Bayesian testing of scientific expectations under exponential random graph models
SOCIAL NETWORKS(2024)
摘要
The exponential random graph (ERGM) model is a commonly used statistical framework for studying the determinants of tie formations from social network data. To test scientific theories under ERGMs, statistical inferential techniques are generally used based on traditional significance testing using p-values. This method-ology has certain limitations, however, such as its inconsistent behavior when the null hypothesis is true, its inability to quantify evidence in favor of a null hypothesis, and its inability to test multiple hypotheses with competing equality and/or order constraints on the parameters of interest in a direct manner. To tackle these shortcomings, this paper presents Bayes factors and posterior probabilities for testing scientific expectations under a Bayesian framework. The methodology is implemented in the R package BFpack. The applicability of the methodology is illustrated using empirical collaboration networks and policy networks.
更多查看译文
关键词
Bayesian hypothesis testing,Bayes factors,Exponential random graph models,g-priors
AI 理解论文
溯源树
样例
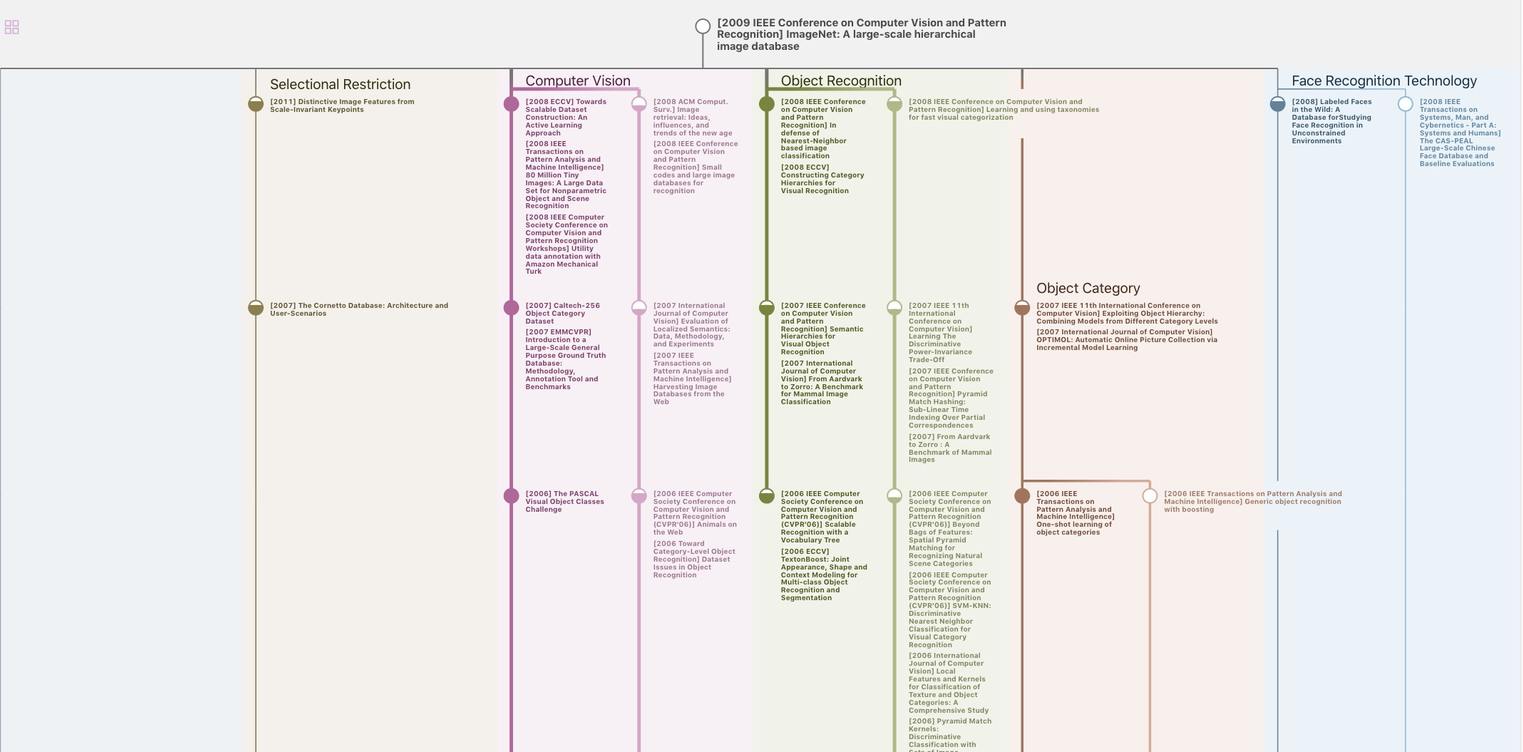
生成溯源树,研究论文发展脉络
Chat Paper
正在生成论文摘要