IMP: Iterative Matching and Pose Estimation With Adaptive Pooling
CVPR 2023(2023)
摘要
Previous methods solve feature matching and pose estimation using a two-stage process by first finding matches and then estimating the pose. As they ignore the geometric relationships between the two tasks, they focus on either improving the quality of matches or filtering potential outliers, leading to limited efficiency or accuracy. In contrast, we propose an iterative matching and pose estimation framework (IMP) leveraging the geometric connections between the two tasks: a few good matches are enough for a roughly accurate pose estimation; a roughly accurate pose can be used to guide the matching by providing geometric constraints. To this end, we implement a geometry-aware recurrent module with transformers which jointly outputs sparse matches and camera poses. Specifically, for each iteration, we first implicitly embed geometric information into the module via a pose-consistency loss, allowing it to predict geometry-aware matches progressively. Second, we introduce an efficient IMP (EIMP) to dynamically discard keypoints without potential matches, avoiding redundant updating and significantly reducing the quadratic time complexity of attention computation in transformers. Experiments on YFCC100m, Scannet, and Aachen Day-Night datasets demonstrate that the proposed method outperforms previous approaches in terms of accuracy and efficiency.
更多查看译文
关键词
pose estimation,iterative matching,adaptive
AI 理解论文
溯源树
样例
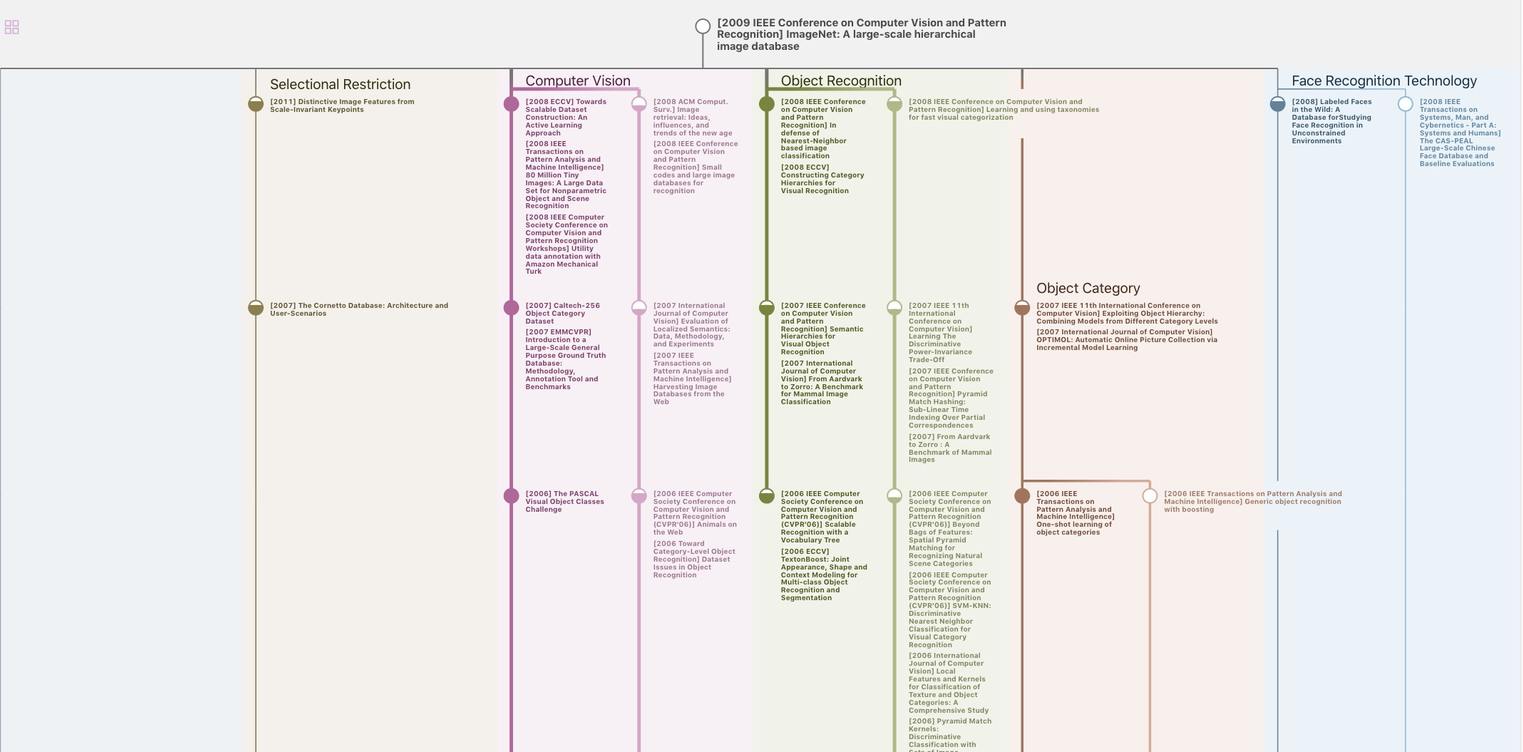
生成溯源树,研究论文发展脉络
Chat Paper
正在生成论文摘要