An EEG Channel Selection Framework for Driver Drowsiness Detection via Interpretability Guidance
2023 45TH ANNUAL INTERNATIONAL CONFERENCE OF THE IEEE ENGINEERING IN MEDICINE & BIOLOGY SOCIETY, EMBC(2023)
摘要
Drowsy driving has a crucial influence on driving safety, creating an urgent demand for driver drowsiness detection. Electroencephalogram (EEG) signal can accurately reflect the mental fatigue state and thus has been widely studied in drowsiness monitoring. However, the raw EEG data is inherently noisy and redundant, which is neglected by existing works that just use single-channel EEG data or full-head channel EEG data for model training, resulting in limited performance of driver drowsiness detection. In this paper, we are the first to propose an Interpretability-guided Channel Selection (ICS) framework for the driver drowsiness detection task. Specifically, we design a two-stage training strategy to progressively select the key contributing channels with the guidance of interpretability. We first train a teacher network in the first stage using full-head channel EEG data. Then we apply the class activation mapping (CAM) to the trained teacher model to highlight the high-contributing EEG channels and further propose a channel voting scheme to select the top N contributing EEG channels. Finally, we train a student network with the selected channels of EEG data in the second stage for driver drowsiness detection. Experiments are designed on a public dataset, and the results demonstrate that our method is highly applicable and can significantly improve the performance of cross-subject driver drowsiness detection.
更多查看译文
关键词
Driver Drowsiness Detection,Channel Selection,EEG and Interpretability
AI 理解论文
溯源树
样例
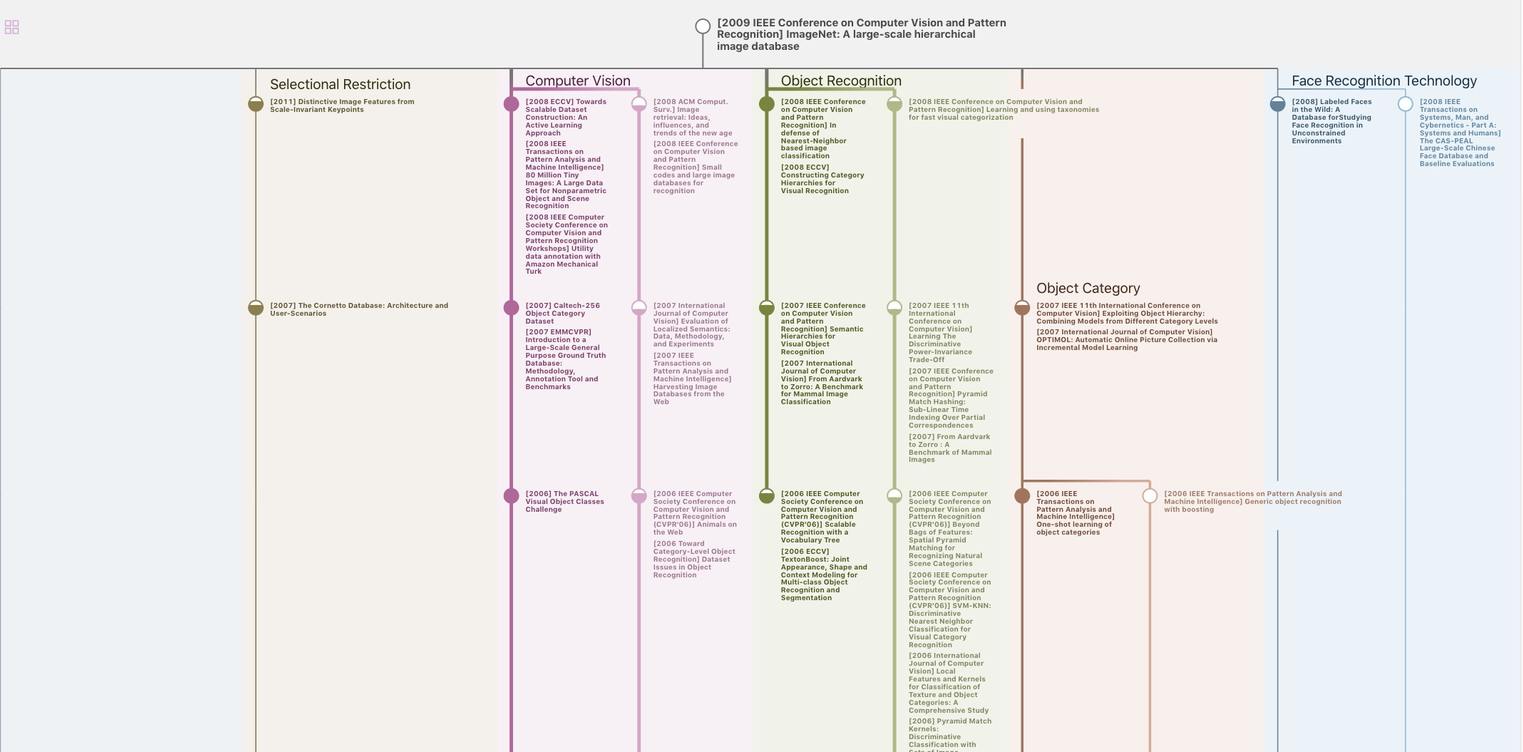
生成溯源树,研究论文发展脉络
Chat Paper
正在生成论文摘要