DD-CISENet: Dual-Domain Cross-Iteration Squeeze and Excitation Network for Accelerated MRI Reconstruction
CoRR(2023)
摘要
Magnetic resonance imaging (MRI) is widely employed for diagnostic tests in neurology. However, the utility of MRI is largely limited by its long acquisition time. Acquiring fewer k-space data in a sparse manner is a potential solution to reducing the acquisition time, but it can lead to severe aliasing reconstruction artifacts. In this paper, we present a novel Dual-Domain Cross-Iteration Squeeze and Excitation Network (DD-CISENet) for accelerated sparse MRI reconstruction. The information of k-spaces and MRI images can be iteratively fused and maintained using the Cross-Iteration Residual connection (CIR) structures. This study included 720 multi-coil brain MRI cases adopted from the open-source fastMRI Dataset. Results showed that the average reconstruction error by DD-CISENet was 2.28 $\pm$ 0.57%, which outperformed existing deep learning methods including image-domain prediction (6.03 $\pm$ 1.31, p < 0.001), k-space synthesis (6.12 $\pm$ 1.66, p < 0.001), and dual-domain feature fusion approaches (4.05 $\pm$ 0.88, p < 0.001).
更多查看译文
关键词
accelerated mri reconstruction,excitation network
AI 理解论文
溯源树
样例
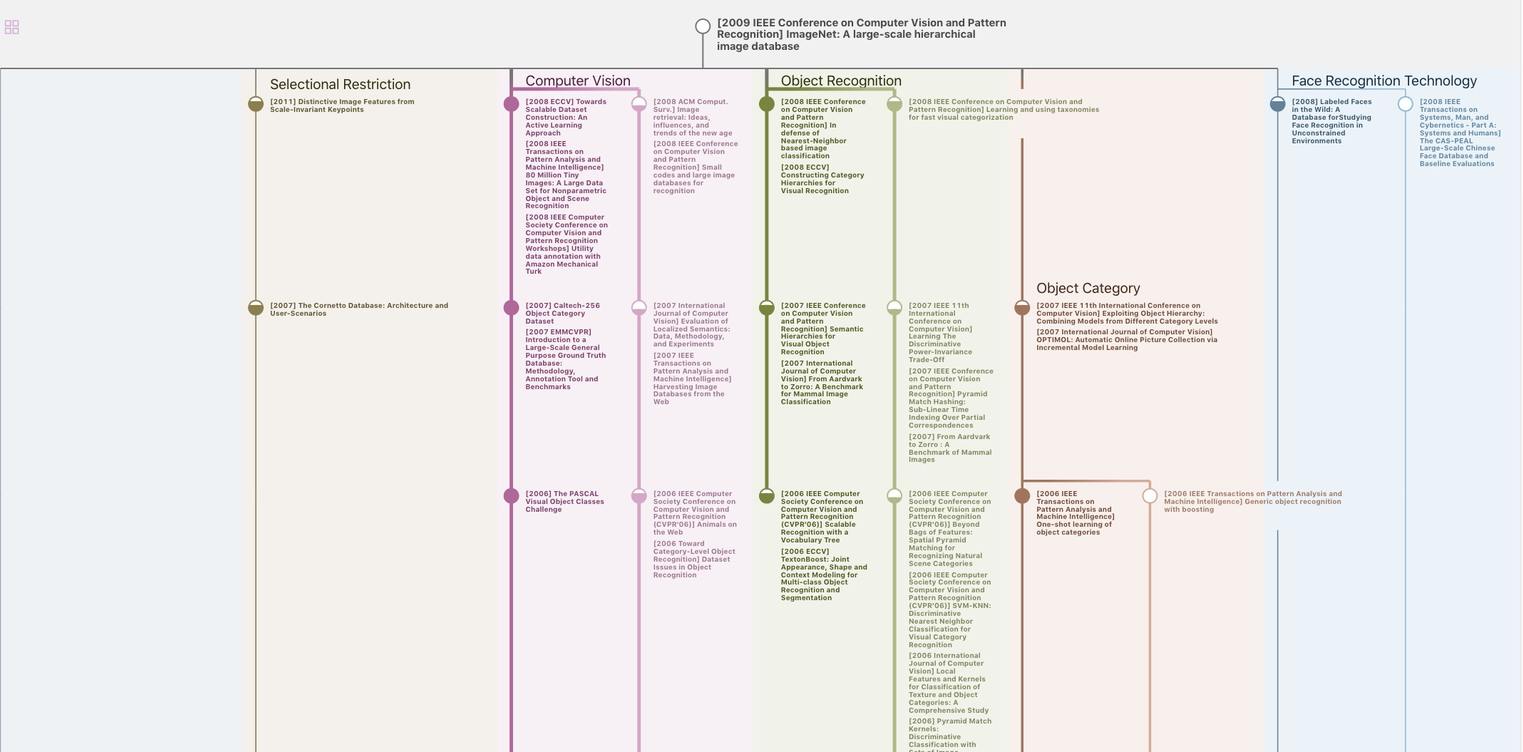
生成溯源树,研究论文发展脉络
Chat Paper
正在生成论文摘要