceanic and Atmospheric Administration (NOAA) and
contains observations from ocean drifters in a domain of the Atlantic Ocean
restricted to the longitude and latitude intervals [-51^∘,
-41^∘], [17^∘, 27^∘] respectively.
更多查看译文
AI 理解论文
溯源树
样例
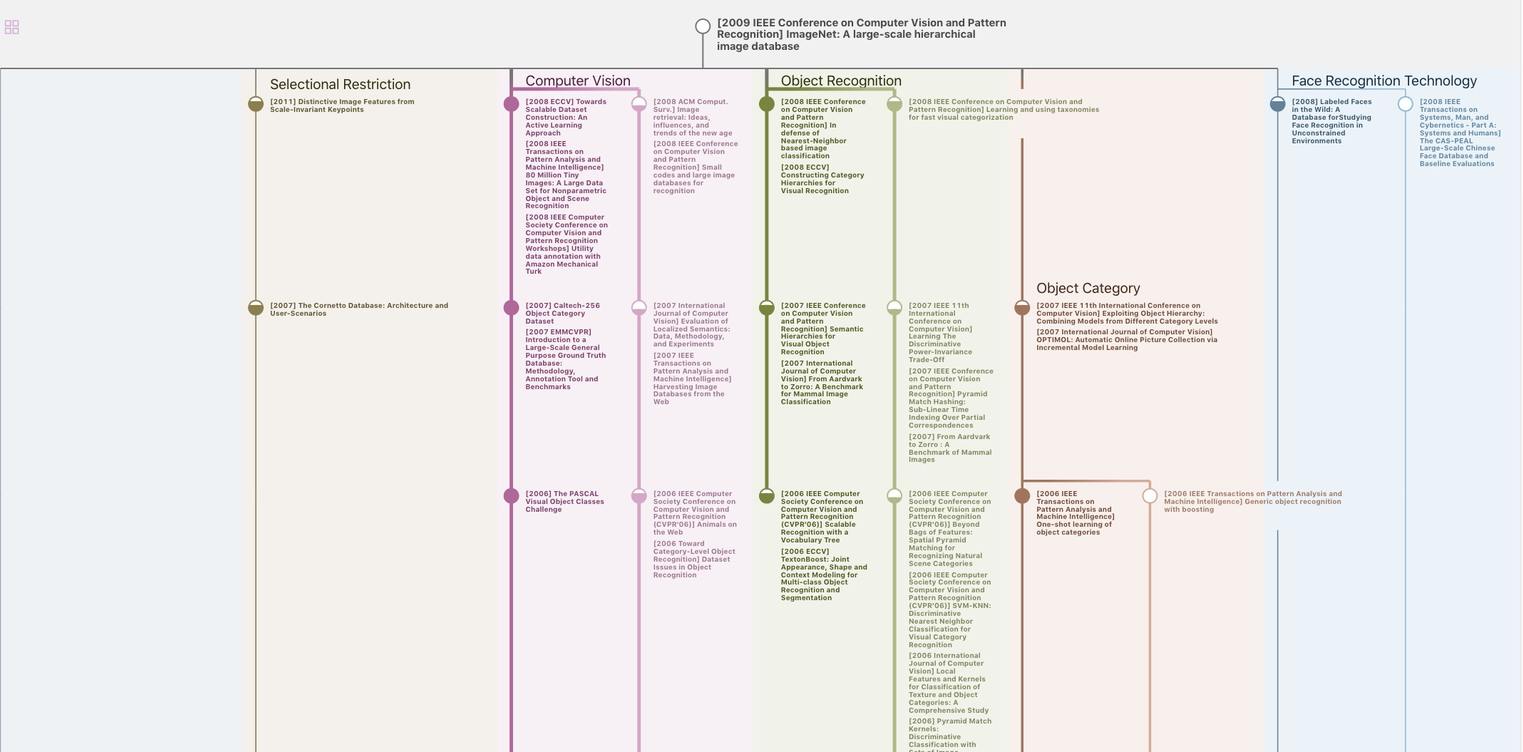
生成溯源树,研究论文发展脉络
Chat Paper
正在生成论文摘要